Generative artificial intelligence, also known as generative AI or gen AI for short, is a type of AI that can create new content and ideas, including conversations, stories, images, videos, and music. It can learn human language, programming languages, art, chemistry, biology, or any complex subject matter. It reuses what it knows to solve new problems.
For example, it can learn English vocabulary and create a poem from the words it processes.
Your organization can use generative AI for various purposes, like chatbots, media creation, product development, and design.","id":"collection-text-media#what-is-gen-ai-overview","mediaPosition":"left","mediaAltText":"Young business people working together on new project"},"metadata":{"tags":[]}}]},"metadata":{"auth":{},"testAttributes":{}},"context":{"page":{"locale":null,"site":null,"pageUrl":"https://aws.amazon.com/what-is/generative-ai/","targetName":null,"pageSlotId":null,"organizationId":null,"availableLocales":null},"environment":{"stage":"prod","region":"us-east-1"},"sdkVersion":"1.0.115"},"refMap":{"manifest.js":"47864e0370","rt-text-media-collection.js":"07ca5af217","rt-text-media-collection.rtl.css":"1747b99355","rt-text-media-collection.css":"6bf3dc6632","rt-text-media-collection.css.js":"9b48f61995","rt-text-media-collection.rtl.css.js":"f0296d99e1"},"settings":{"templateMappings":{"hyperlinkText":"hyperlinkText","hyperlinkUrl":"hyperlinkUrl","heading":"heading","mediaAltText":"mediaAltText","mediaPosition":"mediaPosition","mediaUrl":"mediaUrl","subheader":"subheader","bodyContent":"bodyContent","videoOverlayTitle":"videoOverlayTitle","videoThumbnailUrl":"videoThumbnailUrl","videoPlayButtonText":"videoPlayButtonText","dark":"dark"}}}
What is Gen AI?
Generative artificial intelligence, also known as generative AI or gen AI for short, is a type of AI that can create new content and ideas, including conversations, stories, images, videos, and music. It can learn human language, programming languages, art, chemistry, biology, or any complex subject matter. It reuses what it knows to solve new problems.
For example, it can learn English vocabulary and create a poem from the words it processes.
Your organization can use generative AI for various purposes, like chatbots, media creation, product development, and design.
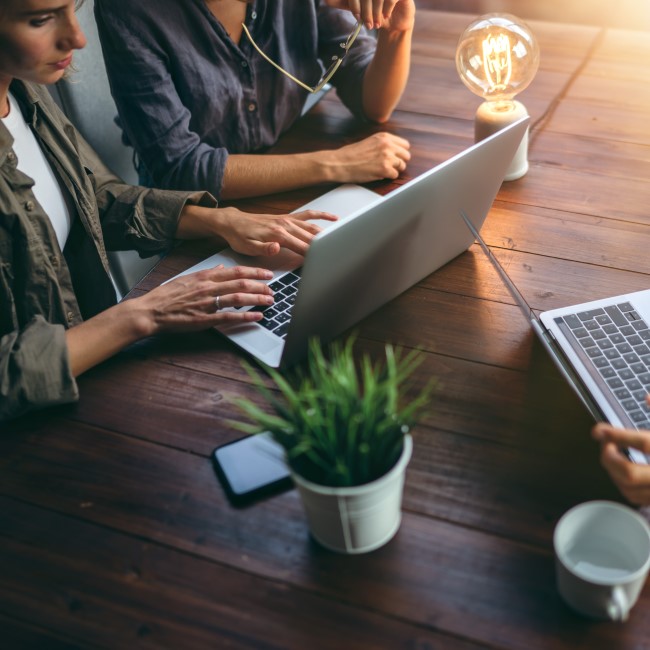
Financial services companies use generative AI tools to serve their customers better while reducing costs: \n
- \n
- Financial institutions use chatbots to generate product recommendations and respond to customer inquiries, which improves overall customer service. \n
- Lending institutions speed up loan approvals for financially underserved markets, especially in developing nations. \n
- Banks quickly detect fraud in claims, credit cards, and loans. \n
- Investment firms use the power of generative AI to provide safe, personalized financial advice to their clients at a low cost. \n \n
Read more about generative AI in Financial Services on AWS","itemMediaAltText":"Finance Pie Chart","itemOption":"right","itemMediaURL":"https://d1.awsstatic.com/device-a-industry-image-assets/pan-industry/pan-industry_1200/studio/Pan-Industry_Studio_277_1200.98c254a26cd597239cadffac460c1a6319f806c7.jpg","itemHeading":"Financial services","id":"awt-content-topics#tab-text-media-generative-ai-examples-1","itemTextLoc3":"Financial services","itemBoolean":"0","patternBoolean":"0"},"metadata":{"tags":[]}},{"fields":{"patternBoolean2":"0","patternBoolean3":"0","itemCustomSortOrder":"2","itemLongLoc":"
One of the most promising generative AI use cases is accelerating drug discovery and research. Generative AI can create novel protein sequences with specific properties for designing antibodies, enzymes, vaccines, and gene therapy. \n
Healthcare and life sciences companies use generative AI tools to design synthetic gene sequences for synthetic biology and metabolic engineering applications. For example, they can create new biosynthetic pathways or optimize gene expression for biomanufacturing purposes. \n
Generative AI tools also create synthetic patient and healthcare data. This data can be useful for training AI models, simulating clinical trials, or studying rare diseases without access to large real-world datasets. \n
Read more about Generative AI in Healthcare & Life Sciences on AWS","itemMediaAltText":"Hands around Globe","itemOption":"right","itemMediaURL":"https://d1.awsstatic.com/NPO%20Getting%20Started.7e43ec32fff02c2e2c0fd84dc31cdebc332ed160.jpg","itemHeading":"Healthcare and life sciences","id":"awt-content-topics#tab-text-media-generative-ai-examples-2","itemTextLoc3":"Healthcare and life sciences","itemBoolean":"0","patternBoolean":"0"},"metadata":{"tags":[]}},{"fields":{"patternBoolean2":"0","patternBoolean3":"0","itemCustomSortOrder":"3","itemLongLoc":"
Automotive companies use generative AI technology for many purposes, from engineering to in-vehicle experiences and customer service. For instance, they optimize the design of mechanical parts to reduce drag in vehicle designs or adapt the design of personal assistants. \n
Auto companies use generative AI tools to deliver better customer service by providing quick responses to the most common customer questions. Generative AI creates new materials, chips, and part designs to optimize manufacturing processes and reduce costs. \n
Another generative AI use case is synthesizing data to test applications. This is especially helpful for data not often included in testing datasets (such as defects or edge cases). \n
Read more about Generative AI for Automotive on AWS \n
Read more about Generative AI in Manufacturing on AWS","itemMediaAltText":"Automotive and manufacturing","itemOption":"right","itemMediaURL":"https://d2908q01vomqb2.cloudfront.net/a17554a0d2b15a664c0e73900184544f19e70227/2024/09/23/3D-Model-1-300x245.png","itemHeading":"Automotive and manufacturing","id":"awt-content-topics#tab-text-media-generative-ai-examples-3","itemTextLoc3":"Automotive and manufacturing","itemBoolean":"0","patternBoolean":"0"},"metadata":{"tags":[]}},{"fields":{"patternBoolean2":"0","patternBoolean3":"0","itemCustomSortOrder":"4","itemLongLoc":"
Generative AI use cases in telecommunication focus on reinventing the customer experience defined by the cumulative interactions of subscribers across all touchpoints of the customer journey. \n
For instance, telecommunication organizations apply generative AI to improve customer service with live human-like conversational agents. They reinvent customer relationships with personalized one-to-one sales assistants. They also optimize network performance by analyzing network data to recommend fixes. \n
Read more about Generative AI for Telecom on AWS","itemMediaAltText":"Telecommunication","itemOption":"right","itemMediaURL":"https://d1.awsstatic.com/industry/telecom/2023-site/transforming-telcos.077e03f55bd9bb9cc193165e533fd5bc40db0de0.jpg","itemHeading":"Telecommunication","id":"awt-content-topics#tab-text-media-generative-ai-examples-5","itemTextLoc3":"Telecommunication","itemBoolean":"0","patternBoolean":"0"},"metadata":{"tags":[]}},{"fields":{"patternBoolean2":"0","patternBoolean3":"0","itemCustomSortOrder":"5","itemLongLoc":"
From animations and scripts to full-length movies, generative AI models produce novel content at a fraction of the cost and time of traditional production. \n
Other generative AI use cases in the industry include: \n
- \n
- Artists can complement and enhance their albums with AI-generated music to create whole new experiences. \n
- Media organizations use generative AI to improve their audience experiences by offering personalized content and ads to grow revenues. \n
- Gaming companies use generative AI to create new games and allow players to build avatars. \n","itemMediaAltText":"Media and entertainment","itemOption":"right","itemMediaURL":"https://d1.awsstatic.com/DynamoDB%20Media%20Entertainment.9678cf61afa4af5af6f80a080e1221a5c69268ea.jpg","itemHeading":"Media and entertainment","id":"awt-content-topics#tab-text-media-generative-ai-examples-4","itemTextLoc3":"Media and entertainment","itemBoolean":"0","patternBoolean":"0"},"metadata":{"tags":[]}}]},"metadata":{"auth":{},"pagination":{"empty":false,"present":true},"testAttributes":{}},"context":{"page":{"locale":null,"site":null,"pageUrl":"https://aws.amazon.com/what-is/generative-ai/","targetName":null,"pageSlotId":null,"organizationId":null,"availableLocales":null},"environment":{"stage":"prod","region":"us-east-1"},"sdkVersion":"1.0.115"},"refMap":{"manifest.js":"d79353171a","rt-text-media-tabs.css":"14aae7f1bf","rt-text-media-tabs.rtl.css":"572f2b8858","rt-text-media-tabs.css.js":"f1a0eebc29","rt-text-media-tabs.js":"d74ed7a7b5","rt-text-media-tabs.rtl.css.js":"d51bcb746b"},"settings":{"templateMappings":{"tabLabel":"itemTextLoc3","patternHeading":"patternHeading","patternSubheading":"patternSubheading","patternTheme":"patternTextNoLoc","hyperlinkText":"itemCTALabel","hyperlinkUrl":"itemCTAURL","heading":"itemHeading","dark":"itemBoolean","mediaAltText":"itemMediaAltText","mediaPosition":"itemOption","mediaUrl":"itemMediaURL","body":"itemLongLoc","videoThumbnailUrl":"itemMediaURL2","videoOverlayTitle":"itemMediaAltText2","videoPlayButtonText":"itemTextLoc2"}}}
Generative AI examples
Generative AI has several use cases across industries
Financial services
Financial services companies use generative AI tools to serve their customers better while reducing costs:
- Financial institutions use chatbots to generate product recommendations and respond to customer inquiries, which improves overall customer service.
- Lending institutions speed up loan approvals for financially underserved markets, especially in developing nations.
- Banks quickly detect fraud in claims, credit cards, and loans.
- Investment firms use the power of generative AI to provide safe, personalized financial advice to their clients at a low cost.
Healthcare and life sciences
One of the most promising generative AI use cases is accelerating drug discovery and research. Generative AI can create novel protein sequences with specific properties for designing antibodies, enzymes, vaccines, and gene therapy.
Healthcare and life sciences companies use generative AI tools to design synthetic gene sequences for synthetic biology and metabolic engineering applications. For example, they can create new biosynthetic pathways or optimize gene expression for biomanufacturing purposes.
Generative AI tools also create synthetic patient and healthcare data. This data can be useful for training AI models, simulating clinical trials, or studying rare diseases without access to large real-world datasets.
Read more about Generative AI in Healthcare & Life Sciences on AWS
Automotive and manufacturing
Automotive companies use generative AI technology for many purposes, from engineering to in-vehicle experiences and customer service. For instance, they optimize the design of mechanical parts to reduce drag in vehicle designs or adapt the design of personal assistants.
Auto companies use generative AI tools to deliver better customer service by providing quick responses to the most common customer questions. Generative AI creates new materials, chips, and part designs to optimize manufacturing processes and reduce costs.
Another generative AI use case is synthesizing data to test applications. This is especially helpful for data not often included in testing datasets (such as defects or edge cases).
Telecommunication
Generative AI use cases in telecommunication focus on reinventing the customer experience defined by the cumulative interactions of subscribers across all touchpoints of the customer journey.
For instance, telecommunication organizations apply generative AI to improve customer service with live human-like conversational agents. They reinvent customer relationships with personalized one-to-one sales assistants. They also optimize network performance by analyzing network data to recommend fixes.
Media and entertainment
From animations and scripts to full-length movies, generative AI models produce novel content at a fraction of the cost and time of traditional production.
Other generative AI use cases in the industry include:
- Artists can complement and enhance their albums with AI-generated music to create whole new experiences.
- Media organizations use generative AI to improve their audience experiences by offering personalized content and ads to grow revenues.
- Gaming companies use generative AI to create new games and allow players to build avatars.
Generative AI benefits
According to Goldman Sachs, generative AI could drive a 7 percent (or almost $7 trillion) increase in global gross domestic product (GDP) and lift productivity growth by 1.5 percentage points over ten years. Next, we give some more benefits of generative AI.
How did generative AI technology evolve?
Primitive generative models have been used for decades in statistics to aid in numerical data analysis. Neural networks and deep learning were recent precursors for modern generative AI. Variational autoencoders, developed in 2013, were the first deep generative models that could generate realistic images and speech.
VAEs
VAEs (variational autoencoders) introduced the capability to create novel variations of multiple data types. This led to the rapid emergence of other generative AI models like generative adversarial networks and diffusion models. These innovations were focused on generating data that increasingly resembled real data despite being artificially created.
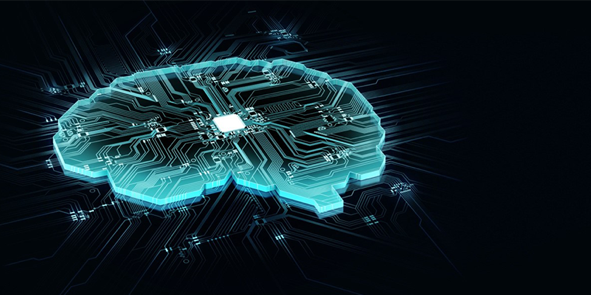
Transformers
In 2017, a further shift in AI research occurred with the introduction of transformers. Transformers seamlessly integrated the encoder-and-decoder architecture with an attention mechanism. They streamlined the training process of language models with exceptional efficiency and versatility. Notable models like GPT emerged as foundational models capable of pretraining on extensive corpora of raw text and fine-tuning for diverse tasks.
Transformers changed what was possible for natural language processing. They empowered generative capabilities for tasks ranging from translation and summarization to answering questions.
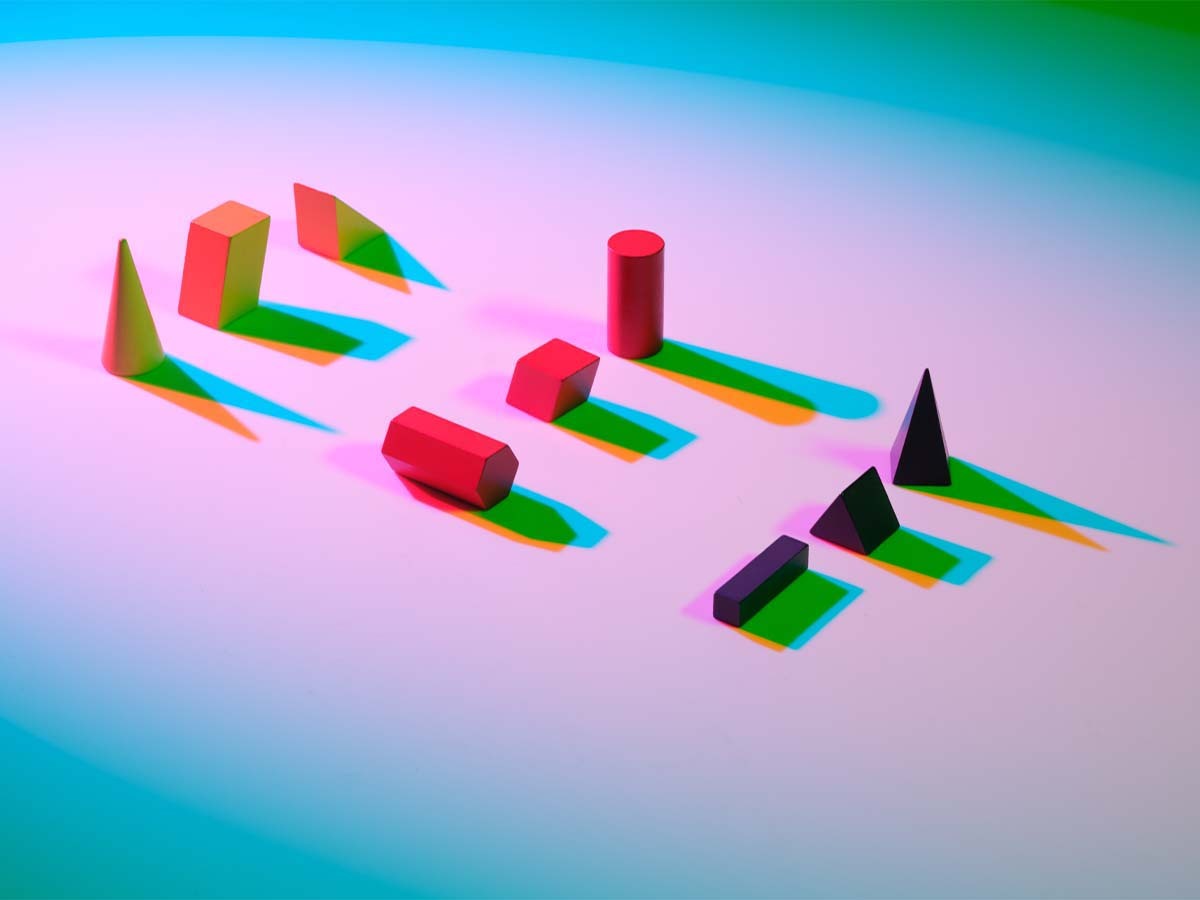
The future
Many generative AI models continue to make significant strides and have found cross-industry applications. Recent innovations focus on refining models to work with proprietary data. Researchers also want to create text, images, videos, and speech that are more and more human-like.
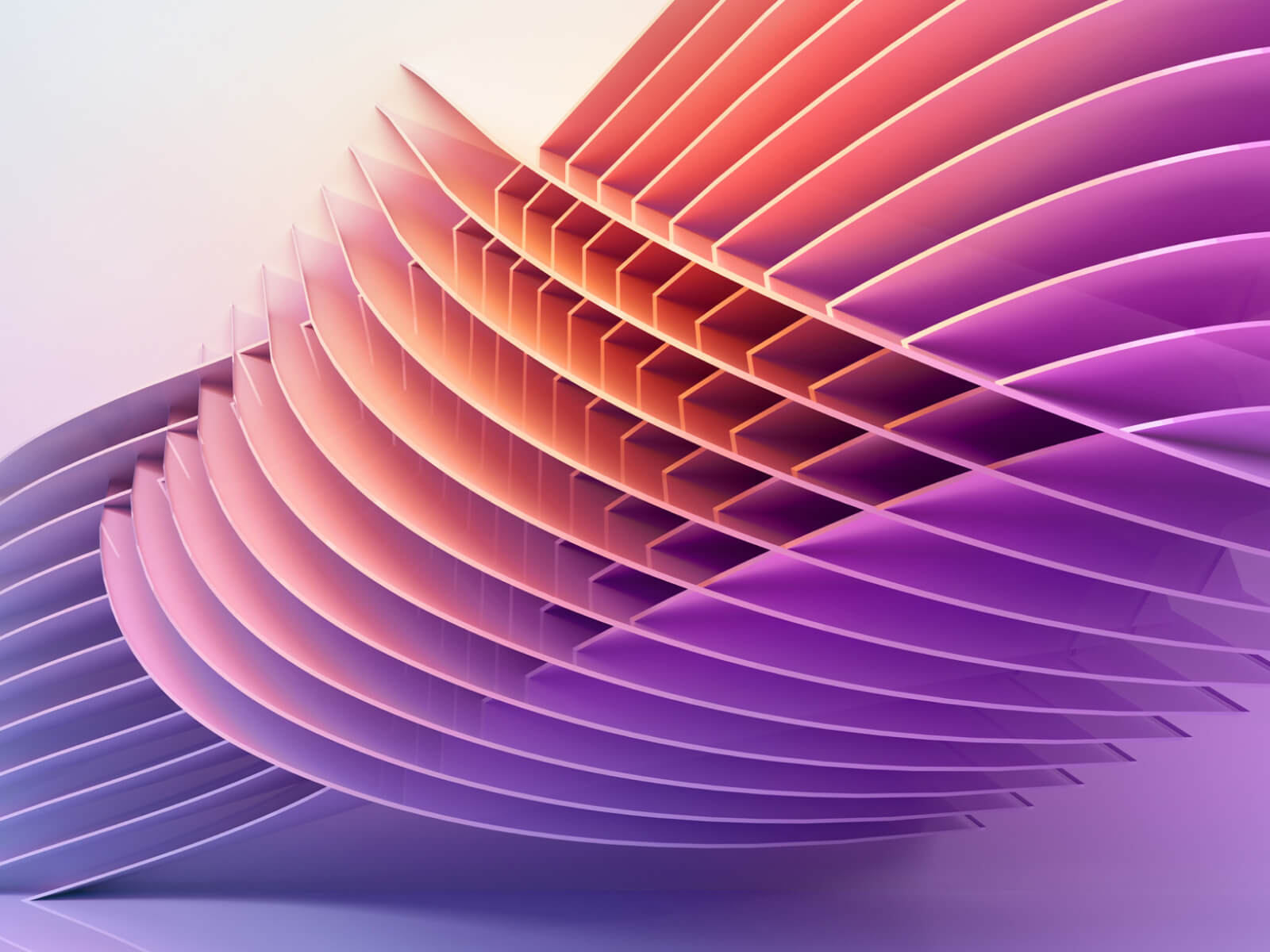
How does generative AI work?
Like all artificial intelligence, generative AI works by using machine learning models—very large models that are pre-trained on vast amounts of data.
Foundation models
Foundation models (FMs) are ML models trained on a broad spectrum of generalized and unlabeled data. They are capable of performing a wide variety of general tasks.
FMs are the result of the latest advancements in a technology that has been evolving for decades. In general, an FM uses learned patterns and relationships to predict the next item in a sequence.
For example, with image generation, the model analyzes the image and creates a sharper, more clearly defined version of the image. Similarly, with text, the model predicts the next word in a string of text based on the previous words and their context. It then selects the next word using probability distribution techniques.
Large language models
Large language models (LLMs) are one class of FMs. For example, OpenAI's generative pre-trained transformer (GPT) models are LLMs. LLMs are specifically focused on language-based tasks such as such as summarization, text generation, classification, open-ended conversation, and information extraction.
What makes LLMs special is their ability to perform multiple tasks. They can do this because they contain many parameters that make them capable of learning advanced concepts.
An LLM like GPT-3 can consider billions of parameters and has the ability to generate content from very little input. Through their pretraining exposure to internet-scale data in all its various forms and myriad patterns, LLMs learn to apply their knowledge in a wide range of contexts.
How do generative AI models work?
Traditional machine learning models were discriminative or focused on classifying data points. They attempted to determine the relationship between known and unknown factors. For example, they look at images—known data like pixel arrangement, line, color, and shape—and map them to words—the unknown factor. Mathematically, the models worked by identifying equations that could numerically map unknown and known factors as x and y variables. Generative models take this one step further. Instead of predicting a label given some features, they try to predict features given a certain label. Mathematically, generative modeling calculates the probability of x and y occurring together. It learns the distribution of different data features and their relationships. For example, generative models analyze animal images to record variables like different ear shapes, eye shapes, tail features, and skin patterns. They learn features and their relations to understand what different animals look like in general. They can then recreate new animal images that were not in the training set. Next, we give some broad categories of generative AI models.
Diffusion models
Diffusion models create new data by iteratively making controlled random changes to an initial data sample. They start with the original data and add subtle changes (noise), progressively making it less similar to the original. This noise is carefully controlled to ensure the generated data remains coherent and realistic.
After adding noise over several iterations, the diffusion model reverses the process. Reverse denoising gradually removes the noise to produce a new data sample that resembles the original.
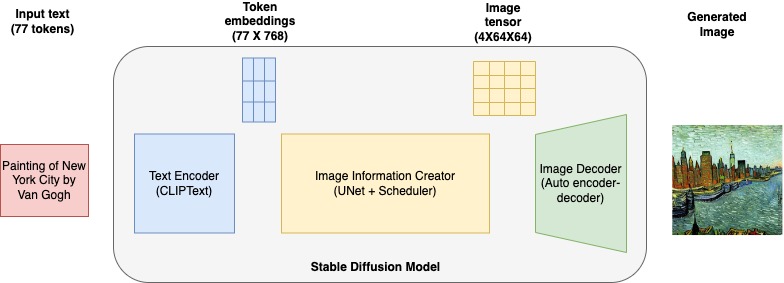
Generative adversarial networks
The generative adversarial network (GAN) is another generative AI model that builds upon the diffusion model’s concept.
GANs work by training two neural networks in a competitive manner. The first network, known as the generator, generates fake data samples by adding random noise. The second network called the discriminator, tries to distinguish between real data and the fake data produced by the generator.
During training, the generator continually improves its ability to create realistic data while the discriminator becomes better at telling real from fake. This adversarial process continues until the generator produces data that is so convincing that the discriminator can't differentiate it from real data.
GANs are widely used in generating realistic images, style transfer, and data augmentation tasks.
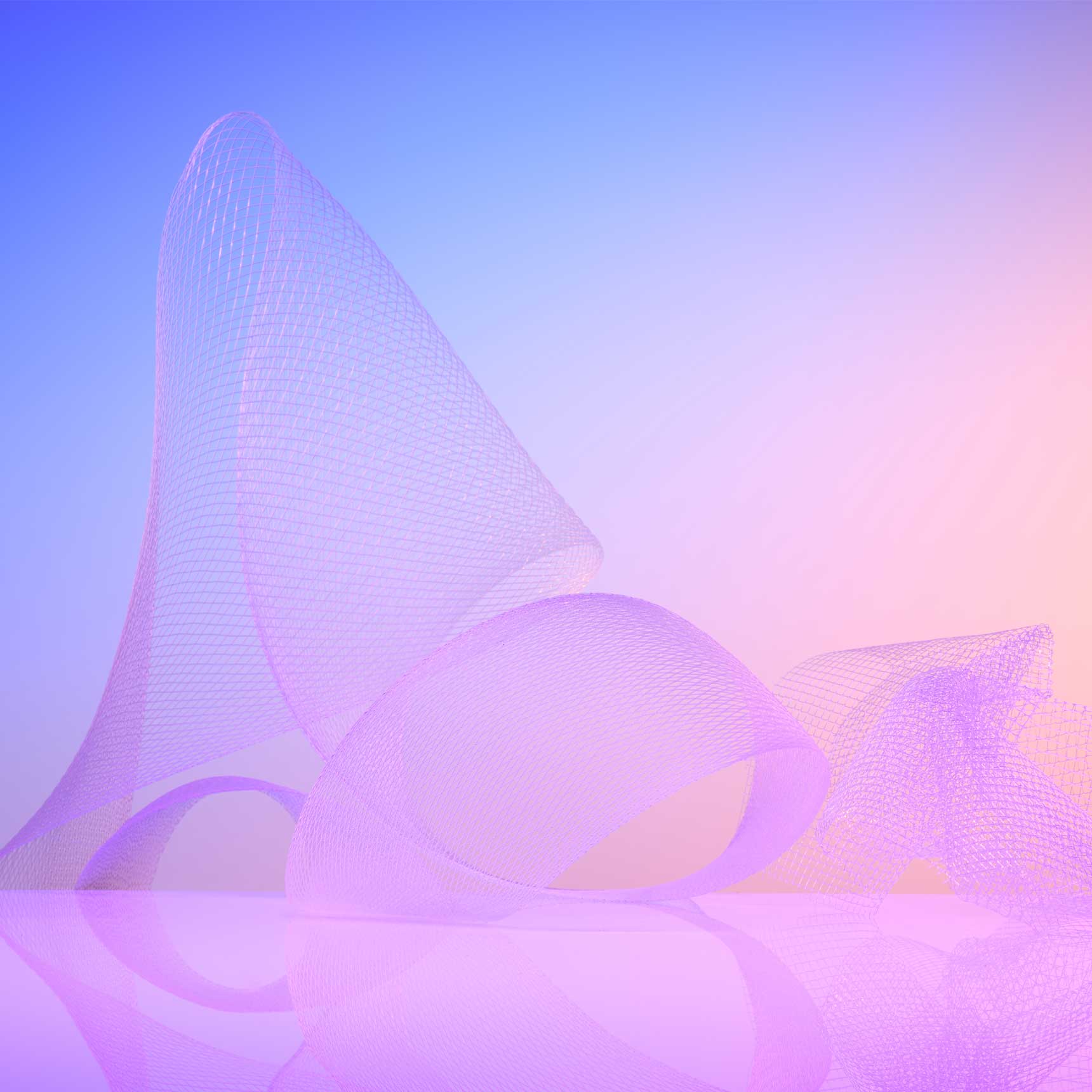
Variational autoencoders
Variational autoencoders (VAEs) learn a compact representation of data called latent space. The latent space is a mathematical representation of the data. You can think of it as a unique code representing the data based on all its attributes. For example, if studying faces, the latent space contains numbers representing eye shape, nose shape, cheekbones, and ears.
VAEs use two neural networks—the encoder and the decoder. The encoder neural network maps the input data to a mean and variance for each dimension of the latent space. It generates a random sample from a Gaussian (normal) distribution. This sample is a point in the latent space and represents a compressed, simplified version of the input data.
The decoder neural network takes this sampled point from the latent space and reconstructs it back into data that resembles the original input. Mathematical functions are used to measure how well the reconstructed data matches the original data.
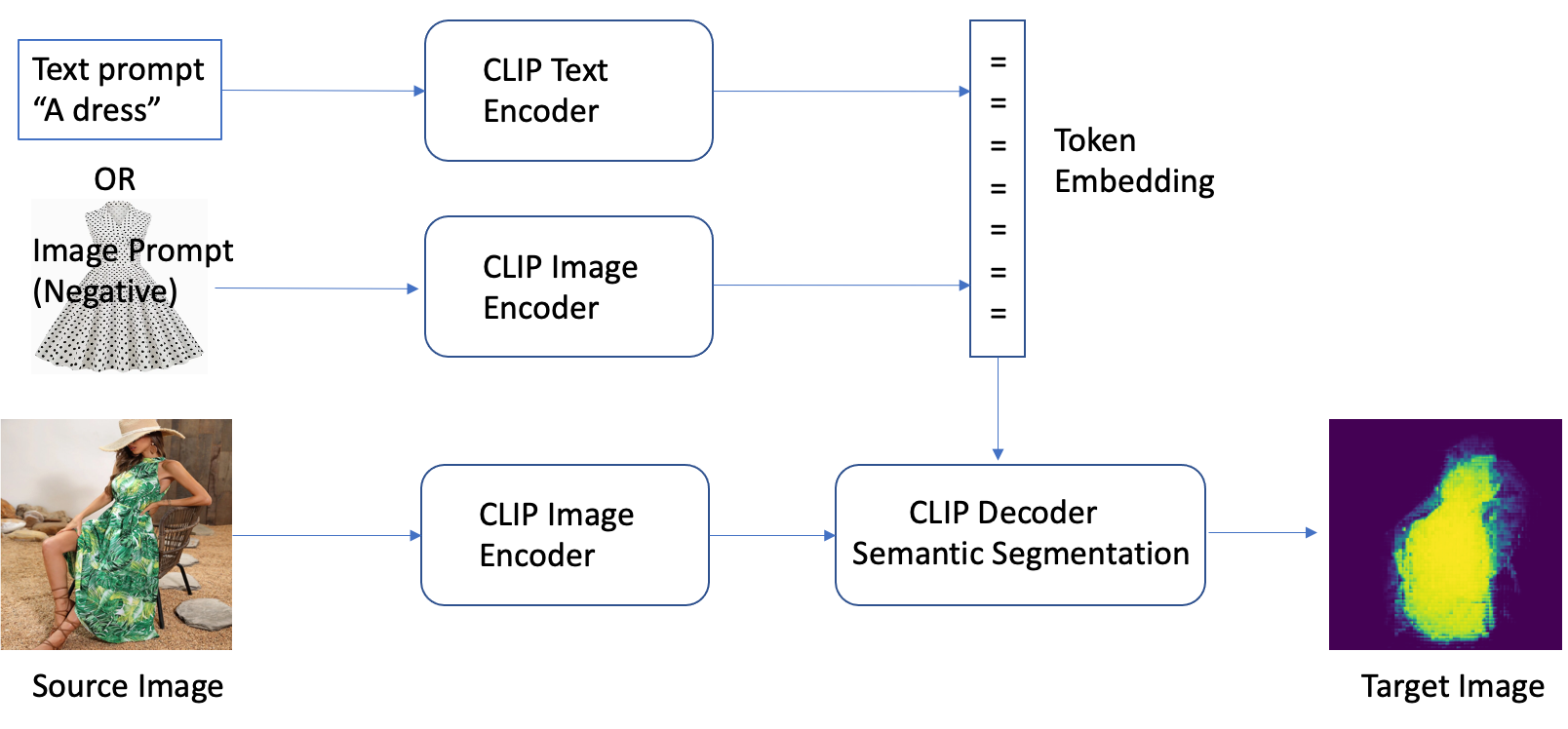
Transformer-based models
The transformer-based generative AI model builds upon the encoder and decoder concepts of VAEs. Transformer-based models add more layers to the encoder to improve performance on text-based tasks like comprehension, translation, and creative writing.
Transformer-based models use a self-attention mechanism. They weigh the importance of different parts of an input sequence when processing each element in the sequence.
Another key feature is that these AI models implement contextual embeddings. The encoding of a sequence element depends not only on the element itself but also on its context within the sequence.
How transformer-based models work
To understand how transformer-based models work, imagine a sentence as a sequence of words.
Self-attention helps the model focus on the relevant words as it processes each word. The transformer-based generative model employs multiple encoder layers called attention heads to capture different types of relationships between words. Each head learns to attend to different parts of the input sequence, allowing the model to simultaneously consider various aspects of the data.
Each layer also refines the contextual embeddings, making them more informative and capturing everything from grammar syntax to complex semantic meanings.
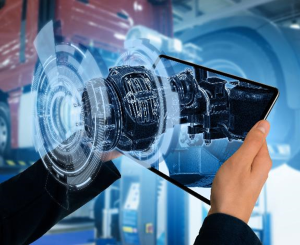
Generative AI training for beginners
Generative AI training begins with understanding foundational machine learning concepts. Learners also have to explore neural networks and AI architecture. Practical experience with Python libraries such as TensorFlow or PyTorch is essential for implementing and experimenting with different models. You also have to learn model evaluation, fine tuning and prompt engineering skills.
A degree in artificial intelligence or machine learning provides in-depth training. Consider online short courses and certifications for professional development. Generative AI training on AWS includes certifications by AWS experts on topics like:
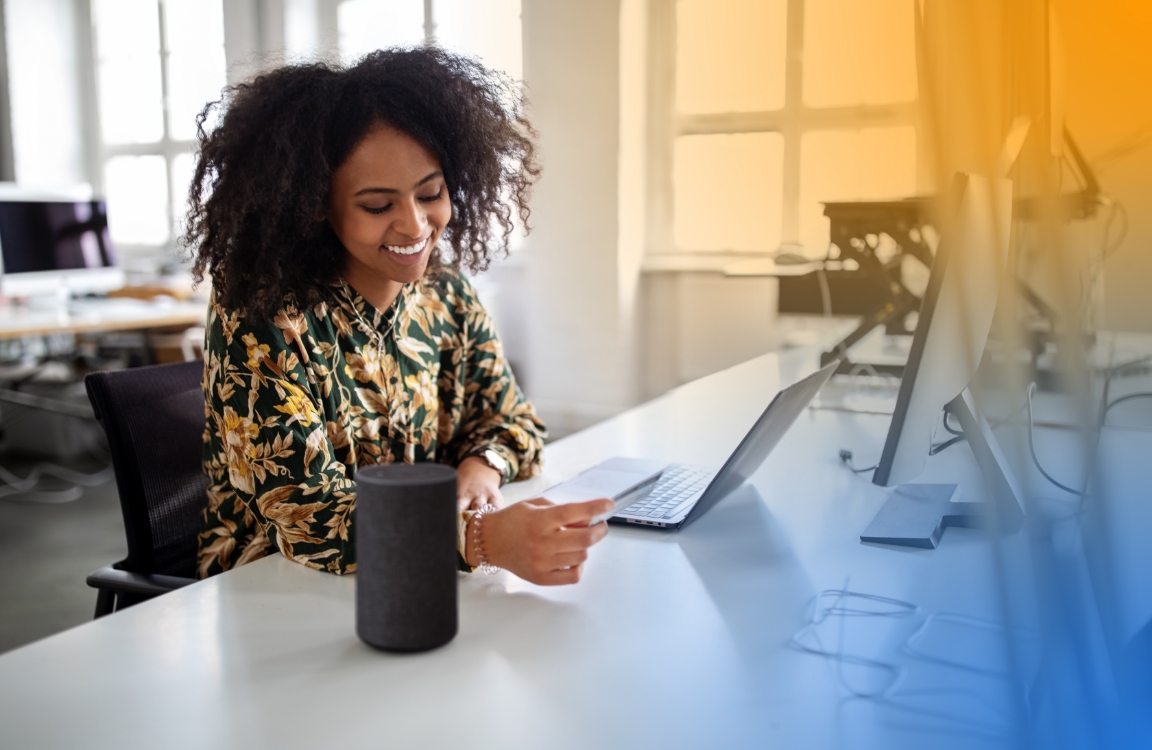
What are the limitations of generative AI?
Despite their advancements, generative AI systems can sometimes produce inaccurate or misleading information. They rely on patterns and data they were trained on and can reflect biases or inaccuracies inherent in that data. Other concerns related to training data include
Security
Data privacy and security concerns arise if proprietary data is used to customize generative AI models. Efforts must be made to ensure that the generative AI tools generate responses that limit unauthorized access to proprietary data. Security concerns also arise if there is a lack of accountability and transparency in how AI models make decisions.
Learn about the secure approach to generative AI using AWS
Creativity
While generative AI can produce creative content, it often lacks true originality. The creativity of AI is bounded by the data it has been trained on, leading to outputs that may feel repetitive or derivative. Human creativity, which involves a deeper understanding and emotional resonance, remains challenging for AI to replicate fully.
Cost
Training and running generative AI models require substantial computational resources. Cloud-based generative AI models are more accessible and affordable than trying to build new models from scratch.
Explainability
Due to their complex and opaque nature, generative AI models are often considered black boxes. Understanding how these models arrive at specific outputs is challenging. Improving interpretability and transparency is essential to increase trust and adoption.
What are the best practices in generative AI adoption?
If your organization wants to implement generative AI solutions, consider the following best practices to enhance your efforts.
How can AWS help Generative AI?
Amazon Web Services (AWS) makes it easy to build and scale generative AI applications for your data, use cases, and customers. With generative AI on AWS, you get enterprise-grade security and privacy, access to industry-leading FMs, generative AI-powered applications, and a data-first approach.