Vision-based Estimation of MDS-UPDRS Gait Scores for Assessing Parkinson's Disease Motor Severity
- PMID: 33103164
- PMCID: PMC7585545
- DOI: 10.1007/978-3-030-59716-0_61
Vision-based Estimation of MDS-UPDRS Gait Scores for Assessing Parkinson's Disease Motor Severity
Abstract
Parkinson's disease (PD) is a progressive neurological disorder primarily affecting motor function resulting in tremor at rest, rigidity, bradykinesia, and postural instability. The physical severity of PD impairments can be quantified through the Movement Disorder Society Unified Parkinson's Disease Rating Scale (MDS-UPDRS), a widely used clinical rating scale. Accurate and quantitative assessment of disease progression is critical to developing a treatment that slows or stops further advancement of the disease. Prior work has mainly focused on dopamine transport neuroimaging for diagnosis or costly and intrusive wearables evaluating motor impairments. For the first time, we propose a computer vision-based model that observes non-intrusive video recordings of individuals, extracts their 3D body skeletons, tracks them through time, and classifies the movements according to the MDS-UPDRS gait scores. Experimental results show that our proposed method performs significantly better than chance and competing methods with an F 1-score of 0.83 and a balanced accuracy of 81%. This is the first benchmark for classifying PD patients based on MDS-UPDRS gait severity and could be an objective biomarker for disease severity. Our work demonstrates how computer-assisted technologies can be used to non-intrusively monitor patients and their motor impairments. The code is available at https://github.com/mlu355/PD-Motor-Severity-Estimation.
Keywords: Computer Vision; Gait Analysis; Movement Disorder Society Unified Parkinson’s Disease Rating Scale.
Figures
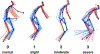
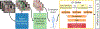
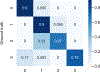
Similar articles
-
Quantifying Parkinson's disease motor severity under uncertainty using MDS-UPDRS videos.Med Image Anal. 2021 Oct;73:102179. doi: 10.1016/j.media.2021.102179. Epub 2021 Jul 21. Med Image Anal. 2021. PMID: 34340101 Free PMC article.
-
Vision-based estimation of MDS-UPDRS scores for quantifying Parkinson's disease tremor severity.Med Image Anal. 2023 Apr;85:102754. doi: 10.1016/j.media.2023.102754. Epub 2023 Jan 20. Med Image Anal. 2023. PMID: 36702036
-
How to identify tremor dominant and postural instability/gait difficulty groups with the movement disorder society unified Parkinson's disease rating scale: comparison with the unified Parkinson's disease rating scale.Mov Disord. 2013 May;28(5):668-70. doi: 10.1002/mds.25383. Epub 2013 Feb 13. Mov Disord. 2013. PMID: 23408503
-
Deep Learning for hand tracking in Parkinson's Disease video-based assessment: Current and future perspectives.Artif Intell Med. 2024 Aug;154:102914. doi: 10.1016/j.artmed.2024.102914. Epub 2024 Jun 17. Artif Intell Med. 2024. PMID: 38909431 Review.
-
Neurological Examination via Telemedicine: An Updated Review Focusing on Movement Disorders.Medicina (Kaunas). 2024 Jun 9;60(6):958. doi: 10.3390/medicina60060958. Medicina (Kaunas). 2024. PMID: 38929575 Free PMC article. Review.
Cited by
-
Clinically Informed Automated Assessment of Finger Tapping Videos in Parkinson's Disease.Sensors (Basel). 2023 Nov 13;23(22):9149. doi: 10.3390/s23229149. Sensors (Basel). 2023. PMID: 38005535 Free PMC article.
-
GaitForeMer: Self-Supervised Pre-Training of Transformers via Human Motion Forecasting for Few-Shot Gait Impairment Severity Estimation.Med Image Comput Comput Assist Interv. 2022 Sep;13438:130-139. doi: 10.1007/978-3-031-16452-1_13. Epub 2022 Sep 16. Med Image Comput Comput Assist Interv. 2022. PMID: 36342887 Free PMC article.
-
An Explainable Geometric-Weighted Graph Attention Network for Identifying Functional Networks Associated with Gait Impairment.Med Image Comput Comput Assist Interv. 2023 Oct;14221:723-733. doi: 10.1007/978-3-031-43895-0_68. Epub 2023 Oct 1. Med Image Comput Comput Assist Interv. 2023. PMID: 37982132 Free PMC article.
-
Computer vision quantification of whole-body Parkinsonian bradykinesia using a large multi-site population.NPJ Parkinsons Dis. 2023 Jan 27;9(1):10. doi: 10.1038/s41531-023-00454-8. NPJ Parkinsons Dis. 2023. PMID: 36707523 Free PMC article.
-
The Parkinson's disease waiting room of the future: measurements, not magazines.Front Neurol. 2023 Aug 21;14:1212113. doi: 10.3389/fneur.2023.1212113. eCollection 2023. Front Neurol. 2023. PMID: 37670776 Free PMC article.
References
-
- Andriluka M, Pishchulin L, Gehler P, Schiele B: 2d human pose estimation: New benchmark and state of the art analysis. In: Proceedings of the IEEE Conference on computer Vision and Pattern Recognition pp. 3686–3693 (2014)
-
- Bewley A, Ge Z, Ott L, Ramos F, Upcroft B: Simple online and realtime tracking In: ICIP. pp. 3464–3468. IEEE (2016)
-
- Bogo F, Kanazawa A, Lassner C, Gehler P, Romero J, Black MJ: Keep it smpl: Automatic estimation of 3d human pose and shape from a single image In: ECCV. pp. 561–578. Springer; (2016)
Grants and funding
LinkOut - more resources
Full Text Sources
Research Materials
Miscellaneous