Quantifying microbial guilds
- PMID: 38707845
- PMCID: PMC11069341
- DOI: 10.1093/ismeco/ycae042
Quantifying microbial guilds
Abstract
The ecological role of microorganisms is of utmost importance due to their multiple interactions with the environment. However, assessing the contribution of individual taxonomic groups has proven difficult despite the availability of high throughput data, hindering our understanding of such complex systems. Here, we propose a quantitative definition of guild that is readily applicable to metagenomic data. Our framework focuses on the functional character of protein sequences, as well as their diversifying nature. First, we discriminate functional sequences from the whole sequence space corresponding to a gene annotation to then quantify their contribution to the guild composition across environments. In addition, we identify and distinguish functional implementations, which are sequence spaces that have different ways of carrying out the function. In contrast, we found that orthology delineation did not consistently align with ecologically (or functionally) distinct implementations of the function. We demonstrate the value of our approach with two case studies: the ammonia oxidation and polyamine uptake guilds from the Malaspina circumnavigation cruise, revealing novel ecological dynamics of the latter in marine ecosystems. Thus, the quantification of guilds helps us to assess the functional role of different taxonomic groups with profound implications on the study of microbial communities.
Keywords: ammonia oxidation; functional ecology; microbial ecology; microbial guilds; phylogenetics; polyamine uptake.
© The Author(s) 2024. Published by Oxford University Press on behalf of the International Society for Microbial Ecology.
Conflict of interest statement
The authors declare no competing interests.
Figures
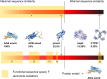
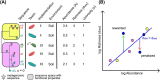
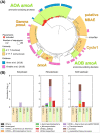
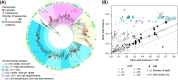
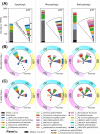
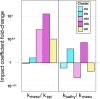
Similar articles
-
"It Is a Big Spider Web of Things": Sensory Experiences of Autistic Adults in Public Spaces.Autism Adulthood. 2023 Dec 1;5(4):411-422. doi: 10.1089/aut.2022.0024. Epub 2023 Dec 12. Autism Adulthood. 2023. PMID: 38116051 Free PMC article.
-
Qualitative evidence synthesis informing our understanding of people's perceptions and experiences of targeted digital communication.Cochrane Database Syst Rev. 2019 Oct 23;10(10):ED000141. doi: 10.1002/14651858.ED000141. Cochrane Database Syst Rev. 2019. PMID: 31643081 Free PMC article.
-
Using Experience Sampling Methodology to Capture Disclosure Opportunities for Autistic Adults.Autism Adulthood. 2023 Dec 1;5(4):389-400. doi: 10.1089/aut.2022.0090. Epub 2023 Dec 12. Autism Adulthood. 2023. PMID: 38116059 Free PMC article.
-
Community views on active case finding for tuberculosis in low- and middle-income countries: a qualitative evidence synthesis.Cochrane Database Syst Rev. 2024 Mar 21;3(3):CD014756. doi: 10.1002/14651858.CD014756.pub2. Cochrane Database Syst Rev. 2024. PMID: 38511668 Free PMC article. Review.
-
Interventions to reduce harm from continued tobacco use.Cochrane Database Syst Rev. 2016 Oct 13;10(10):CD005231. doi: 10.1002/14651858.CD005231.pub3. Cochrane Database Syst Rev. 2016. PMID: 27734465 Free PMC article. Review.
References
-
-
Ladero M, Ruiz G, Pessela BCC et al. Thermal and ph inactivation of an immobilized thermostable
-galactosidase from thermus sp. strain t2: comparison to the free enzyme. Biochem Eng J 2006;31:14–24. 10.1016/j.bej.2006.05.012 - DOI
-
Ladero M, Ruiz G, Pessela BCC et al. Thermal and ph inactivation of an immobilized thermostable
LinkOut - more resources
Full Text Sources