Clinically Applicable Segmentation of Head and Neck Anatomy for Radiotherapy: Deep Learning Algorithm Development and Validation Study
- PMID: 34255661
- PMCID: PMC8314151
- DOI: 10.2196/26151
Clinically Applicable Segmentation of Head and Neck Anatomy for Radiotherapy: Deep Learning Algorithm Development and Validation Study
Abstract
Background: Over half a million individuals are diagnosed with head and neck cancer each year globally. Radiotherapy is an important curative treatment for this disease, but it requires manual time to delineate radiosensitive organs at risk. This planning process can delay treatment while also introducing interoperator variability, resulting in downstream radiation dose differences. Although auto-segmentation algorithms offer a potentially time-saving solution, the challenges in defining, quantifying, and achieving expert performance remain.
Objective: Adopting a deep learning approach, we aim to demonstrate a 3D U-Net architecture that achieves expert-level performance in delineating 21 distinct head and neck organs at risk commonly segmented in clinical practice.
Methods: The model was trained on a data set of 663 deidentified computed tomography scans acquired in routine clinical practice and with both segmentations taken from clinical practice and segmentations created by experienced radiographers as part of this research, all in accordance with consensus organ at risk definitions.
Results: We demonstrated the model's clinical applicability by assessing its performance on a test set of 21 computed tomography scans from clinical practice, each with 21 organs at risk segmented by 2 independent experts. We also introduced surface Dice similarity coefficient, a new metric for the comparison of organ delineation, to quantify the deviation between organ at risk surface contours rather than volumes, better reflecting the clinical task of correcting errors in automated organ segmentations. The model's generalizability was then demonstrated on 2 distinct open-source data sets, reflecting different centers and countries to model training.
Conclusions: Deep learning is an effective and clinically applicable technique for the segmentation of the head and neck anatomy for radiotherapy. With appropriate validation studies and regulatory approvals, this system could improve the efficiency, consistency, and safety of radiotherapy pathways.
Keywords: UNet; artificial intelligence; contouring; convolutional neural networks; machine learning; radiotherapy; segmentation; surface DSC.
©Stanislav Nikolov, Sam Blackwell, Alexei Zverovitch, Ruheena Mendes, Michelle Livne, Jeffrey De Fauw, Yojan Patel, Clemens Meyer, Harry Askham, Bernadino Romera-Paredes, Christopher Kelly, Alan Karthikesalingam, Carlton Chu, Dawn Carnell, Cheng Boon, Derek D'Souza, Syed Ali Moinuddin, Bethany Garie, Yasmin McQuinlan, Sarah Ireland, Kiarna Hampton, Krystle Fuller, Hugh Montgomery, Geraint Rees, Mustafa Suleyman, Trevor Back, Cían Owen Hughes, Joseph R Ledsam, Olaf Ronneberger. Originally published in the Journal of Medical Internet Research (https://www.jmir.org), 12.07.2021.
Conflict of interest statement
Conflicts of Interest: GR, HM, CK, COH, and DC were paid contractors of DeepMind and Google Health.
Figures
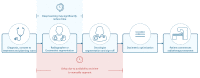
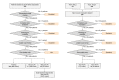
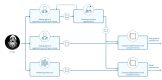
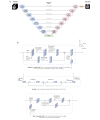
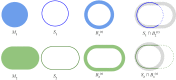
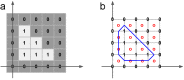
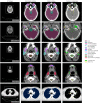
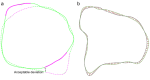
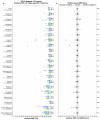
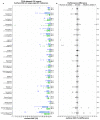
Similar articles
-
Fully automatic multi-organ segmentation for head and neck cancer radiotherapy using shape representation model constrained fully convolutional neural networks.Med Phys. 2018 Oct;45(10):4558-4567. doi: 10.1002/mp.13147. Epub 2018 Sep 19. Med Phys. 2018. PMID: 30136285 Free PMC article.
-
Cascaded deep learning-based auto-segmentation for head and neck cancer patients: Organs at risk on T2-weighted magnetic resonance imaging.Med Phys. 2021 Dec;48(12):7757-7772. doi: 10.1002/mp.15290. Epub 2021 Nov 1. Med Phys. 2021. PMID: 34676555
-
AnatomyNet: Deep learning for fast and fully automated whole-volume segmentation of head and neck anatomy.Med Phys. 2019 Feb;46(2):576-589. doi: 10.1002/mp.13300. Epub 2018 Dec 17. Med Phys. 2019. PMID: 30480818
-
Auto-segmentation of organs at risk for head and neck radiotherapy planning: From atlas-based to deep learning methods.Med Phys. 2020 Sep;47(9):e929-e950. doi: 10.1002/mp.14320. Epub 2020 Jul 28. Med Phys. 2020. PMID: 32510603 Review.
-
Deep learning algorithm performance in contouring head and neck organs at risk: a systematic review and single-arm meta-analysis.Biomed Eng Online. 2023 Nov 1;22(1):104. doi: 10.1186/s12938-023-01159-y. Biomed Eng Online. 2023. PMID: 37915046 Free PMC article. Review.
Cited by
-
Evaluation of deep learning-based target auto-segmentation for Magnetic Resonance Imaging-guided cervix brachytherapy.Phys Imaging Radiat Oncol. 2024 Nov 3;32:100669. doi: 10.1016/j.phro.2024.100669. eCollection 2024 Oct. Phys Imaging Radiat Oncol. 2024. PMID: 39559487 Free PMC article.
-
Retrospective Comparison of Geometrical Accuracy among Atlas-based Auto-segmentation, Deep Learning Auto-segmentation, and Deformable Image Registration in the Treatment Replanning for Adaptive Radiotherapy of Head-and-Neck Cancer.J Med Phys. 2024 Jul-Sep;49(3):335-342. doi: 10.4103/jmp.jmp_39_24. Epub 2024 Sep 21. J Med Phys. 2024. PMID: 39526151 Free PMC article.
-
OAR-Weighted Dice Score: A spatially aware, radiosensitivity aware metric for target structure contour quality assessment.Use Comput Radiat Ther. 2024 Jul;2024:755-758. Use Comput Radiat Ther. 2024. PMID: 39512542 Free PMC article.
-
AI-Generated Annotations Dataset for Diverse Cancer Radiology Collections in NCI Image Data Commons.Sci Data. 2024 Oct 23;11(1):1165. doi: 10.1038/s41597-024-03977-8. Sci Data. 2024. PMID: 39443503 Free PMC article.
-
Proton Therapy Adaptation of Perisinusoidal and Brain Areas in the Cyclotron Centre Bronowice in Krakow: A Dosimetric Analysis.Cancers (Basel). 2024 Sep 11;16(18):3128. doi: 10.3390/cancers16183128. Cancers (Basel). 2024. PMID: 39335100 Free PMC article.
References
-
- Head and neck cancers incidence statistics. Cancer Research UK. [2018-02-08]. https://www.cancerresearchuk.org/health-professional/cancer-statistics/s....
-
- NCIN data briefing: potentially HPV-related head and neck cancers. National Cancer Intelligence Network. [2021-05-17]. http://www.ncin.org.uk/publications/data_briefings/potentially_hpv_relat....
-
- Profile of head and neck cancers in England: incidence, mortality and survival. Oxford Cancer Intelligence Unit. 2010. [2021-05-17]. http://www.ncin.org.uk/view?rid=69.
-
- Parkin DM, Boyd L, Walker LC. 16. The fraction of cancer attributable to lifestyle and environmental factors in the UK in 2010. Br J Cancer. 2011 Dec 06;105 Suppl 2:77–81. doi: 10.1038/bjc.2011.489. http://europepmc.org/abstract/MED/22158327 - DOI - PMC - PubMed
MeSH terms
LinkOut - more resources
Full Text Sources
Medical