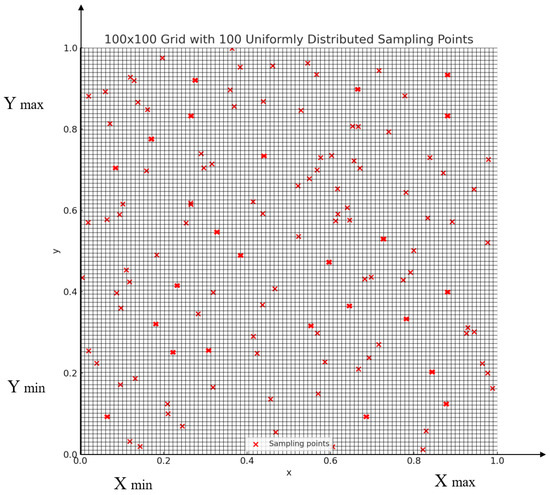
Figure 3
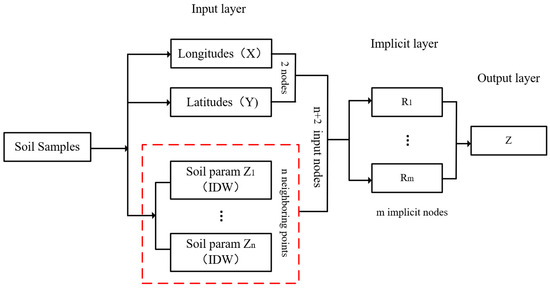
Figure 4
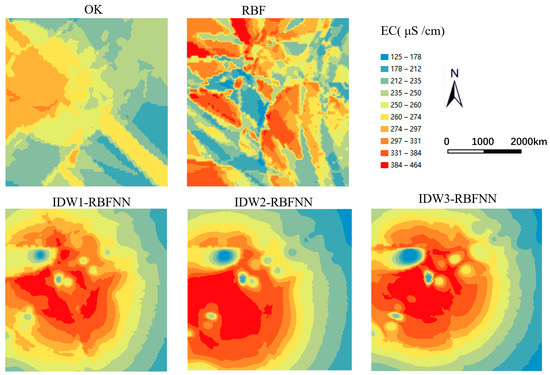
Figure 5
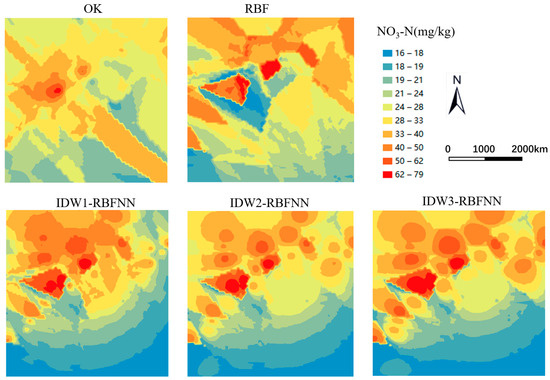
Figure 6
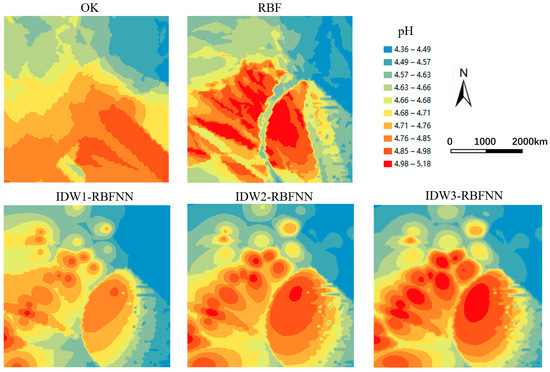
Figure 7
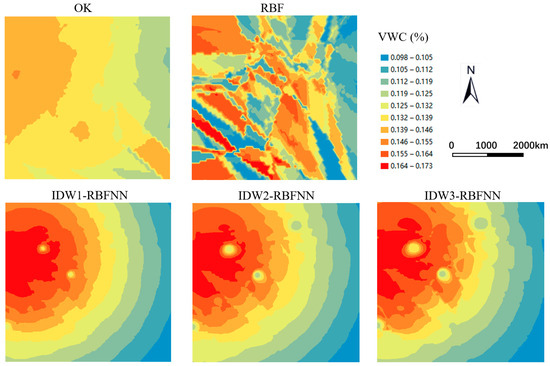
Figure 8
A special issue of Agronomy (ISSN 2073-4395). This special issue belongs to the section "Precision and Digital Agriculture".
Deadline for manuscript submissions: 31 March 2025 | Viewed by 10118
Dear Colleagues,
Responding to an era marked by the relentless pursuit of innovation and sustainability in farming practices, this collection of articles delves into the transformative potential of artificial intelligence, specifically machine learning and deep learning techniques, in revolutionizing the agricultural landscape.
In today's world, where the global population continues to rise and climate change presents increasingly complex challenges, agriculture stands at a crossroads. It must meet the growing demand for food while mitigating its environmental footprint. The emergence of smart farming and smart agriculture, driven by machine learning, holds immense promise in achieving this delicate balance.
Machine learning, with its ability to process vast datasets and uncover hidden patterns, enables us to make sense of the intricate web of factors that affect agricultural production. Whether predicting crop yields with unprecedented accuracy, identifying and managing pest infestations, optimizing resource allocation, or enhancing the breeding of resilient crops, machine learning empowers us to make informed decisions that drive efficiency, sustainability, and profitability in agriculture.
We are particularly excited about the diverse array of topics covered in this Special Issue. We welcome contributions that encompass smart farming, precision agriculture, and data-driven solutions across the agricultural spectrum. Our contributors include esteemed researchers and practitioners from around the globe, each offering valuable insights into the dynamic field of advanced machine learning in agriculture.
Dr. Paul Kwan
Dr. Jing Zhou
Dr. Beibei Xu
Guest Editors
Manuscript Submission Information
Manuscripts should be submitted online at www.mdpi.com by registering and logging in to this website. Once you are registered, click here to go to the submission form. Manuscripts can be submitted until the deadline. All submissions that pass pre-check are peer-reviewed. Accepted papers will be published continuously in the journal (as soon as accepted) and will be listed together on the special issue website. Research articles, review articles as well as short communications are invited. For planned papers, a title and short abstract (about 100 words) can be sent to the Editorial Office for announcement on this website.
Submitted manuscripts should not have been published previously, nor be under consideration for publication elsewhere (except conference proceedings papers). All manuscripts are thoroughly refereed through a single-blind peer-review process. A guide for authors and other relevant information for submission of manuscripts is available on the Instructions for Authors page. Agronomy is an international peer-reviewed open access monthly journal published by MDPI.
Please visit the Instructions for Authors page before submitting a manuscript. The Article Processing Charge (APC) for publication in this open access journal is 2600 CHF (Swiss Francs). Submitted papers should be well formatted and use good English. Authors may use MDPI's English editing service prior to publication or during author revisions.
Further information on MDPI's Special Issue polices can be found here.
Figure 3
Figure 4
Figure 5
Figure 6
Figure 7
Figure 8
Figure 3
Figure 4
Figure 5
Figure 6
Figure 7
Figure 8
Figure 9
Figure 10
Figure 11
Figure 12
Figure 13
Figure 3
Figure 4
Figure 5
Figure 6
Figure 7
Figure 8
Figure 9
Figure 10
Figure 11
Figure 12
Figure 13
Figure 14
Figure 15
Figure 3
Figure 4
Figure 5
Figure 6
Figure 7
Figure 8
Figure 9
Figure 10
Figure 11
Figure 12
Figure 3
Figure 4
Figure 5
Figure 6
Figure 7
Figure 8
Figure 9
Figure 10
Figure 11
Figure 12
Figure 13
Figure 14
Figure 15
Figure 16
Figure 17
Figure 3
Figure 4
Figure 5
Figure 6
Figure 7
Figure 8
Figure 9
Figure 10
Figure 11
Figure 12
Figure 13
Figure 14
Figure 15
Figure 16
Figure 3
Figure 4
Figure 5
Figure 6
Figure 7
Figure 8
Jump to: Research
Figure 3
Figure 4
Figure 5
Figure 6
Figure 7
Figure 8
Figure 9
Figure 10
Figure 11