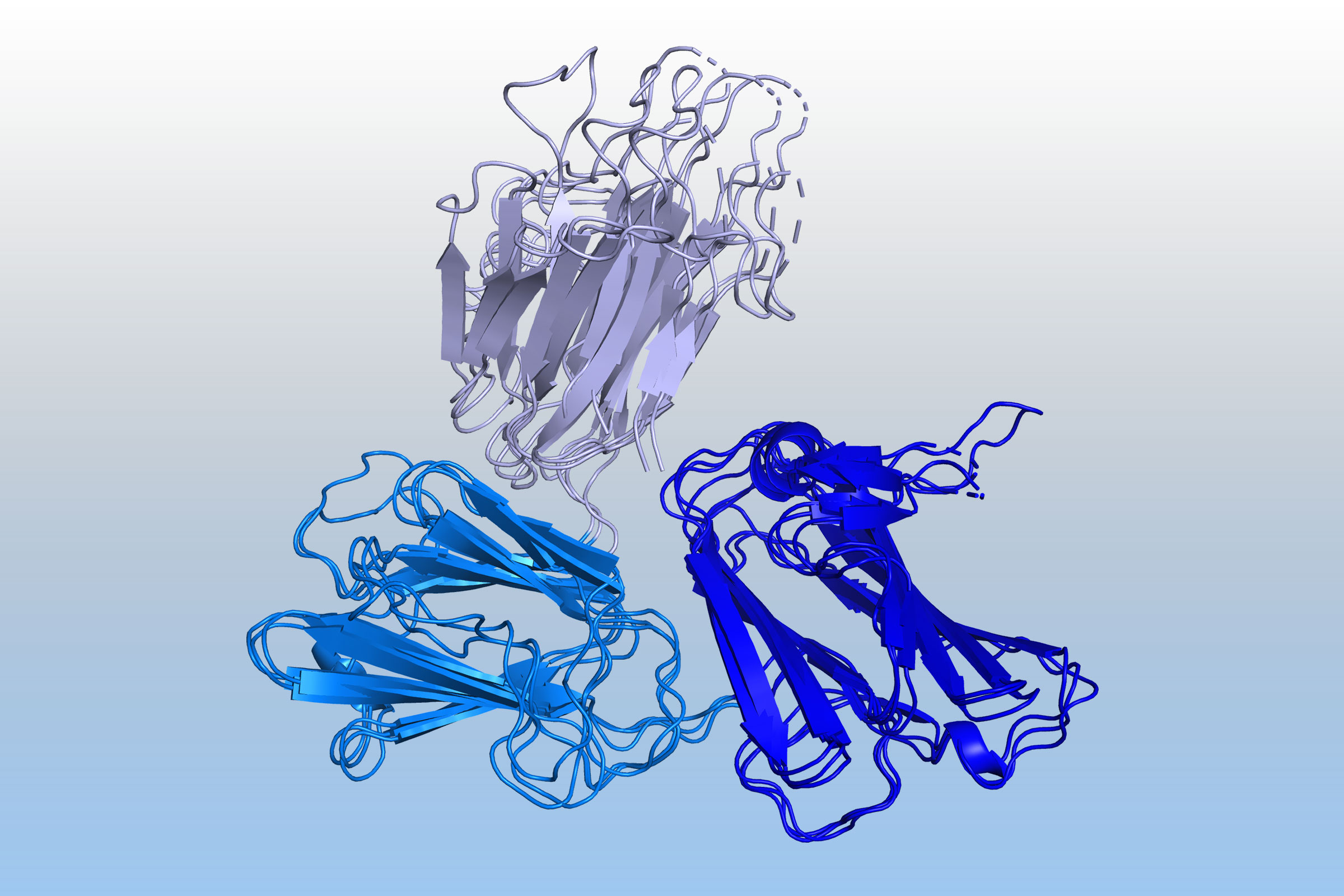
Matt Higgins and his team of researchers at the University of Oxford had a problem.
For years, they had been studying the parasite that spreads malaria, a disease that still kills hundreds of thousands of people every year. They had identified an important protein on the surface of the parasite as a focal point for a potential future vaccine. They knew its underlying chemical code. But the protein’s all-important 3D structure was eluding them. That shape was the key to developing the right vaccine to slide in and block the parasite from infecting human cells.
The team’s best way of taking a “photograph” of the protein was using X-rays—an imprecise tool that only returned the fuzziest of images. Without a clear 3D picture, their dream of developing truly effective malaria vaccines was just that: A dream. “We were never able, despite many years of work, to see in sufficient detail what this molecule looked like,” Higgins told reporters on Tuesday.
Then DeepMind came along. The artificial intelligence lab, which is a subsidiary of Google’s parent company Alphabet, had set its sights on solving the longstanding “grand challenge” within science of accurately predicting the 3D structures of proteins and enzymes. DeepMind built a program called AlphaFold that, by analyzing the chemical makeup of thousands of known proteins and their 3D shapes, could use that information to predict the shapes of unknown proteins with startling accuracy.
When DeepMind gave Higgins and his colleagues access to AlphaFold, the team was amazed by the results. “The use of AlphaFold was really transformational, giving us a really sharp view of this malaria surface protein,” Higgins told reporters, adding that the new clarity had allowed his team to begin testing new vaccines that targeted the protein. “AlphaFold has provided the ability to transform the speed and capability of our research.”
On Thursday, DeepMind announced that it would now be making its predictions of the 3D structures of 200 million proteins—almost all that are known to science—available to the entire scientific community. The disclosure, DeepMind CEO Demis Hassabis told reporters, would turbocharge the world of biology, facilitating faster work in fields as diverse as sustainability, food security and neglected diseases. “Now you can look up a 3D structure of a protein almost as easily as doing a keyword Google search,” Hassabis said. “It’s sort of like unlocking scientific exploration at digital speed.”
Read More: Demis Hassabis Is on the 2017 TIME 100
The AlphaFold project is good publicity for DeepMind, whose stated end goal is to build “artificial general intelligence,” or a theoretical computer that could carry out most imaginable tasks more competently and quickly than any human. Hassabis has described solving scientific challenges as necessary steps toward that end goal which, if successful, could transform scientific progress and human prosperity.
The DeepMind CEO has described AlphaFold as a “gift to humanity.” A DeepMind spokesperson told TIME that the company was making AlphaFold’s code and data freely available for any use, commercial or academic, under irrevocable open source licenses in order to benefit humanity and the scientific community. But some researchers and AI experts have raised concerns that even if machine learning research does accelerate the pace of scientific progress, it could also concentrate wealth and power in the hands of a tiny number of companies, threatening equity and political participation in wider society.
The allure of “artificial general intelligence” perhaps explains why DeepMind’s owner Alphabet (then known as Google), which paid more than $500 million for the lab in 2014, has historically allowed it to work on areas it sees as beneficial to humanity as a whole, even at great immediate cost to the company. DeepMind ran at a loss for years, with Alphabet writing off $1.1 billion of debt incurred from those losses in 2019, but it turned a modest profit of $60 million for the first time in 2020. That profit came entirely from selling its AI to other arms of the Alphabet empire, including tech that improves the efficiency of Google’s voice assistant, its Maps service, and the battery life on its Android phones.
AI’s complicated role in scientific discovery
The combination of masses of data and computing power, combined with powerful methods for spotting patterns known as neural networks, are fast transforming the scientific landscape. These technologies, often described as artificial intelligence, are helping scientists in fields as diverse as understanding the evolution of stars and boosting drug discovery.
But this transformation isn’t without its risks. In a recent study, researchers for a drug discovery company said that with only small tweaks, their drug discovery algorithm could generate toxic molecules like the VX nerve agent—and others, unknown to science, that could be even more deadly. “We have spent decades using computers and AI to improve human health—not to degrade it,” the researchers wrote. “We were naive in thinking about the potential misuse of our trade.”
For its part, DeepMind says it has carefully considered the risks of releasing the AlphaFold database to the public, saying it had made the decision after consulting with more than 30 experts in bioethics and security. “The assessment came back saying that [with] this release, the benefits far outweigh any risks,” Hassabis, DeepMind’s CEO, told TIME at a briefing with reporters on Tuesday.
Hassabis added that DeepMind had made some adjustments in response to the risk assessment, to be “careful” with the structure of viral proteins. A DeepMind spokesperson later clarified that viral proteins had been excluded from AlphaFold for technical reasons, and that the consensus among experts was that AlphaFold would not meaningfully lower the barrier to entry for causing harm with proteins.
The risks of making it possible for anybody to determine the 3D structure of a protein are far lower than the risk of allowing anybody access to a drug discovery algorithm, according to Ewan Birney, the director of the European Bioinformatics Institute, which partnered with DeepMind on the research. Even if AlphaFold were to facilitate a bad actor to design a dangerous compound, the same technology in the hands of the scientific community at large could be a force-multiplier for efforts to design antidotes or vaccines. “I think, like all risks, you have to think about the balance here and the positive side,” Birney told reporters Tuesday. “The accumulation of human knowledge is just a massive benefit. And the entities which could be risky are likely to be a very small handful. So I think we are comfortable.”
But DeepMind acknowledges the balance of risks may play out differently in the future. Artificial intelligence research has long been characterized by a culture of openness, with researchers at competing labs often sharing their source code and results publicly. But Hassabis indicated to reporters on Tuesday that as machine learning makes greater headway into other potentially more-risky areas of science, that open culture may need to narrow. “Future [systems], if they do carry risks, the whole community would need to consider different ways of giving access to that system—not necessarily open sourcing everything—because that could enable bad actors,” Hassabis said.
“Open-sourcing isn’t some sort of panacea,” Hassabis added. “It’s great when you can do it. But there are often cases where the risks may be too great.”
More Must-Reads from TIME
- Why Trump’s Message Worked on Latino Men
- What Trump’s Win Could Mean for Housing
- The 100 Must-Read Books of 2024
- Sleep Doctors Share the 1 Tip That’s Changed Their Lives
- Column: Let’s Bring Back Romance
- What It’s Like to Have Long COVID As a Kid
- FX’s Say Nothing Is the Must-Watch Political Thriller of 2024
- Merle Bombardieri Is Helping People Make the Baby Decision
Write to Billy Perrigo at [email protected]