Deep learning to estimate gestational age from fly-to cineloop videos: A novel approach to ultrasound quality control
- PMID: 38189177
- PMCID: PMC11214162
- DOI: 10.1002/ijgo.15321
Deep learning to estimate gestational age from fly-to cineloop videos: A novel approach to ultrasound quality control
Abstract
Objective: Low-cost devices have made obstetric sonography possible in settings where it was previously unfeasible, but ensuring quality and consistency at scale remains a challenge. In the present study, we sought to create a tool to reduce substandard fetal biometry measurement while minimizing care disruption.
Methods: We developed a deep learning artificial intelligence (AI) model to estimate gestational age (GA) in the second and third trimester from fly-to cineloops-brief videos acquired during routine ultrasound biometry-and evaluated its performance in comparison to expert sonographer measurement. We then introduced random error into fetal biometry measurements and analyzed the ability of the AI model to flag grossly inaccurate measurements such as those that might be obtained by a novice.
Results: The mean absolute error (MAE) of our model (±standard error) was 3.87 ± 0.07 days, compared to 4.80 ± 0.10 days for expert biometry (difference -0.92 days; 95% CI: -1.10 to -0.76). Based on simulated novice biometry with average absolute error of 7.5%, our model reliably detected cases where novice biometry differed from expert biometry by 10 days or more, with an area under the receiver operating characteristics curve of 0.93 (95% CI: 0.92, 0.95), sensitivity of 81.0% (95% CI: 77.9, 83.8), and specificity of 89.9% (95% CI: 88.1, 91.5). These results held across a range of sensitivity analyses, including where the model was provided suboptimal truncated fly-to cineloops.
Conclusions: Our AI model estimated GA more accurately than expert biometry. Because fly-to cineloop videos can be obtained without any change to sonographer workflow, the model represents a no-cost guardrail that could be incorporated into both low-cost and commercial ultrasound devices to prevent reporting of most gross GA estimation errors.
Keywords: artificial intelligence; biometry; deep learning; gestational age; quality control; ultrasound.
© 2024 International Federation of Gynecology and Obstetrics.
Conflict of interest statement
Conflict of interest
The authors have no conflict of interest.
Figures
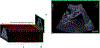
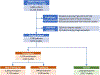
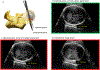
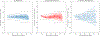
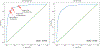
Similar articles
-
Depressing time: Waiting, melancholia, and the psychoanalytic practice of care.In: Kirtsoglou E, Simpson B, editors. The Time of Anthropology: Studies of Contemporary Chronopolitics. Abingdon: Routledge; 2020. Chapter 5. In: Kirtsoglou E, Simpson B, editors. The Time of Anthropology: Studies of Contemporary Chronopolitics. Abingdon: Routledge; 2020. Chapter 5. PMID: 36137063 Free Books & Documents. Review.
-
Development and validation of prediction models for fetal growth restriction and birthweight: an individual participant data meta-analysis.Health Technol Assess. 2024 Aug;28(47):1-119. doi: 10.3310/DABW4814. Health Technol Assess. 2024. PMID: 39252507 Free PMC article.
-
Dynamic Field Theory of Executive Function: Identifying Early Neurocognitive Markers.Monogr Soc Res Child Dev. 2024 Dec;89(3):7-109. doi: 10.1111/mono.12478. Monogr Soc Res Child Dev. 2024. PMID: 39628288 Free PMC article.
-
Falls prevention interventions for community-dwelling older adults: systematic review and meta-analysis of benefits, harms, and patient values and preferences.Syst Rev. 2024 Nov 26;13(1):289. doi: 10.1186/s13643-024-02681-3. Syst Rev. 2024. PMID: 39593159 Free PMC article.
-
Strategies to improve smoking cessation rates in primary care.Cochrane Database Syst Rev. 2021 Sep 6;9(9):CD011556. doi: 10.1002/14651858.CD011556.pub2. Cochrane Database Syst Rev. 2021. PMID: 34693994 Free PMC article. Review.
Cited by
-
Diagnostic Accuracy of an Integrated AI Tool to Estimate Gestational Age From Blind Ultrasound Sweeps.JAMA. 2024 Aug 27;332(8):649-657. doi: 10.1001/jama.2024.10770. JAMA. 2024. PMID: 39088200 Free PMC article.
-
Artificial Intelligence (AI) Applications for Point of Care Ultrasound (POCUS) in Low-Resource Settings: A Scoping Review.Diagnostics (Basel). 2024 Aug 1;14(15):1669. doi: 10.3390/diagnostics14151669. Diagnostics (Basel). 2024. PMID: 39125545 Free PMC article.
References
-
- WHO recommendations on antenatal care for a positive pregnancy experience. https://www.who.int/publications/i/item/9789241549912. Accessed 2023 Mar 30. - PubMed
-
- Becker DM, Tafoya CA, Becker SL, Kruger GH, Tafoya MJ, Becker TK. The use of portable ultrasound devices in low- and middle-income countries: a systematic review of the literature. Trop Med Int Health. 2016;21(3):294–311. - PubMed
MeSH terms
Grants and funding
LinkOut - more resources
Full Text Sources