Exercise-changed gut mycobiome as a potential contributor to metabolic benefits in diabetes prevention: an integrative multi-omics study
- PMID: 39473051
- PMCID: PMC11533799
- DOI: 10.1080/19490976.2024.2416928
Exercise-changed gut mycobiome as a potential contributor to metabolic benefits in diabetes prevention: an integrative multi-omics study
Abstract
Background: The importance of gut microbes in mediating the benefits of lifestyle intervention is increasingly recognized. However, compared to the bacterial microbiome, the role of intestinal fungi in exercise remains elusive. With our established randomized controlled trial of exercise intervention in Chinese males with prediabetes (n = 39, ClinicalTrials.gov:NCT03240978), we investigated the dynamics of human gut mycobiome and further interrogated their associations with exercise-elicited outcomes using multi-omics approaches.
Methods: Clinical variations and biological samples were collected before and after training. Fecal fungal composition was analyzed using the internal transcribed spacer 2 (ITS2) sequencing and integrated with paired shotgun metagenomics, untargeted metabolomics, and Olink proteomics.
Results: Twelve weeks of exercise training profoundly promoted fungal ecological diversity and intrakingdom connection. We further identified exercise-responsive genera with potential metabolic benefits, including Verticillium, Sarocladium, and Ceratocystis. Using multi-omics approaches, we elucidated comprehensive associations between changes in gut mycobiome and exercise-shaped metabolic phenotypes, bacterial microbiome, and circulating metabolomics and proteomics profiles. Furthermore, a machine-learning algorithm built using baseline microbial signatures and clinical characteristics predicted exercise responsiveness in improvements of insulin sensitivity, with an area under the receiver operating characteristic (AUROC) of 0.91 (95% CI: 0.85-0.97) in the discovery cohort and of 0.79 (95% CI: 0.74-0.86) in the independent validation cohort (n = 30).
Conclusions: Our findings suggest that intense exercise training significantly remodels the human fungal microbiome composition. Changes in gut fungal composition are associated with the metabolic benefits of exercise, indicating gut mycobiome is a possible molecular transducer of exercise. Moreover, baseline gut fungal signatures predict exercise responsiveness for diabetes prevention, highlighting that targeting the gut mycobiome emerges as a prospective strategy in tailoring personalized training for diabetes prevention.
Keywords: Gut mycobiome; diabetes prevention; exercise training; fungal microbiome; intervention responsiveness; multi-omics; randomized controlled trial.
Conflict of interest statement
No potential conflict of interest was reported by the author(s).
Figures
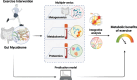
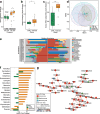
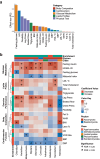
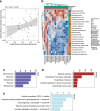
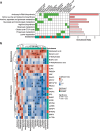
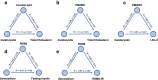
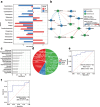
Similar articles
-
The gut mycobiome of the Human Microbiome Project healthy cohort.Microbiome. 2017 Nov 25;5(1):153. doi: 10.1186/s40168-017-0373-4. Microbiome. 2017. PMID: 29178920 Free PMC article.
-
An integrative multi-omic analysis defines gut microbiota, mycobiota, and metabolic fingerprints in ulcerative colitis patients.Front Cell Infect Microbiol. 2024 May 8;14:1366192. doi: 10.3389/fcimb.2024.1366192. eCollection 2024. Front Cell Infect Microbiol. 2024. PMID: 38779566 Free PMC article.
-
Changes in the type 2 diabetes gut mycobiome associate with metformin treatment across populations.mBio. 2024 Jun 12;15(6):e0016924. doi: 10.1128/mbio.00169-24. Epub 2024 May 20. mBio. 2024. PMID: 38767350 Free PMC article.
-
Diabetic foot exacerbates gut mycobiome dysbiosis in adult patients with type 2 diabetes mellitus: revealing diagnostic markers.Nutr Diabetes. 2024 Sep 3;14(1):71. doi: 10.1038/s41387-024-00328-9. Nutr Diabetes. 2024. PMID: 39223127 Free PMC article. Review.
-
Gut mycobiome in metabolic diseases: Mechanisms and clinical implication.Biomed J. 2024 Jun;47(3):100625. doi: 10.1016/j.bj.2023.100625. Epub 2023 Jun 25. Biomed J. 2024. PMID: 37364760 Free PMC article. Review.
References
Publication types
MeSH terms
Associated data
LinkOut - more resources
Full Text Sources
Medical