Development of a Machine Learning Model for Sonographic Assessment of Gestational Age
- PMID: 36598790
- PMCID: PMC9857195
- DOI: 10.1001/jamanetworkopen.2022.48685
Development of a Machine Learning Model for Sonographic Assessment of Gestational Age
Abstract
Importance: Fetal ultrasonography is essential for confirmation of gestational age (GA), and accurate GA assessment is important for providing appropriate care throughout pregnancy and for identifying complications, including fetal growth disorders. Derivation of GA from manual fetal biometry measurements (ie, head, abdomen, and femur) is operator dependent and time-consuming.
Objective: To develop artificial intelligence (AI) models to estimate GA with higher accuracy and reliability, leveraging standard biometry images and fly-to ultrasonography videos.
Design, setting, and participants: To improve GA estimates, this diagnostic study used AI to interpret standard plane ultrasonography images and fly-to ultrasonography videos, which are 5- to 10-second videos that can be automatically recorded as part of the standard of care before the still image is captured. Three AI models were developed and validated: (1) an image model using standard plane images, (2) a video model using fly-to videos, and (3) an ensemble model (combining both image and video models). The models were trained and evaluated on data from the Fetal Age Machine Learning Initiative (FAMLI) cohort, which included participants from 2 study sites at Chapel Hill, North Carolina (US), and Lusaka, Zambia. Participants were eligible to be part of this study if they received routine antenatal care at 1 of these sites, were aged 18 years or older, had a viable intrauterine singleton pregnancy, and could provide written consent. They were not eligible if they had known uterine or fetal abnormality, or had any other conditions that would make participation unsafe or complicate interpretation. Data analysis was performed from January to July 2022.
Main outcomes and measures: The primary analysis outcome for GA was the mean difference in absolute error between the GA model estimate and the clinical standard estimate, with the ground truth GA extrapolated from the initial GA estimated at an initial examination.
Results: Of the total cohort of 3842 participants, data were calculated for a test set of 404 participants with a mean (SD) age of 28.8 (5.6) years at enrollment. All models were statistically superior to standard fetal biometry-based GA estimates derived from images captured by expert sonographers. The ensemble model had the lowest mean absolute error compared with the clinical standard fetal biometry (mean [SD] difference, -1.51 [3.96] days; 95% CI, -1.90 to -1.10 days). All 3 models outperformed standard biometry by a more substantial margin on fetuses that were predicted to be small for their GA.
Conclusions and relevance: These findings suggest that AI models have the potential to empower trained operators to estimate GA with higher accuracy.
Conflict of interest statement
Figures
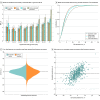
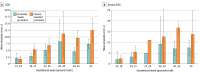
Similar articles
-
Diagnostic Accuracy of an Integrated AI Tool to Estimate Gestational Age From Blind Ultrasound Sweeps.JAMA. 2024 Aug 27;332(8):649-657. doi: 10.1001/jama.2024.10770. JAMA. 2024. PMID: 39088200
-
Deep learning to estimate gestational age from fly-to cineloop videos: A novel approach to ultrasound quality control.Int J Gynaecol Obstet. 2024 Jun;165(3):1013-1021. doi: 10.1002/ijgo.15321. Epub 2024 Jan 8. Int J Gynaecol Obstet. 2024. PMID: 38189177 Free PMC article.
-
Deep learning for estimation of fetal weight throughout the pregnancy from fetal abdominal ultrasound.Am J Obstet Gynecol MFM. 2023 Dec;5(12):101182. doi: 10.1016/j.ajogmf.2023.101182. Epub 2023 Oct 10. Am J Obstet Gynecol MFM. 2023. PMID: 37821009
-
Routine ultrasound for fetal assessment before 24 weeks' gestation.Cochrane Database Syst Rev. 2021 Aug 26;8(8):CD014698. doi: 10.1002/14651858.CD014698. Cochrane Database Syst Rev. 2021. PMID: 34438475 Free PMC article. Review.
-
Fetal growth velocity and body proportion in the assessment of growth.Am J Obstet Gynecol. 2018 Feb;218(2S):S700-S711.e1. doi: 10.1016/j.ajog.2017.12.014. Am J Obstet Gynecol. 2018. PMID: 29422209 Review.
Cited by
-
Advancements in Artificial Intelligence for Fetal Neurosonography: A Comprehensive Review.J Clin Med. 2024 Sep 22;13(18):5626. doi: 10.3390/jcm13185626. J Clin Med. 2024. PMID: 39337113 Free PMC article. Review.
-
Artificial intelligence assistance for fetal development: evaluation of an automated software for biometry measurements in the mid-trimester.BMC Pregnancy Childbirth. 2024 Feb 23;24(1):158. doi: 10.1186/s12884-024-06336-y. BMC Pregnancy Childbirth. 2024. PMID: 38395822 Free PMC article.
-
Prediction of hyperuricemia in people taking low-dose aspirin using a machine learning algorithm: a cross-sectional study of the National Health and Nutrition Examination Survey.Front Pharmacol. 2024 Jan 19;14:1276149. doi: 10.3389/fphar.2023.1276149. eCollection 2023. Front Pharmacol. 2024. PMID: 38313076 Free PMC article.
-
Evolving the Era of 5D Ultrasound? A Systematic Literature Review on the Applications for Artificial Intelligence Ultrasound Imaging in Obstetrics and Gynecology.J Clin Med. 2023 Oct 29;12(21):6833. doi: 10.3390/jcm12216833. J Clin Med. 2023. PMID: 37959298 Free PMC article. Review.
-
The relationship between placental thickness and gestational age in pregnant women: A cross-sectional study.Health Sci Rep. 2023 Apr 27;6(5):e1228. doi: 10.1002/hsr2.1228. eCollection 2023 May. Health Sci Rep. 2023. PMID: 37123553 Free PMC article.
References
-
- Ravishankar H, Prabhu SM, Vaidya V, Singhal N. Hybrid approach for automatic segmentation of fetal abdomen from ultrasound images using deep learning. 2016 IEEE 13th International Symposium on Biomedical Imaging (ISBI). June 16, 2016. doi:10.1109/ISBI.2016.7493382 - DOI