Biological insights from 108 schizophrenia-associated genetic loci
- PMID: 25056061
- PMCID: PMC4112379
- DOI: 10.1038/nature13595
Biological insights from 108 schizophrenia-associated genetic loci
Abstract
Schizophrenia is a highly heritable disorder. Genetic risk is conferred by a large number of alleles, including common alleles of small effect that might be detected by genome-wide association studies. Here we report a multi-stage schizophrenia genome-wide association study of up to 36,989 cases and 113,075 controls. We identify 128 independent associations spanning 108 conservatively defined loci that meet genome-wide significance, 83 of which have not been previously reported. Associations were enriched among genes expressed in brain, providing biological plausibility for the findings. Many findings have the potential to provide entirely new insights into aetiology, but associations at DRD2 and several genes involved in glutamatergic neurotransmission highlight molecules of known and potential therapeutic relevance to schizophrenia, and are consistent with leading pathophysiological hypotheses. Independent of genes expressed in brain, associations were enriched among genes expressed in tissues that have important roles in immunity, providing support for the speculated link between the immune system and schizophrenia.
Figures
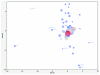
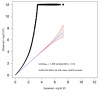
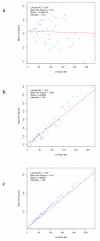
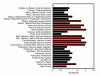
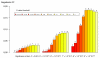
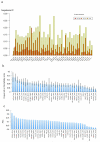
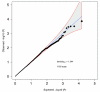
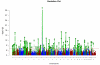
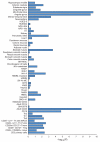
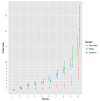
Comment in
-
Schizophrenia: genesis of a complex disease.Nature. 2014 Jul 24;511(7510):412-3. doi: 10.1038/nature13645. Epub 2014 Jul 22. Nature. 2014. PMID: 25056056 No abstract available.
-
Genetics: finding genes for schizophrenia.Curr Biol. 2014 Aug 18;24(16):R755-7. doi: 10.1016/j.cub.2014.07.018. Curr Biol. 2014. PMID: 25137590 Free PMC article.
-
Schizophrenia genetics comes of age.Neuron. 2014 Aug 20;83(4):760-3. doi: 10.1016/j.neuron.2014.08.015. Neuron. 2014. PMID: 25144873
-
Pamela Sklar (1959-2017).Nature. 2018 Feb 1;554(7690):32. doi: 10.1038/d41586-018-01382-x. Nature. 2018. PMID: 29388987 No abstract available.
Similar articles
-
Genetics: finding genes for schizophrenia.Curr Biol. 2014 Aug 18;24(16):R755-7. doi: 10.1016/j.cub.2014.07.018. Curr Biol. 2014. PMID: 25137590 Free PMC article.
-
Genome-wide analysis reveals extensive genetic overlap between schizophrenia, bipolar disorder, and intelligence.Mol Psychiatry. 2020 Apr;25(4):844-853. doi: 10.1038/s41380-018-0332-x. Epub 2019 Jan 4. Mol Psychiatry. 2020. PMID: 30610197 Free PMC article.
-
Genetic evidence for role of integration of fast and slow neurotransmission in schizophrenia.Mol Psychiatry. 2017 Jun;22(6):792-801. doi: 10.1038/mp.2017.33. Epub 2017 Mar 28. Mol Psychiatry. 2017. PMID: 28348379 Free PMC article. Review.
-
Common schizophrenia alleles are enriched in mutation-intolerant genes and in regions under strong background selection.Nat Genet. 2018 Mar;50(3):381-389. doi: 10.1038/s41588-018-0059-2. Epub 2018 Feb 26. Nat Genet. 2018. PMID: 29483656 Free PMC article.
-
The polygenic architecture of schizophrenia - rethinking pathogenesis and nosology.Nat Rev Neurol. 2020 Jul;16(7):366-379. doi: 10.1038/s41582-020-0364-0. Epub 2020 Jun 11. Nat Rev Neurol. 2020. PMID: 32528109 Review.
Cited by
-
Causal effect between breast cancer and ovarian cancer: a two-sample mendelian randomization study.BMC Cancer. 2024 Nov 21;24(1):1433. doi: 10.1186/s12885-024-13033-8. BMC Cancer. 2024. PMID: 39573997 Free PMC article.
-
Genetic variants associated with longevity in long-living Indians.NPJ Aging. 2024 Nov 20;10(1):51. doi: 10.1038/s41514-024-00179-9. NPJ Aging. 2024. PMID: 39567526 Free PMC article.
-
Causality between autoimmune diseases and schizophrenia: a bidirectional Mendelian randomization study.BMC Psychiatry. 2024 Nov 16;24(1):817. doi: 10.1186/s12888-024-06287-w. BMC Psychiatry. 2024. PMID: 39550571 Free PMC article.
-
Meta-analyses of epigenetic age acceleration and GrimAge components of schizophrenia or first-episode psychosis.Schizophrenia (Heidelb). 2024 Nov 15;10(1):108. doi: 10.1038/s41537-024-00531-8. Schizophrenia (Heidelb). 2024. PMID: 39548083 Free PMC article.
-
Deciphering the tissue-specific functional effect of Alzheimer risk SNPs with deep genome annotation.BioData Min. 2024 Nov 13;17(1):50. doi: 10.1186/s13040-024-00400-1. BioData Min. 2024. PMID: 39538253 Free PMC article.
References
-
- Saha S, Chant D, McGrath J. A systematic review of mortality in schizophrenia: is the differential mortality gap worsening over time? Archives of General Psychiatry. 2007;64:1123–31. - PubMed
-
- World Health Organization . The Global Burden of Disease: 2004 Update. WHO Press; Geneva: 2008.
-
- Knapp M, Mangalore R, Simon J. The global costs of schizophrenia. Schizophrenia Bulletin. 2004;30:279–93. - PubMed
-
- Lieberman JA, et al. Effectiveness of antipsychotic drugs in patients with chronic schizophrenia. N Engl J Med. 2005;353:1209–23. - PubMed
-
- Carlsson A, Lindqvist M. Effect of Chlorpromazine or Haloperidol on Formation of 3methoxytyramine and Normetanephrine in Mouse Brain. Acta Pharmacologica et Toxicologica. 1963;20:140–4. - PubMed
Publication types
MeSH terms
Substances
Grants and funding
- R01 MH040799/MH/NIMH NIH HHS/United States
- K99 MH101367/MH/NIMH NIH HHS/United States
- G0601635/MRC_/Medical Research Council/United Kingdom
- R01 MH077139/MH/NIMH NIH HHS/United States
- G0800509/MRC_/Medical Research Council/United Kingdom
- G1000718/MRC_/Medical Research Council/United Kingdom
- G1100583/MRC_/Medical Research Council/United Kingdom
- WT_/Wellcome Trust/United Kingdom
- U01 MH094432/MH/NIMH NIH HHS/United States
- U01 MH096296/MH/NIMH NIH HHS/United States
- SCD/12/CSO_/Chief Scientist Office/United Kingdom
- I01 BX001356/BX/BLRD VA/United States
- MR/L010305/1/MRC_/Medical Research Council/United Kingdom
- T32 MH020030/MH/NIMH NIH HHS/United States
- PDA/02/06/016/DH_/Department of Health/United Kingdom
- G0801418/MRC_/Medical Research Council/United Kingdom
- I01 BX002395/BX/BLRD VA/United States
- R01 MH085548/MH/NIMH NIH HHS/United States
- G0901310/MRC_/Medical Research Council/United Kingdom
- U01 MH094421/MH/NIMH NIH HHS/United States
- R01 MH100141/MH/NIMH NIH HHS/United States
LinkOut - more resources
Full Text Sources
Other Literature Sources
Medical