Embodied intelligence via learning and evolution
- PMID: 34615862
- PMCID: PMC8494941
- DOI: 10.1038/s41467-021-25874-z
Embodied intelligence via learning and evolution
Abstract
The intertwined processes of learning and evolution in complex environmental niches have resulted in a remarkable diversity of morphological forms. Moreover, many aspects of animal intelligence are deeply embodied in these evolved morphologies. However, the principles governing relations between environmental complexity, evolved morphology, and the learnability of intelligent control, remain elusive, because performing large-scale in silico experiments on evolution and learning is challenging. Here, we introduce Deep Evolutionary Reinforcement Learning (DERL): a computational framework which can evolve diverse agent morphologies to learn challenging locomotion and manipulation tasks in complex environments. Leveraging DERL we demonstrate several relations between environmental complexity, morphological intelligence and the learnability of control. First, environmental complexity fosters the evolution of morphological intelligence as quantified by the ability of a morphology to facilitate the learning of novel tasks. Second, we demonstrate a morphological Baldwin effect i.e., in our simulations evolution rapidly selects morphologies that learn faster, thereby enabling behaviors learned late in the lifetime of early ancestors to be expressed early in the descendants lifetime. Third, we suggest a mechanistic basis for the above relationships through the evolution of morphologies that are more physically stable and energy efficient, and can therefore facilitate learning and control.
© 2021. The Author(s).
Conflict of interest statement
The authors declare no competing interests.
Figures
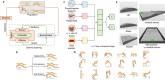
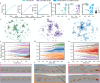
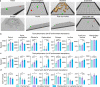
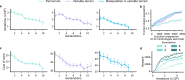
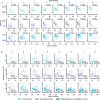
Similar articles
-
Exploring Embodied Intelligence in Soft Robotics: A Review.Biomimetics (Basel). 2024 Apr 19;9(4):248. doi: 10.3390/biomimetics9040248. Biomimetics (Basel). 2024. PMID: 38667259 Free PMC article. Review.
-
Environmental influence on the evolution of morphological complexity in machines.PLoS Comput Biol. 2014 Jan;10(1):e1003399. doi: 10.1371/journal.pcbi.1003399. Epub 2014 Jan 2. PLoS Comput Biol. 2014. PMID: 24391483 Free PMC article.
-
Risk preference as an outcome of evolutionarily adaptive learning mechanisms: An evolutionary simulation under diverse risky environments.PLoS One. 2024 Aug 1;19(8):e0307991. doi: 10.1371/journal.pone.0307991. eCollection 2024. PLoS One. 2024. PMID: 39088544 Free PMC article.
-
PaCAR: COVID-19 Pandemic Control Decision Making via Large-Scale Agent-Based Modeling and Deep Reinforcement Learning.Med Decis Making. 2022 Nov;42(8):1064-1077. doi: 10.1177/0272989X221107902. Epub 2022 Jul 1. Med Decis Making. 2022. PMID: 35775610
-
Deep reinforcement learning for modeling human locomotion control in neuromechanical simulation.J Neuroeng Rehabil. 2021 Aug 16;18(1):126. doi: 10.1186/s12984-021-00919-y. J Neuroeng Rehabil. 2021. PMID: 34399772 Free PMC article. Review.
Cited by
-
Artificial intelligence for geoscience: Progress, challenges, and perspectives.Innovation (Camb). 2024 Aug 22;5(5):100691. doi: 10.1016/j.xinn.2024.100691. eCollection 2024 Sep 9. Innovation (Camb). 2024. PMID: 39285902 Free PMC article. Review.
-
Exploring Embodied Intelligence in Soft Robotics: A Review.Biomimetics (Basel). 2024 Apr 19;9(4):248. doi: 10.3390/biomimetics9040248. Biomimetics (Basel). 2024. PMID: 38667259 Free PMC article. Review.
-
Integration of cognitive tasks into artificial general intelligence test for large models.iScience. 2024 Mar 22;27(4):109550. doi: 10.1016/j.isci.2024.109550. eCollection 2024 Apr 19. iScience. 2024. PMID: 38595796 Free PMC article. Review.
-
Body size as a metric for the affordable world.Elife. 2024 Mar 28;12:RP90583. doi: 10.7554/eLife.90583. Elife. 2024. PMID: 38547366 Free PMC article.
-
Enhancing robot evolution through Lamarckian principles.Sci Rep. 2023 Nov 30;13(1):21109. doi: 10.1038/s41598-023-48338-4. Sci Rep. 2023. PMID: 38036589 Free PMC article.
References
-
- Darwin, C. On the Origin of Species by Means of Natural Selection, Vol. 167 (John Murray, London, 1859).
-
- Pfeifer, R. & Scheier, C. Understanding Intelligence (MIT Press, 2001).
-
- Bongard J. Why morphology matters. Horiz. Evolut. Robot. 2014;6:125–152.
Publication types
MeSH terms
LinkOut - more resources
Full Text Sources