Synergistic Effects of Fructose and Food Preservatives on Metabolic Dysfunction-Associated Steatotic Liver Disease (MASLD): From Gut Microbiome Alterations to Hepatic Gene Expression
- PMID: 39519554
- PMCID: PMC11547954
- DOI: 10.3390/nu16213722
Synergistic Effects of Fructose and Food Preservatives on Metabolic Dysfunction-Associated Steatotic Liver Disease (MASLD): From Gut Microbiome Alterations to Hepatic Gene Expression
Abstract
Background/Objectives: Metabolic dysfunction-associated steatotic liver disease (MASLD) is a growing global health problem closely linked to dietary habits, particularly high fructose consumption. This study investigates the combined effects of fructose and common food preservatives (sodium benzoate, sodium nitrite, and potassium sorbate) on the development and progression of MASLD. Methods: We utilized a human microbiota-associated mouse model, administering 10% fructose with or without preservatives for 11 weeks. Liver histology, hepatic gene expression (microarray analysis), biochemical markers, cytokine profiles, intestinal permeability, and gut microbiome composition (16S rRNA and Internal Transcribed Spacer (ITS) sequencing) were evaluated. Results: Fructose and potassium sorbate synergistically induced liver pathology characterized by increased steatosis, inflammation and fibrosis. These histological changes were associated with elevated liver function markers and altered lipid profiles. The treatments also induced significant changes in both the bacterial and fungal communities and disrupted intestinal barrier function, leading to increased pro-inflammatory responses in the mesenteric lymph nodes. Liver gene expression analysis revealed a wide range of transcriptional changes induced by fructose and modulated by the preservative. Key genes involved in lipid metabolism, oxidative stress, and inflammatory responses were affected. Conclusions: Our findings highlight the complex interactions between dietary components, gut microbiota, and host metabolism in the development of MASLD. The study identifies potential risks associated with the combined consumption of fructose and preservatives, particularly potassium sorbate. Our data reveal new mechanisms that are involved in the development of MASLD and open up a new avenue for the prevention and treatment of MASLD through dietary interventions and the modulation of the microbiome.
Keywords: food additives; fructose; gut microbiome; hepatic gene expression; inflammation; intestinal permeability; mycobiome; non-alcoholic fatty liver disease (NAFLD).
Conflict of interest statement
The authors declare no conflicts of interest.
Figures
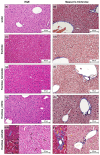
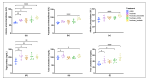
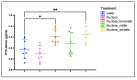
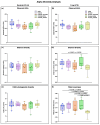
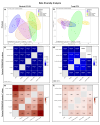
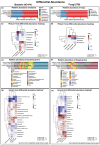
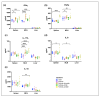
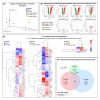
Similar articles
-
Exploring the Regulatory Effect of LPJZ-658 on Copper Deficiency Combined with Sugar-Induced MASLD in Middle-Aged Mice Based on Multi-Omics Analysis.Nutrients. 2024 Jun 25;16(13):2010. doi: 10.3390/nu16132010. Nutrients. 2024. PMID: 38999758 Free PMC article.
-
Dietary copper-fructose interactions alter gut microbial activity in male rats.Am J Physiol Gastrointest Liver Physiol. 2018 Jan 1;314(1):G119-G130. doi: 10.1152/ajpgi.00378.2016. Epub 2017 Oct 12. Am J Physiol Gastrointest Liver Physiol. 2018. PMID: 29025734 Free PMC article.
-
Lupeol improves bile acid metabolism and metabolic dysfunction-associated steatotic liver disease in mice via FXR signaling pathway and gut-liver axis.Biomed Pharmacother. 2024 Aug;177:116942. doi: 10.1016/j.biopha.2024.116942. Epub 2024 Jun 17. Biomed Pharmacother. 2024. PMID: 38889641
-
Breaking the barriers: the role of gut homeostasis in Metabolic-Associated Steatotic Liver Disease (MASLD).Gut Microbes. 2024 Jan-Dec;16(1):2331460. doi: 10.1080/19490976.2024.2331460. Epub 2024 Mar 21. Gut Microbes. 2024. PMID: 38512763 Free PMC article. Review.
-
Biomarkers for Health Functional Foods in Metabolic Dysfunction-Associated Steatotic Liver Disorder (MASLD) Prevention: An Integrative Analysis of Network Pharmacology, Gut Microbiota, and Multi-Omics.Nutrients. 2024 Sep 11;16(18):3061. doi: 10.3390/nu16183061. Nutrients. 2024. PMID: 39339660 Free PMC article. Review.
References
-
- Hrncir T., Hrncirova L., Kverka M., Hromadka R., Machova V., Trckova E., Kostovcikova K., Kralickova P., Krejsek J., Tlaskalova-Hogenova H. Gut Microbiota and NAFLD: Pathogenetic Mechanisms, Microbiota Signatures, and Therapeutic Interventions. Microorganisms. 2021;9:957. doi: 10.3390/microorganisms9050957. - DOI - PMC - PubMed
MeSH terms
Substances
Grants and funding
LinkOut - more resources
Full Text Sources