Article
Generative AI glossary: Key AI terms for 2025 and beyond
Our generative AI glossary covers the common AI words you need to know to understand artificial intelligence, including AI agents, automation, bias, and more.
Last updated December 3, 2024
Generative AI is transforming how businesses interact with customers and empower their employees. From streamlining responses to proactively identifying needs, generative AI improves experiences by enabling new levels of automation and personalization. As generative AI evolves, staying up to date on the key generative AI terms, concepts, and common AI words that define this field is crucial.
In our generative AI glossary, you’ll find 50+ essential AI terms to help you understand this evolving technology and its applications in customer experience (CX) and employee service.
Find a key term:
Artificial intelligence (AI)
Artificial intelligence (AI) is a concept referring to the ability of machines to mimic human cognitive functions and carry out tasks like understanding language, identifying patterns, solving complex problems, making decisions, and more.
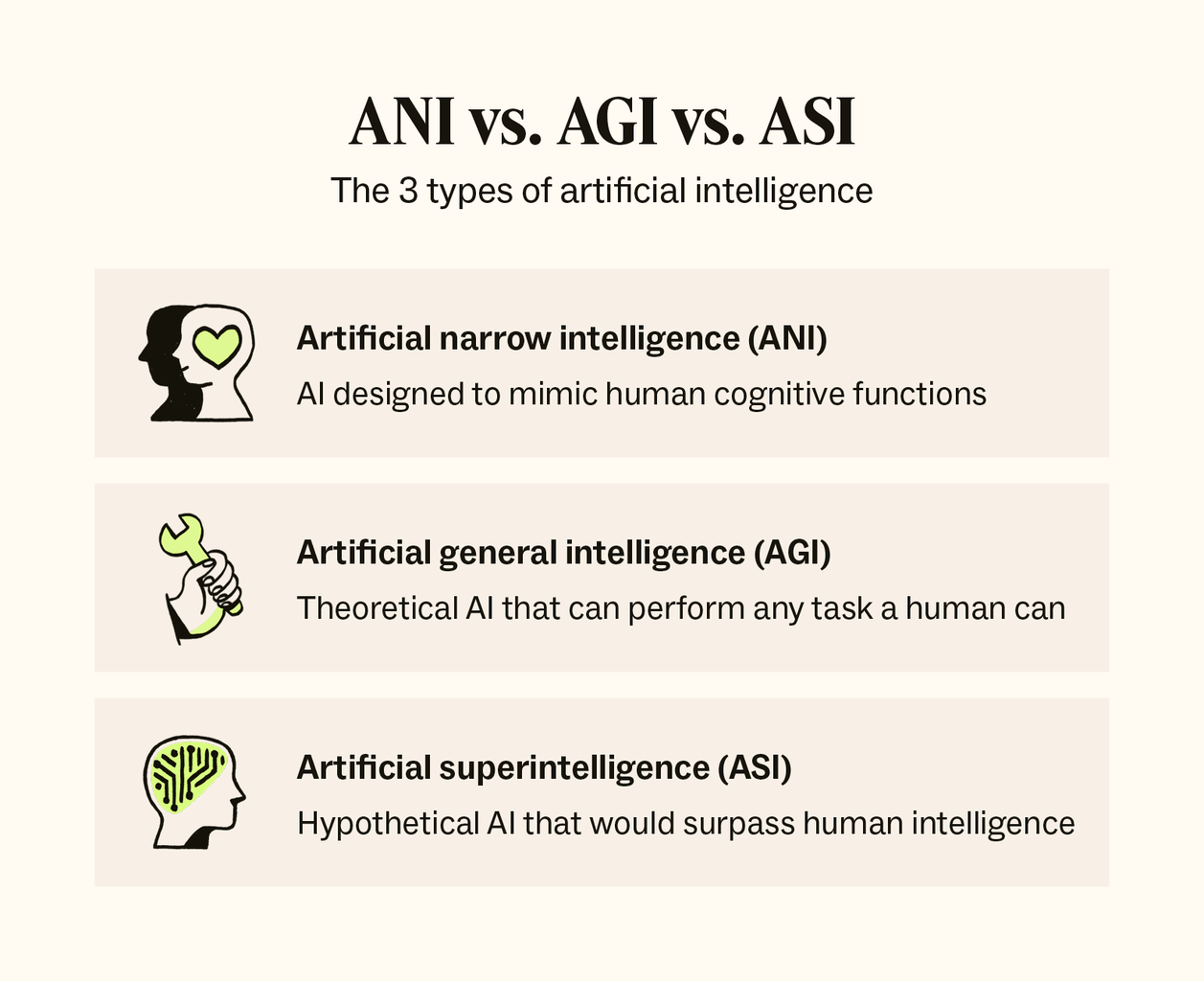
Artificial general intelligence (AGI)
Artificial general intelligence (AGI) is a theoretical form of AI that can perform any intellectual task a human can. An AGI system could understand, learn, and adapt independently, making it versatile beyond the abilities of narrow AI, which focuses on specific, pre-defined tasks.
Artificial narrow intelligence (ANI)
Artificial narrow intelligence (ANI), also known as weak AI, is an AI designed to perform a single task or a narrow set of functions with high efficiency and accuracy. Unlike general AI, ANI is limited in scope and cannot transfer knowledge or skills across domains.
Artificial superintelligence (ASI)
Artificial superintelligence (ASI) refers to a hypothetical intelligence level of AI that would surpass human intelligence in every field. While speculative, ASI goes beyond even AGI with extraordinary problem-solving and decision-making capabilities and raises important ethical considerations regarding AI’s potential impact on jobs and privacy.
AI agent
AI agents are the next generation of AI-powered bots equipped to understand and fully resolve even the most sophisticated customer issues. Zendesk AI Agents are the most autonomous bots in CX that can automatically detect what customers want, respond as your human agents would, and deliver immediate value from day one.
AI copilot
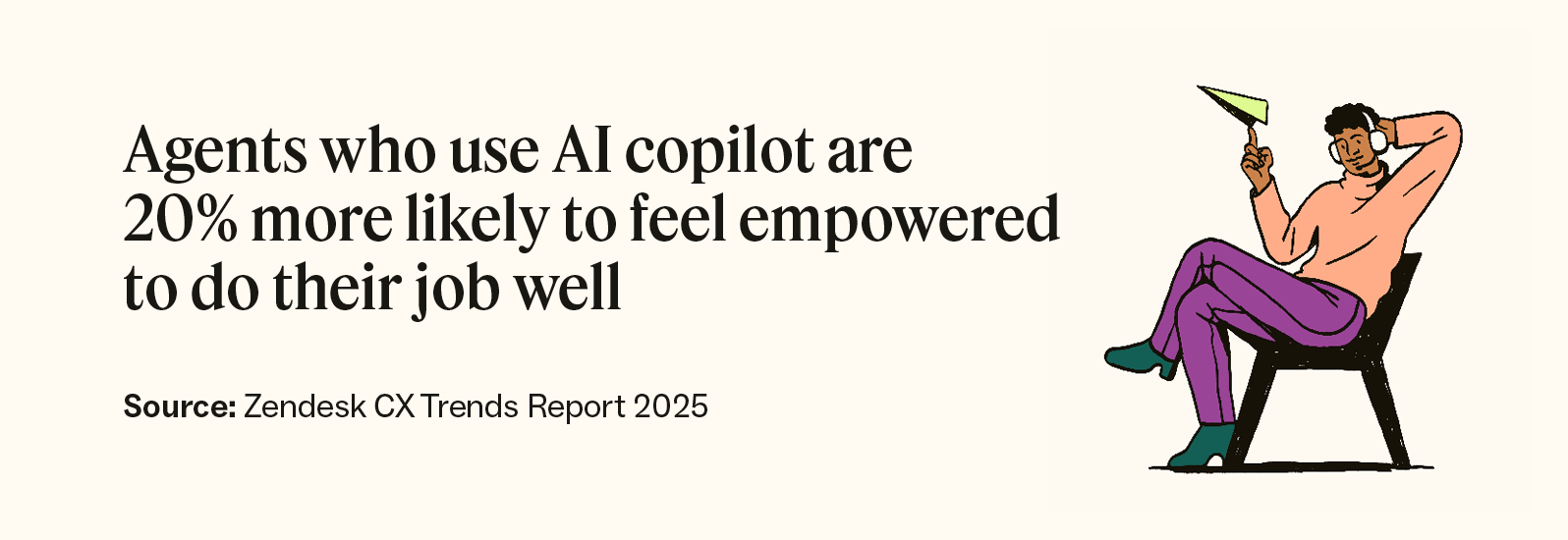
An AI copilot is an AI assistant that provides suggestions, resources, or actions for human users. As a feature of AI in customer service, AI copilots act similarly to actual airplane copilots by helping with tasks and even taking over certain functions. Zendesk AI copilot is the first advanced assistant for modern CX agents, increasing efficiency while helping agents respond to queries quickly and accurately.
AI ethics
AI ethics refers to the moral principles and values that guide the design, development, and deployment of responsible AI use. The common considerations of AI ethics in CX include AI transparency, fairness, privacy, and societal impact.
AI safety
AI safety focuses on measures and practices that prevent harm from AI systems, including errors or harmful biases. This involves mitigating potential risks and aligning AI objectives with human values.
AI toxicity
AI toxicity refers to the negative, harmful, false, inappropriate, or offensive outputs an AI system may unintentionally generate due to bias or misalignment.
Anthropomorphism
Anthropomorphism is attributing human traits, emotions, or intentions to non-human entities, including AI systems and other machines. Anthropomorphism can lead to unrealistic expectations and misunderstandings about an AI system’s true capabilities, but it can also make automated interactions feel more personal.
Augmentation
Augmentation refers to how technology can enhance and empower human capabilities rather than replace them entirely. In CX, AI can boost agent intelligence and augment human performance by managing repetitive tasks, allowing them to focus on complex, high-value interactions.
Automation
Automation is the use of AI and other technologies to perform tasks with minimal human intervention. In CX, there are many forms of automation, including:
Intelligent automation can improve response times, reduce workload, and boost efficiency in various business processes.
Bias
Bias in AI refers to the systematic errors or prejudices that favor certain groups or perspectives and can be introduced into machine learning models, often due to the system’s training data or algorithms. Addressing and reducing bias in conversational AI and generative AI is a key challenge in responsible AI development and ensuring fair, inclusive interactions for diverse audiences.
Chain of thought
When discussing AI, a chain of thought refers to the step-by-step reasoning process that an AI system uses to arrive at an output or decision. This method reaches conclusions by simulating human-like reasoning.
Chatbot
A chatbot is an AI-powered conversational interface that can engage in natural language interactions and human conversations with users. In customer service, chatbots, including natural language processing (NLP) chatbots, often provide automated support, answer queries, or complete simple tasks.
Conditional generation
Conditional generation is the ability of an AI system to generate outputs based on specific conditions or prompts. This allows for the creation of contextually relevant responses that are tailored to user needs or preferences.
Context window
In AI, the context window is the amount of preceding information that an AI model can retain or refer to during an interaction. A larger context window enables more coherent and detailed responses.
Conversational AI
Conversational AI encompasses intelligent technologies and systems that simulate natural, human-like interactions between humans and machines. This common AI term typically describes the technology used to run chatbots, virtual assistants, and other dialogue-based interfaces.
Deep learning
Deep learning (DL) is a subset of machine learning (ML) that uses artificial neural networks with many layers to learn and make predictions from data. In CX, deep learning and machine learning improve AI accuracy in understanding complex language and images and enhancing customer support capabilities.
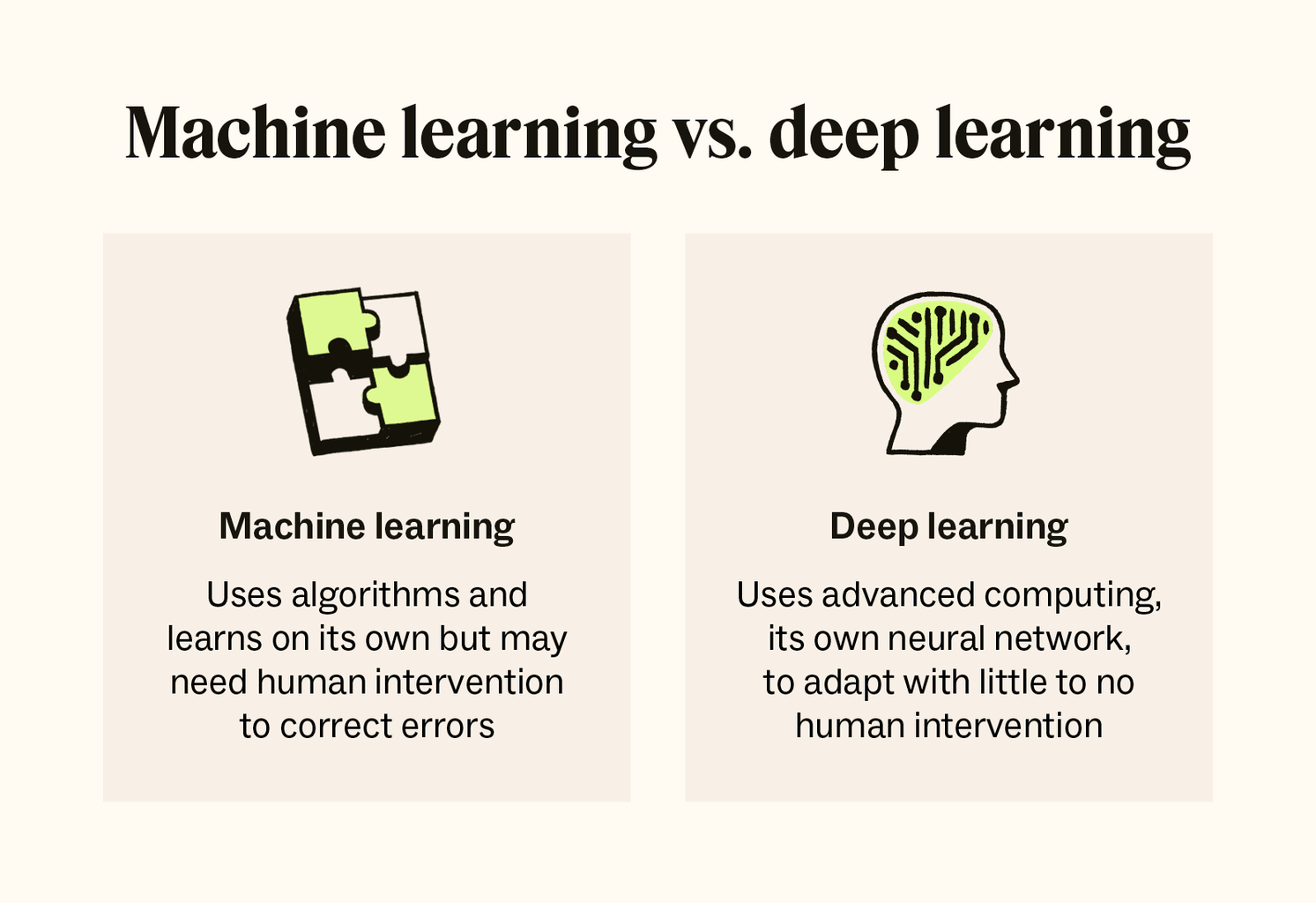
Emergent behavior
Emergent behavior refers to the unpredictable or unexpected patterns, actions, and capabilities that can arise from the interactions of complex AI systems with large datasets.
Explainable AI (XAI)
Explainable AI (XAI) is an AI model with transparent and interpretable systems. This type of AI model ensures human users understand its decision-making processes. It is one of the tools CX leaders use to prepare for the future, build trust, and prioritize accountability in using AI.
Feedback loops
Feedback loops in AI refer to the process of using an AI system’s outputs or results to inform and improve its future performance. These loops allow models to learn from user input and refine interactions over time.
Few-shot learning
Few-shot learning is the ability of an AI model to learn new tasks or concepts from a small number of examples rather than a large training dataset.
Fine-tuning
In the context of AI, fine-tuning refers to further adjusting a pre-trained model on a specific task or dataset to improve its performance in a certain domain.
Generator
In generative AI, a generator is the component of the system responsible for producing new, original content, including text, images, and audio. Generators are often paired with discriminators to form generative adversarial networks.
See also: Generative adversarial network (GAN)
Generative adversarial network (GAN)
A generative adversarial network (GAN) is a type of machine learning model that uses two neural networks—a generator and a discriminator—to generate realistic data.
- The generator creates new, synthetic data.
- The discriminator identifies whether the data is real or generated.
GANs can be used in areas like customer experience to generate synthetic data to improve AI models.
Generative AI
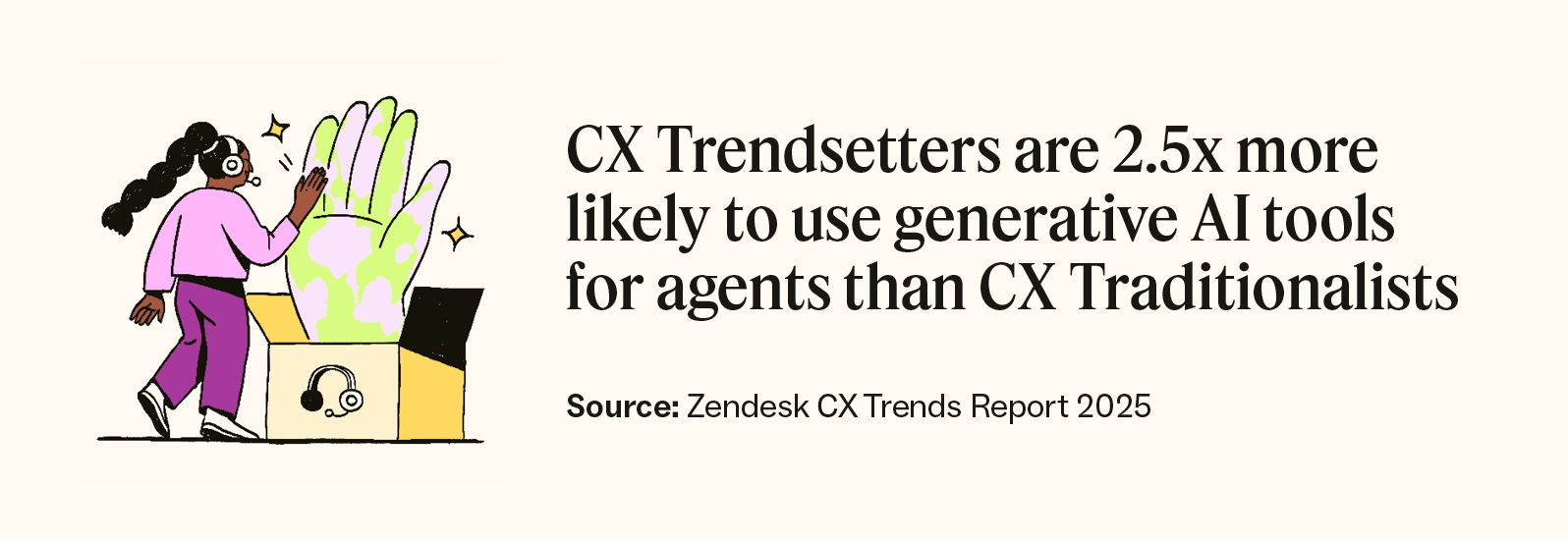
Generative AI is the branch of AI that uses large datasets to create new, original content, including text, images, audio, code, and more. This AI model focuses less on analysis, categorization, or decision-making and more on simulating human-like interactions.
Generative pre-trained transformer (GPT)
A generative pre-trained transformer (GPT) is a type of large language model (LLM) developed by OpenAI that has become a foundational technology for many NLP and generative AI applications.
Discover the future
Meet the future head-on as AI leads a customer service revolution.
Hallucination
Hallucination refers to the phenomenon where an AI system generates content that appears plausible but is factually incorrect, nonsensical, implausible, or unrelated. Address this generative AI problem during training to ensure the reliability of generative AI.
Inference
An inference in AI is the process of using a trained model to make predictions or decisions based on new input data. This is also a distinct learning phase where the model learns patterns from historical data.
Large language model (LLM)
Large language models (LLMs) are AI systems trained on vast amounts of text data, allowing them to understand and generate human-like language and responses. LLMs form the foundation for many advanced natural language processing applications.
Machine learning
Machine learning is a field of AI that teaches machines to learn and improve from data or experiences without being explicitly programmed. ML also uses statistical techniques to train models on data to make predictions or decisions.
Model
A model represents an AI structure trained to complete specific tasks, such as classification, prediction, or generation. For example, CX AI models are trained on customer service data to predict needs, improve responses, and personalize interactions.
See also: Large language model (LLM)
Natural language processing (NLP)
Natural language processing is an AI field focused on enabling machines to understand, interpret, and generate human-like language. It includes tasks like text classification, sentiment analysis, and language translation.
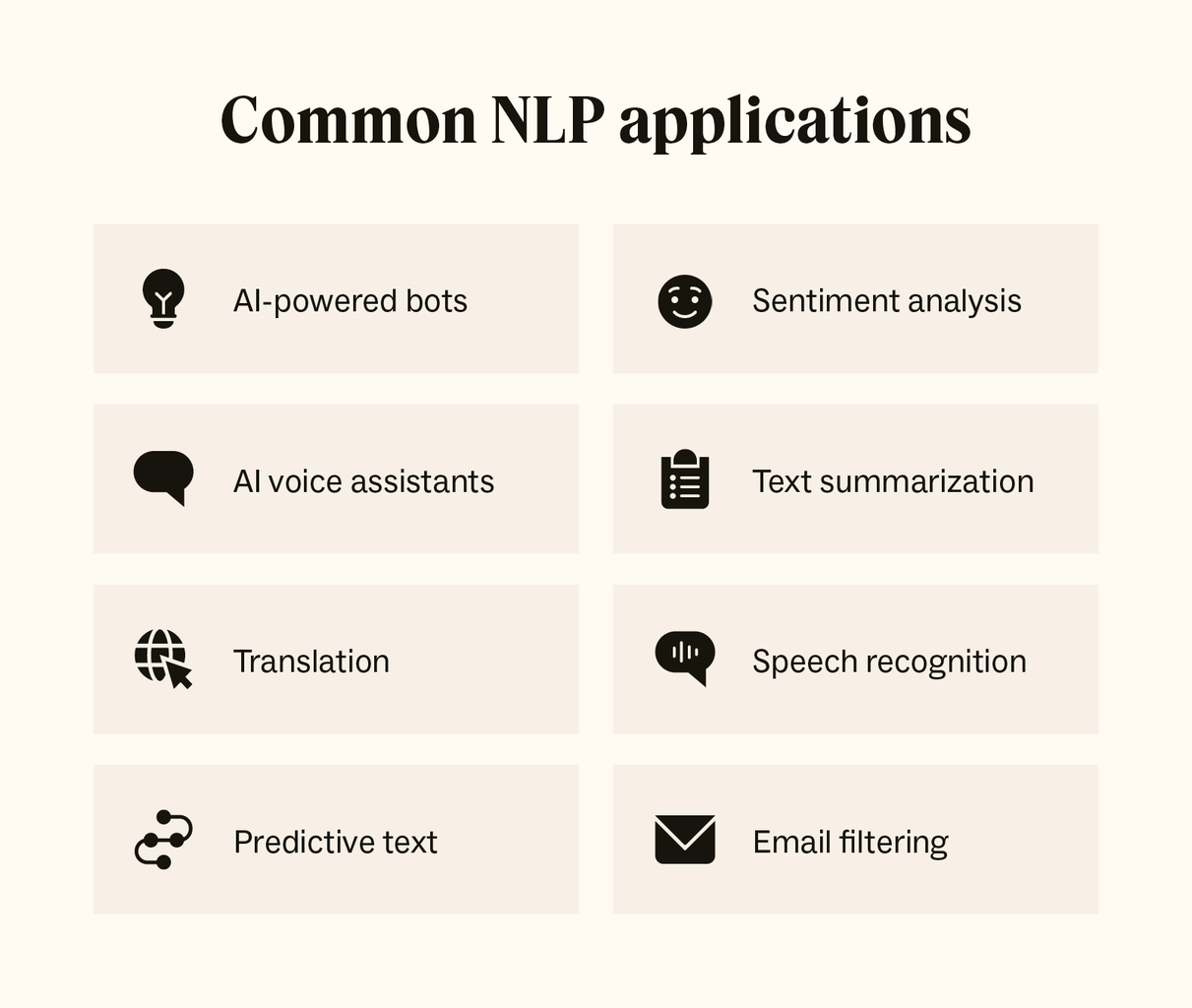
Neural network
A neural network is a machine-learning model that simulates human brain activity to process complex data. It is made of interconnected nodes (like neurons) that learn to perform specific tasks by processing data and recognizing patterns.
Parameters
In the context of AI, parameters refer to the internal variables that are adjusted during training to fine-tune performance and used by AI models to make predictions or generate new content.
Pre-trained model
A pre-trained model is an AI model trained on a large, general dataset and can be fine-tuned for specific tasks or applications. For example, Zendesk AI Agents are pre-trained on billions of real CX interactions, making them experts in customer service out of the box.
Prompt
A prompt is the initial textual or informational input provided to an AI system that guides an AI model’s generation of new content. Effective prompt engineering improves the output of AI models and helps users achieve desired results.
Prompt engineering
Prompt engineering refers to designing and optimizing prompts to gain a desired behavior or output from a generative AI system. Prompt engineering includes techniques like:
Prompt templates
Prompt chaining
Prompt tuning
Even though AI systems model human speech, they require detailed inputs to produce high-quality, usable outputs.
Reinforcement learning
Reinforcement learning is a type of training method in which an AI model learns by receiving rewards or penalties. A lack of reinforcement learning from human feedback—when users directly tell a generative AI system where it failed or succeeded—is a common pitfall of AI in customer service.
Retrieval augmented generation (RAG)
Retrieval augmented generation (RAG) is a technique that combines LLMs with information retrieval systems to produce more accurate and factual outputs. RAG models can draw on internal AI knowledge bases and external knowledge sources during generation.
Sentiment analysis
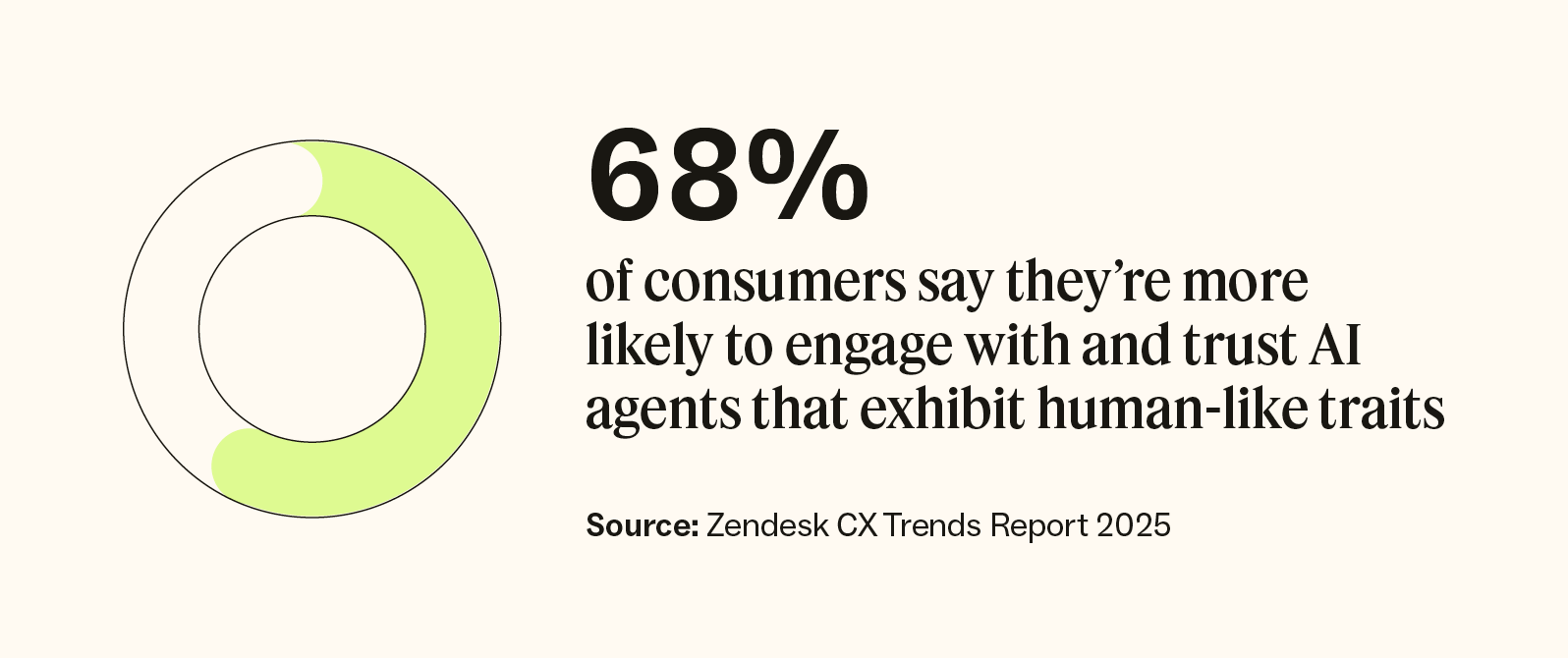
Sentiment analysis uses NLP to detect and determine emotional tones or opinions expressed in a piece of text. CX-focused AI models use this analysis process to determine customer sentiment, which can improve customer support and guide product or service improvements.
Supervised learning
Supervised learning is a machine-learning training method where the AI system is trained on labeled data, meaning the input data is paired with the desired output. This helps each model learn to map the inputs to the outputs.
Temperature
In generative AI, temperature is a parameter that controls the level of randomness or creativity in a model’s output. Higher temperatures can result in more diverse, potentially less coherent responses.
Token
In NLP, a token is the basic unit of text—like a word or punctuation mark—that an AI model processes and analyzes. Tokens enable large language models to handle detailed responses in customer service.
Training
Training refers to teaching an AI model how to perform a specific task using specific datasets, such as classification, prediction, or generation.
Training data
Training data is the dataset used to train a machine learning model. The quality and diversity of the training data can significantly impact the model’s performance.
Transformer
A transformer is a type of neural network architecture that processes sequences of data for tasks. This type of model architecture has become popular for natural language processing and is the foundation for many LLMs like GPT.
Unsupervised learning
Unsupervised learning is a machine-learning training method in which the AI system is trained on unlabeled data that is not paired with specific outputs, requiring it to discover patterns and data structures on its own.
Validation
Validation refers to evaluating a trained model’s performance and accuracy on a new dataset to assess its generalization capabilities.
Zero data retention
Zero data retention is a privacy-preserving technique used in AI systems to ensure that no personal or sensitive information is retained or stored from training data. This technique is also known as differential privacy.
Zero-shot learning
Zero-shot learning is the ability of an AI system to perform a task or understand a concept without prior training. This requires models to make generalizations from known data and can produce more flexible, adaptable AI applications.
Take your CX to the next level with Zendesk AI
By familiarizing yourself with our generative AI glossary, you’re preparing to leverage AI for more meaningful CX and service experiences. Educating yourself and your teams also allows you to transform customer service with generative AI by understanding how to communicate clearly and provide proactive, personalized, and efficient services.
With AI-powered solutions like Zendesk, you can explore AI’s practical applications to meet customer needs and improve service standards, setting a foundation for future growth.
Learn more about how generative AI capabilities can transform your customer service and support with a Zendesk free trial.