Maneesh Goyal—just hitting his third anniversary as the COO of the Mayo Clinic Platform (their new technology-meets-big data-meets-AI team)—says his entire career has been "training for this job, and for this point in healthcare."
And "this point in healthcare," says Goyal, is an intersection of two things that, if merged together effectively, can revolutionize patient care.
ADVERTISEMENT
First, the healthcare industry is capturing more—and more important—patient data than ever before.
Second, the emergence of AI means that this data can be understood, quickly, in ways that are already saving lives.
For Goyal, that career "training" includes a background in finance, investing, product development, and engineering. He was in leadership roles at Welltok (which provides data-driven health solutions) and Miramar Venture Partners (an early investor in information technology). In early work with the Mayo Clinic Platform, he helped develop the original business model. He also helped launch the Advanced Care at Home program, which "allows patients with conditions previously managed in a hospital to have the option to transition to a home setting and receive high-quality virtual and in-person care and recovery services."
Today, Goyal is responsible for "developing and executing strategic plans and overall operational excellence and for assessing and developing processes, systems, and infrastructure" for Mayo Clinic Platform.
He oversees the unit's investments in technology, data analytics, and AI.
ROCHESTER MAGAZINE: How will the patient experience change for Mayo Clinic patients in the next five or ten years or 10 years?
MANEESH GOYAL: At a really macro level, what we're doing in the platform is addressing the point that Mayo sees 1.2, 1.3, maybe going to 1.4 million patients a year across our three domestic sites in our health system. We see patients from 130 different countries. But there's close to 8 billion people on the planet that are getting care. How do we enable better quality care for the folks that can't find their way into one of our facilities? And I think it's our opportunity, our mandate, to enable better delivery of care, whether the patient comes to Mayo Clinic or doesn't come to Mayo Clinic. So Mayo Clinic Platform is really geared toward trying to level the playing field. It'll never be level. The experience at Mayo is always going to be the experience at Mayo. But the experience at, say, Seoul National University in South Korea might be better in a partnership with us. We want to embed Mayo Clinic in greater than 50% of global healthcare providers. So it's a fairly big mandate.
ADVERTISEMENT
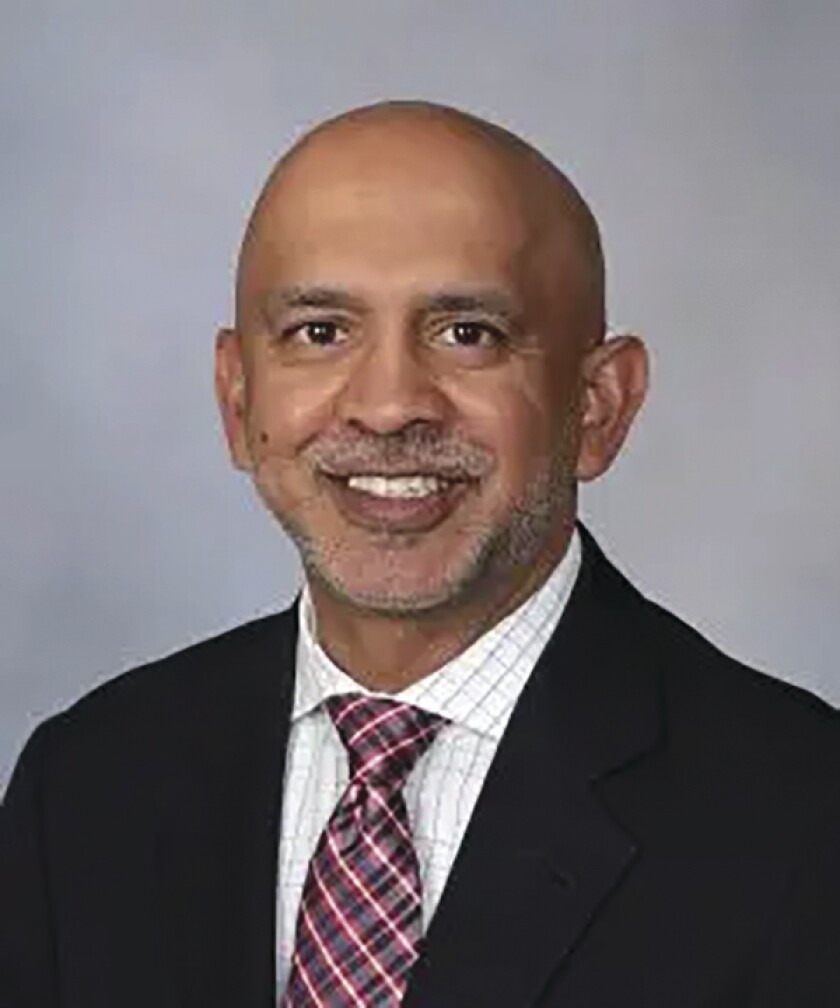
RM: Wow. That's a big footprint. How do patients get value out of that?
MG: First, the amount of data that's being produced on you and me as patients—or maybe better, you and me as consumers of healthcare goods and services—is increasing asymptotically every year, whether it's imaging data, whether it's metrics coming off of devices that you wear inside or outside of a hospital, or just notes that are being captured. We're at this interesting point in time where the information is far exceeding the ability of any human being to make sense of it all for an individual patient. So how our physicians and nurses interact with that data has to evolve.
RM: Can you give a specific example?
MG: Again, Mayo Clinic Platform is about innovation outside of Mayo and inside of Mayo. But I'm going to talk about innovation coming inside of Mayo in one example. So cardiology looked at, I think, 4 million ECGs [electrocardiograms, which record the electrical signal from the heart] of patients that came into Mayo, not for cardiovascular workup, but they were here for some other reason. So think of them as a massive control group. An algorithm was trained on that data set to find this left ventricular cardiovascular disease that otherwise doesn't go addressed until there's a cardiac event. It's usually somewhat catastrophic and sometimes fatal. So these are, honest to goodness, ticking time bombs inside of people. They trained an algorithm to identify risk of this particular condition. And remember, we have clinical history for all of those 4 million patients. So the algorithm identified a set of patients that we thought would probably be best to get a workup, about 400,000 patients. We reached out to them and said, "Would you come in for a clinical workup?" And a subset of them came in, and several hundred were put on a therapeutic because it was determined clinically that they did suffer from this condition. So those several hundred people experienced care that was otherwise the same, but then we just saved their lives and we saved the healthcare system a ton of money because the preventative measures are almost always cheaper than things when you have catastrophic conditions. That's just one example.
RM: That's amazing. I know you've worked a lot with creating better chat systems for patients to get better information more quickly. I love when the Mayo Clinic Portal sends me a reminder that I'm due for a follow-up from a previous visit, or I'm due for a colonoscopy or whatever. I also like when my auto mechanic sends me a text saying "Hey, your car's due for an oil change."
MG: And what's interesting about that analogy is, even that, is just based on standard of care for your car. Meaning at 5,000 miles you do your oil change. By this time you're looking at winterizing your car ... all of those things that are just normal. But if you knew the mechanical history of your car because you got into a fender bender that maybe offset the center of your car somewhat, or you haven't rotated your tires in some time, and you know the car has been driven off-road. Now you make that connection to healthcare. If you know that information, that colonoscopy isn't just because you're 50 years old and you should have a colonoscopy every other year. It's that you have a clinical history or you've got a genomic background that puts you at a higher risk. That is AI enablement, because now it's coming down to you.
RM: And really, this is what Mayo wants, right? They want to free up doctors and nurses to spend more time face-to-face with patients.
ADVERTISEMENT
MG: Well, that's exactly right. The idea is from moving behind my computer to actually looking and interacting with the consumer or patient. Mayo is experimenting with ambient listening. So there's a device in the room capturing all the notes so that the physician or nurse doesn't have to be frantically typing everything that you're saying. It's just a natural conversation. And then that conversation is turned into clinical notes for the physician, but also notes for the patient. So as the patient walks out, they've got a summary. So how many times have you gone to a physician and she's listed a set of things for you to do and you don't remember a single thing? If you've got actually a summary of the notes walking out, that's really helpful for you as a patient.
RM: And how far away is that kind of transcription process?
MG: I would say that's within the next one to three years.
RM: I could use that. I do think it's really important that Mayo is clearly on the forefront of AI because a lot of organizations will be looking to see how Mayo deals with AI. And I'm sure you're well aware of the weight of that.
MG: Absolutely. And we know what our lines are and we're well ahead of them. We are setting standards that are being established as law in other countries, including Europe. So it is a heavy load to carry, but also a pretty exciting thing to be in the forefront of AI.
AI, a timeline
When we were looking for a timeline on AI, we reached out to IBM, whose offices opened in Rochester 1958, just two years after the first use of the term “Artificial Intelligence.”
Today, the main focus of the Rochester IBM business is no longer mainframes, but AI.
ADVERTISEMENT
“For us, hardware systems are still an integral part of our strategy, and we’re still supporting the hardware business,” said former IBM Rochester leader Tory Johnson (now retired). “But we’re also a cloud and AI company now,” Johnson told us for a story in 2020. “That’s our fastest growing segment. This site represents what IBM is today.”
History of artificial intelligence: Key dates and names
The idea of ‘a machine that thinks’ dates back to ancient Greece. But since the advent of electronic computing (and relative to some important events and milestones in the evolution of artificial intelligence include the following:
1950: Alan Turing (pictured) publishes Computing Machinery and Intelligence. In the paper, Turing—famous for breaking the Nazi’s ENIGMA code during WWII—proposes to answer the question “Can machines think?” and introduces the Turing Test to determine if a computer can demonstrate the same intelligence (or the results of the same intelligence) as a human. The value of the Turing test has been debated ever since.
1956: John McCarthy coins the term ‘artificial intelligence’ at the first-ever AI conference at Dartmouth College. (McCarthy would go on to invent the Lisp language.) Later that year, Allen Newell, J.C. Shaw, and Herbert Simon create the Logic Theorist, the first-ever running AI software program.
1967: Frank Rosenblatt builds the Mark 1 Perceptron, the first computer based on a neural network that ‘learned’ through trial and error. Just a year later, Marvin Minsky and Seymour Papert publish a book titled Perceptrons, which becomes both the landmark work on neural networks and, at least for a while, an argument against future neural network research projects.
1980s: Neural networks which use a backpropagation algorithm to train itself become widely used in AI applications.
1997: IBM’s Deep Blue (pictured) beats then world chess champion Garry Kasparov, in a chess match (and rematch).
ADVERTISEMENT
2011: IBM Watson (pictured) beats champions Ken Jennings and Brad Rutter at Jeopardy!
2015: Baidu’s Minwa supercomputer uses a special kind of deep neural network called a convolutional neural network to identify and categorize images with a higher rate of accuracy than the average human.
2016: DeepMind’s AlphaGo program, powered by a deep neural network, beats Lee Sodol, the world champion Go player, in a five-game match. The victory is significant given the huge number of possible moves as the game progresses (over 14.5 trillion after just four moves!). Later, Google purchases DeepMind for a reported $400 million.
2023: A rise in large language models, or LLMs, such as ChatGPT, create an enormous change in performance of AI and its potential to drive enterprise value. With these new generative AI practices, deep-learning models can be pre-trained on vast amounts of raw, unlabeled data.