ReactomeGSA - Efficient Multi-Omics Comparative Pathway Analysis
- PMID: 32907876
- PMCID: PMC7710148
- DOI: 10.1074/mcp.TIR120.002155
ReactomeGSA - Efficient Multi-Omics Comparative Pathway Analysis
Abstract
Pathway analyses are key methods to analyze 'omics experiments. Nevertheless, integrating data from different 'omics technologies and different species still requires considerable bioinformatics knowledge.Here we present the novel ReactomeGSA resource for comparative pathway analyses of multi-omics datasets. ReactomeGSA can be used through Reactome's existing web interface and the novel ReactomeGSA R Bioconductor package with explicit support for scRNA-seq data. Data from different species is automatically mapped to a common pathway space. Public data from ExpressionAtlas and Single Cell ExpressionAtlas can be directly integrated in the analysis. ReactomeGSA greatly reduces the technical barrier for multi-omics, cross-species, comparative pathway analyses.We used ReactomeGSA to characterize the role of B cells in anti-tumor immunity. We compared B cell rich and poor human cancer samples from five of the Cancer Genome Atlas (TCGA) transcriptomics and two of the Clinical Proteomic Tumor Analysis Consortium (CPTAC) proteomics studies. B cell-rich lung adenocarcinoma samples lacked the otherwise present activation through NFkappaB. This may be linked to the presence of a specific subset of tumor associated IgG+ plasma cells that lack NFkappaB activation in scRNA-seq data from human melanoma. This showcases how ReactomeGSA can derive novel biomedical insights by integrating large multi-omics datasets.
Keywords: Pathway analysis; bioinformatics software; cancer biology*; cancer immunology; data evaluation; melanoma; multi-omics data integration; tumor microenvironment.
© 2020 Griss et al.
Conflict of interest statement
Conflict of interest—The authors declare that they have no conflicts of interest with the contents of this article.
Figures
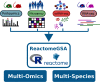
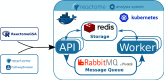
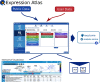
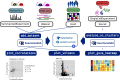
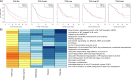
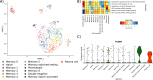
Similar articles
-
ReactomeGSA: new features to simplify public data reuse.Bioinformatics. 2024 Jun 3;40(6):btae338. doi: 10.1093/bioinformatics/btae338. Bioinformatics. 2024. PMID: 38806182 Free PMC article.
-
A User-Friendly Visualization Tool for Multi-Omics Data.Proteomics. 2020 Nov;20(21-22):e2000136. doi: 10.1002/pmic.202000136. Epub 2020 Sep 3. Proteomics. 2020. PMID: 32744797
-
LinkedOmics: analyzing multi-omics data within and across 32 cancer types.Nucleic Acids Res. 2018 Jan 4;46(D1):D956-D963. doi: 10.1093/nar/gkx1090. Nucleic Acids Res. 2018. PMID: 29136207 Free PMC article.
-
Web-based multi-omics integration using the Analyst software suite.Nat Protoc. 2024 May;19(5):1467-1497. doi: 10.1038/s41596-023-00950-4. Epub 2024 Feb 14. Nat Protoc. 2024. PMID: 38355833 Review.
-
Network-Based Approaches for Multi-omics Integration.Methods Mol Biol. 2020;2104:469-487. doi: 10.1007/978-1-0716-0239-3_23. Methods Mol Biol. 2020. PMID: 31953831 Review.
Cited by
-
Proteomic Profiling of Endothelial Cell Secretomes After Exposure to Calciprotein Particles Reveals Downregulation of Basement Membrane Assembly and Increased Release of Soluble CD59.Int J Mol Sci. 2024 Oct 23;25(21):11382. doi: 10.3390/ijms252111382. Int J Mol Sci. 2024. PMID: 39518935 Free PMC article.
-
Combination of bulk RNA and single-cell sequencing unveils PANoptosis-related immunological ecology hallmarks and classification for clinical decision-making in hepatocellular carcinoma.Sci Rep. 2024 Sep 28;14(1):22517. doi: 10.1038/s41598-024-73847-1. Sci Rep. 2024. PMID: 39342037 Free PMC article.
-
DDI2 protease controls embryonic development and inflammation via TCF11/NRF1.iScience. 2024 Sep 5;27(10):110893. doi: 10.1016/j.isci.2024.110893. eCollection 2024 Oct 18. iScience. 2024. PMID: 39328932 Free PMC article.
-
Proteomic profiling of oleamide-mediated polarization in a primary human monocyte-derived tumor-associated macrophages (TAMs) model: a functional analysis.PeerJ. 2024 Sep 18;12:e18090. doi: 10.7717/peerj.18090. eCollection 2024. PeerJ. 2024. PMID: 39308806 Free PMC article.
-
Scoping Review: Methods and Applications of Spatial Transcriptomics in Tumor Research.Cancers (Basel). 2024 Sep 6;16(17):3100. doi: 10.3390/cancers16173100. Cancers (Basel). 2024. PMID: 39272958 Free PMC article. Review.
References
-
- Subramanian A., Tamayo P., Mootha V. K., Mukherjee S., Ebert B. L., Gillette M. A., Paulovich A., Pomeroy S. L., Golub T. R., Lander E. S., and Mesirov J. P. (2005) Gene set enrichment analysis: a knowledge-based approach for interpreting genome-wide expression profiles. Proc. Natl. Acad. Sci. U S A. 102, 15545–15550 - PMC - PubMed
-
- Jassal B., Matthews L., Viteri G., Gong C., Lorente P., Fabregat A., Sidiropoulos K., Cook J., Gillespie M., Haw R., Loney F., May B., Milacic M., Rothfels K., Sevilla C., Shamovsky V., Shorser S., Varusai T., Weiser J., Wu G., Stein L., Hermjakob H., and D'Eustachio P. (2020) The reactome pathway knowledgebase. Nucleic Acids Res. 48, D498–D503 - PMC - PubMed
Publication types
MeSH terms
Grants and funding
LinkOut - more resources
Full Text Sources
Other Literature Sources
Miscellaneous