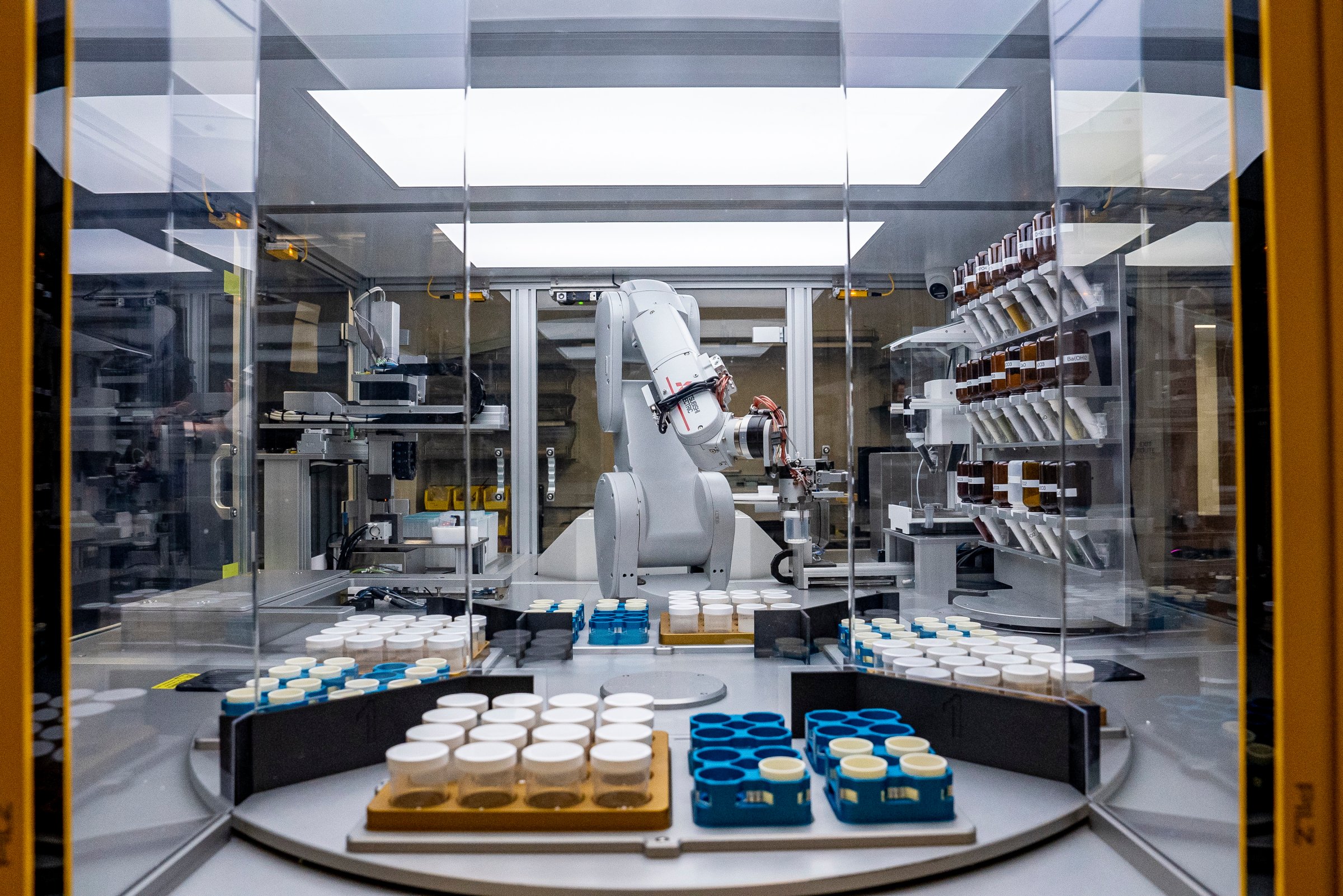
Researchers at Google DeepMind have used artificial intelligence to predict the structures of more than 2 million new materials, in a breakthrough that could have wide-reaching benefits in sectors such as renewable energy and computing.
DeepMind published 381,000 of the 2.2 million crystal structures that it predicts to be most stable.
The breakthrough increases the number of known stable materials by a factor of ten. Although the materials will still need to be synthesized and tested, steps which can take months or even years, the latest development is expected to accelerate the discovery of new materials, which will be required for applications such as energy storage, solar cells, and superconductor chips.
“While materials play a very critical role in almost any technology, we as humanity know only about a few tens of thousands of stable materials,” says Ekin Dogus Cubuk, a Staff Research Scientist at Google Brain, who worked on the DeepMind AI tool, known as Graph Networks for Materials Exploration (GNoME). That number gets even smaller when considering which materials are suitable for specific technologies, Cubuk told journalists at a briefing on Nov. 28. “Let's say you want to find a new solid electrolyte for better batteries. These electrolytes have to be ionically good conductors but electronically bad conductors, and they should not be toxic, they should not be radioactive. Once you apply all these filters, it turns out we only have a few options that we can go with, which end up not really revolutionizing our batteries.”
Only certain combinations of elements react to form stable solids—if the bonds between the constituent atoms aren’t strong enough, the solid will spontaneously decompose. Typically, new stable materials are discovered through trial and error by making incremental changes to known materials or by mixing elements together in line with principles derived from the field of solid state chemistry. The process is often expensive and can take months—human experimentation has yielded the structures of 20,000 stable materials in total. These structures are available in the Inorganic Crystal Structures Database (ICSD), the world’s largest database of identified materials.
Efforts have been made to computationally predict new materials in the past, most significantly by the Materials Project, a multinational research effort founded by Kristin Persson at the Lawrence Berkeley National Laboratory. These efforts have so far yielded an additional 28,000 stable materials.
GNoME was trained using data on material structures and their stability from the Materials Project. Next, the researchers had GNoME suggest new structures that its model determined would likely be stable. Established computational techniques were used to more accurately assess the stability of the materials generated by GNoME. This high-quality data was fed back into GNoME, increasing its stability prediction accuracy.
Google DeepMind took the 381,000 materials that are most likely to be stable from the 2.2 million total likely stable materials and added them to the ISCD—increasing the number of known materials predicted to be stable by a factor of ten. To test whether the materials GNoME predicts to be stable are indeed stable, Google DeepMind partnered with external researchers who successfully synthesized 736 of them.
Among the 381,000 materials were 528 potential lithium ion conductors that might be used in batteries, and 52,000 new layered compounds with a similar structure to graphene, opening up the possibility that some of these could be the basis for new superconducting materials. “We believe that some of these will be made in the lab, which will hopefully lead to very exciting applications,” said Cubuk.
Predicting whether crystal structures are likely to be stable gives materials scientists more targets to aim at, Cubuk noted. But that still leaves many time-consuming stages before the material can be useful: synthesizing the material, testing to see whether it exhibits useful properties such as conductivity, and devising larger scale synthesis methods.
Researchers at the Lawrence Berkeley National Laboratory are working to speed up the synthesis step. The A-Lab, an automated materials synthesis system, worked 24 hours a day, 7 days a week for 17 days to attempt to synthesize 58 materials predicted by the Materials Project, succeeding in 41 cases. Normally, it can take six months or even years to synthesize a material, Cubuk said.
“This is the future—to design materials autonomously using computers, but also then to make them autonomously using these robotic labs and learn from the process,” Persson said at the briefing.
In addition to accurately predicting whether a material will be stable, GNoME can predict whether it will behave as an efficient ionic conductor—an important property for batteries. The Google DeepMind researchers are optimistic that future AI tools will be able to predict other useful properties. “Machine learning models, when trained on a lot of data, really learn interesting aspects of quantum mechanics, and are able to generalize and make predictions about things that they were never trained on,” Cubuk said. “Which makes us very excited about our next challenges, such as predicting synthesizability.”
The GNoME breakthrough is just the latest from Google DeepMind, which previously produced the protein-folding predicting AlphaFold, genetic disease screening tool AlphaMissense, and weather-forecasting GraphCast.
“If you think about the protein structure prediction problem and if you think about material stability, both of them are root node problems, [that] we think unlock a number of different applications beyond those problems themselves,” Pushmeet Kohli, who leads Google DeepMind’s AI for Science team, said. “This specific problem has implications for so many other problems that society really cares about today.”
Correction, Nov. 29
The original version of this story misstated the organization that predicted the materials synthesized by the A-Lab. It was the Materials Project, not the Google DeepMind GNoME team.
More Must-Reads from TIME
- Why Trump’s Message Worked on Latino Men
- What Trump’s Win Could Mean for Housing
- The 100 Must-Read Books of 2024
- Sleep Doctors Share the 1 Tip That’s Changed Their Lives
- Column: Let’s Bring Back Romance
- What It’s Like to Have Long COVID As a Kid
- FX’s Say Nothing Is the Must-Watch Political Thriller of 2024
- Merle Bombardieri Is Helping People Make the Baby Decision
Write to Will Henshall at [email protected]