Quantifying Parkinson's disease motor severity under uncertainty using MDS-UPDRS videos
- PMID: 34340101
- PMCID: PMC8453121
- DOI: 10.1016/j.media.2021.102179
Quantifying Parkinson's disease motor severity under uncertainty using MDS-UPDRS videos
Abstract
Parkinson's disease (PD) is a brain disorder that primarily affects motor function, leading to slow movement, tremor, and stiffness, as well as postural instability and difficulty with walking/balance. The severity of PD motor impairments is clinically assessed by part III of the Movement Disorder Society Unified Parkinson's Disease Rating Scale (MDS-UPDRS), a universally-accepted rating scale. However, experts often disagree on the exact scoring of individuals. In the presence of label noise, training a machine learning model using only scores from a single rater may introduce bias, while training models with multiple noisy ratings is a challenging task due to the inter-rater variabilities. In this paper, we introduce an ordinal focal neural network to estimate the MDS-UPDRS scores from input videos, to leverage the ordinal nature of MDS-UPDRS scores and combat class imbalance. To handle multiple noisy labels per exam, the training of the network is regularized via rater confusion estimation (RCE), which encodes the rating habits and skills of raters via a confusion matrix. We apply our pipeline to estimate MDS-UPDRS test scores from their video recordings including gait (with multiple Raters, R=3) and finger tapping scores (single rater). On a sizable clinical dataset for the gait test (N=55), we obtained a classification accuracy of 72% with majority vote as ground-truth, and an accuracy of ∼84% of our model predicting at least one of the raters' scores. Our work demonstrates how computer-assisted technologies can be used to track patients and their motor impairments, even when there is uncertainty in the clinical ratings. The latest version of the code will be available at https://github.com/mlu355/PD-Motor-Severity-Estimation.
Keywords: Computer vision; Finger tapping; Gait analysis; Movement disorder society Unified Parkinsons Disease Rating Scale; Uncertainty.
Copyright © 2021. Published by Elsevier B.V.
Conflict of interest statement
Declaration of Competing Interest The authors declare that they have no known competing financial interests or personal relationships that could have appeared to influence the work reported in this paper.
Figures
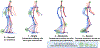
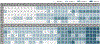
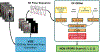
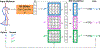
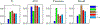
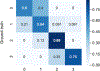
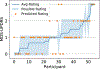
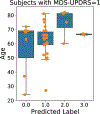
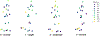
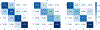
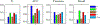
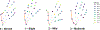
Similar articles
-
Vision-based estimation of MDS-UPDRS scores for quantifying Parkinson's disease tremor severity.Med Image Anal. 2023 Apr;85:102754. doi: 10.1016/j.media.2023.102754. Epub 2023 Jan 20. Med Image Anal. 2023. PMID: 36702036
-
Vision-based Estimation of MDS-UPDRS Gait Scores for Assessing Parkinson's Disease Motor Severity.Med Image Comput Comput Assist Interv. 2020 Oct;12263:637-647. doi: 10.1007/978-3-030-59716-0_61. Epub 2020 Sep 29. Med Image Comput Comput Assist Interv. 2020. PMID: 33103164 Free PMC article.
-
Treatment Detection and Movement Disorder Society-Unified Parkinson's Disease Rating Scale, Part III Estimation Using Finger Tapping Tasks.Mov Disord. 2023 Oct;38(10):1795-1805. doi: 10.1002/mds.29520. Epub 2023 Jul 4. Mov Disord. 2023. PMID: 37401265
-
The Unified Parkinson's Disease Rating Scale (UPDRS): status and recommendations.Mov Disord. 2003 Jul;18(7):738-50. doi: 10.1002/mds.10473. Mov Disord. 2003. PMID: 12815652 Review.
-
Deep Learning for hand tracking in Parkinson's Disease video-based assessment: Current and future perspectives.Artif Intell Med. 2024 Aug;154:102914. doi: 10.1016/j.artmed.2024.102914. Epub 2024 Jun 17. Artif Intell Med. 2024. PMID: 38909431 Review.
Cited by
-
Deep Brain Stimulation restores information processing in parkinsonian cortical networks.medRxiv [Preprint]. 2024 Aug 28:2024.08.25.24310748. doi: 10.1101/2024.08.25.24310748. medRxiv. 2024. PMID: 39252923 Free PMC article. Preprint.
-
Assisted technology in Parkinson's disease gait: what's up?Arq Neuropsiquiatr. 2024 Jun;82(6):1-10. doi: 10.1055/s-0043-1777782. Epub 2024 Feb 23. Arq Neuropsiquiatr. 2024. PMID: 38395424 Free PMC article. Review.
-
Computer Vision for Parkinson's Disease Evaluation: A Survey on Finger Tapping.Healthcare (Basel). 2024 Feb 8;12(4):439. doi: 10.3390/healthcare12040439. Healthcare (Basel). 2024. PMID: 38391815 Free PMC article. Review.
-
Pose-based tremor type and level analysis for Parkinson's disease from video.Int J Comput Assist Radiol Surg. 2024 May;19(5):831-840. doi: 10.1007/s11548-023-03052-4. Epub 2024 Jan 18. Int J Comput Assist Radiol Surg. 2024. PMID: 38238490 Free PMC article.
-
Evaluating the ability of a predictive vision-based machine learning model to measure changes in gait in response to medication and DBS within individuals with Parkinson's disease.Biomed Eng Online. 2023 Dec 11;22(1):120. doi: 10.1186/s12938-023-01175-y. Biomed Eng Online. 2023. PMID: 38082277 Free PMC article.
References
-
- Adeli V, Adeli E, Reid I, Niebles JC, Rezatofighi H, 2020. Socially and contextually aware human motion and pose forecasting. IEEE Robotics and Automation Letters 5, 6033–6040.
-
- Andriluka M, Pishchulin L, Gehler P, Schiele B, 2014. 2d human pose estimation: New benchmark and state of the art analysis, in: Proceedings of the IEEE Conference on computer Vision and Pattern Recognition, pp. 3686–3693.
-
- Anscombe FJ, 1948. The validity of comparative experiments. Journal of the royal statistical society. series A (General) 111, 181–211. - PubMed
-
- Arteta C, Lempitsky V, Zisserman A, 2016. Counting in the wild, in: European conference on computer vision, Springer. pp. 483–498.
Publication types
MeSH terms
Grants and funding
LinkOut - more resources
Full Text Sources
Medical
Research Materials
Miscellaneous