ABOVE: The SNARC machine included 40 artificial neurons (one pictured), which were interconnected via a plugboard and held in racks in a contraption about the size of a grand piano. At one end of the neuron was a potentiometer (bar on far right), a sort of volume knob that could adjust the probability that an incoming signal would result in an outgoing signal. If the neuron did fire, a capacitor (red) on the other end of the neuron retained a memory of the firing for a few seconds. If the system was “rewarded”—either by the researchers pushing a button or an electrical signal from an outside circuit—a chain connected to the volume knobs for all 40 neurons would crank. This would cause the volume knob to increase the future probability of the neuron firing, but only if a magnetic clutch had been engaged by a recent firing.
COURTESY OF MARGARET...
As an undergraduate at Harvard in the late 1940s and in his first year of grad school at Princeton in 1950, Marvin Minsky pondered how to build a machine that could learn. At both universities, Minsky studied mathematics, but he was curious about the human mind—what he saw as the most profound mystery in science. He wanted to better understand intelligence by recreating it.
In the summer of 1951, he got his chance. George Miller, an up-and-coming psychologist at Harvard, secured funding for Minsky to return to Boston for the summer and build his device. Minsky enlisted the help of fellow Princeton graduate student Dean Edmonds, and the duo crafted what would become known as the first artificial neural network. Called SNARC, for stochastic neural-analog reinforcement calculator, the network included 40 interconnected artificial neurons, each of which had short-term and long-term memory of sorts. The short-term memory came in the form of a capacitor, a piece of hardware that stores electrical energy, that could remember for a few seconds if the neuron had recently relayed a signal. Long-term memory was handled by a potentiometer, or volume knob, that would increase a neuron’s probability of relaying a signal if it had just fired when the system was “rewarded,” either manually or through an automated electrical signal.
Minsky and Edmonds tested the model’s ability to learn a maze. The details of how the young researchers tracked the output are unclear, but one theory is that they observed, through an arrangement of lights, how a signal moved through the network from a random starting place in the neural network to a predetermined finish line. The duo referred to the signal as “rats” running through a maze of tunnels. When the rats followed a path that led toward the finish line, the system adjusted to increase the likelihood of that firing pattern happening again. Sure enough, the rats began making fewer wrong turns. Multiple rats could run at once to increase the speed at which the system learned.
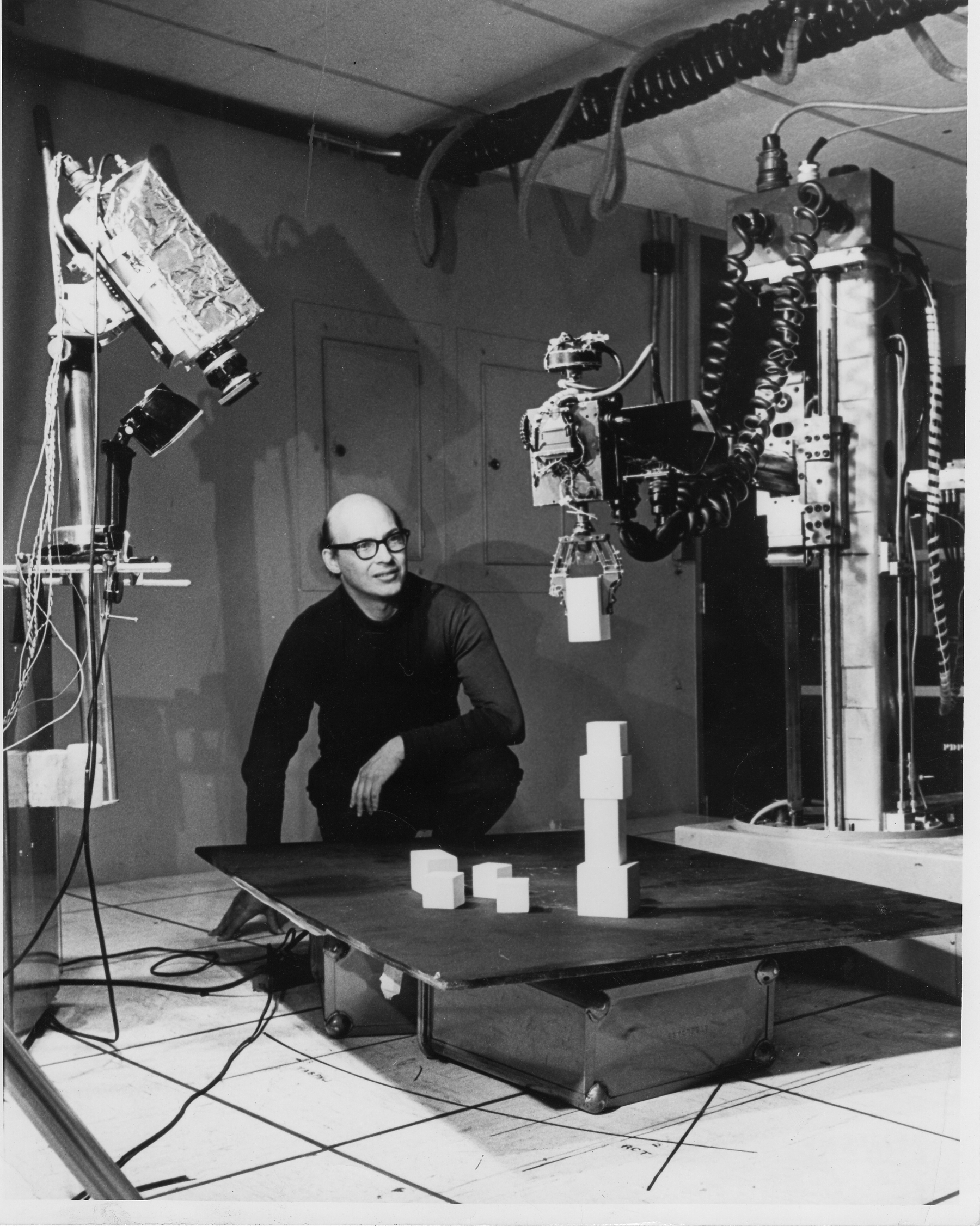
“We sort of quit science for a while to watch the machine,” Minsky told the New Yorker in a 1981 profile. “We were amazed that it could have several activities going on at once in its little nervous system.”
Artificial neural networks continued to advance over the next seven decades, but it’s only been since the turn of the 21st century that the approach has taken center stage in the field of artificial intelligence. Deep neural networks, which involve many layers of computation, are now powering myriad applications, including ever-more-realistic models of the human brain. (See “Building a Silicon Brain.”) But nearly 70 years ago, Minsky felt neural networks were too limited because they didn’t have a sense of expectation, which is critical for filling in gaps in human perception. He shifted his focus to symbolic artificial intelligence, which, instead of trying to mimic the brain’s approach to information processing, draws inspiration from the nature of human thought, doing computations based on high-level concepts that are interpretable by people.
Minsky, who died in 2016, did think that neural networks would be one component of a truly intelligent machine, notes his daughter, interactive computing expert Margaret Minsky, a visiting professor at New York University Shanghai. She wonders what he would think about that today. “Given the things [artificial neural networks] do and don’t do, and how they fit into systems now, I really want to know what he would say.”
Interested in reading more?
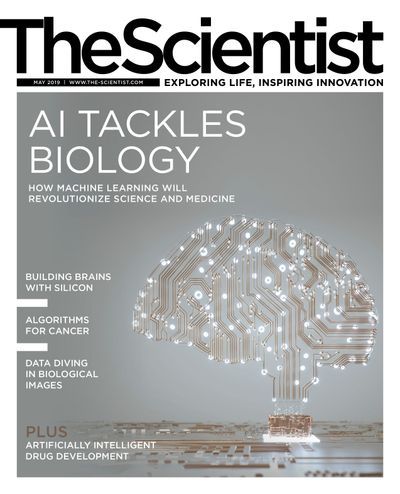