Sequencing and beyond: integrating molecular 'omics' for microbial community profiling
- PMID: 25915636
- PMCID: PMC4800835
- DOI: 10.1038/nrmicro3451
Sequencing and beyond: integrating molecular 'omics' for microbial community profiling
Abstract
High-throughput DNA sequencing has proven invaluable for investigating diverse environmental and host-associated microbial communities. In this Review, we discuss emerging strategies for microbial community analysis that complement and expand traditional metagenomic profiling. These include novel DNA sequencing strategies for identifying strain-level microbial variation and community temporal dynamics; measuring multiple 'omic' data types that better capture community functional activity, such as transcriptomics, proteomics and metabolomics; and combining multiple forms of omic data in an integrated framework. We highlight studies in which the 'multi-omics' approach has led to improved mechanistic models of microbial community structure and function.
Figures
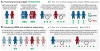
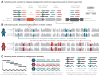
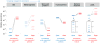
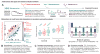
Similar articles
-
Omics approaches to study host-microbiota interactions.Curr Opin Microbiol. 2013 Jun;16(3):270-7. doi: 10.1016/j.mib.2013.07.001. Epub 2013 Jul 23. Curr Opin Microbiol. 2013. PMID: 23891019 Review.
-
Foodomics as part of the host-microbiota-exposome interplay.J Proteomics. 2016 Sep 16;147:3-20. doi: 10.1016/j.jprot.2016.04.033. Epub 2016 Apr 26. J Proteomics. 2016. PMID: 27130534 Review.
-
Integration of 'omics' data: does it lead to new insights into host-microbe interactions?Future Microbiol. 2010 Feb;5(2):313-28. doi: 10.2217/fmb.10.1. Future Microbiol. 2010. PMID: 20143952 Review.
-
MetaComp: comprehensive analysis software for comparative meta-omics including comparative metagenomics.BMC Bioinformatics. 2017 Oct 2;18(1):434. doi: 10.1186/s12859-017-1849-8. BMC Bioinformatics. 2017. PMID: 28969605 Free PMC article.
-
Integrated omics for the identification of key functionalities in biological wastewater treatment microbial communities.Microb Biotechnol. 2015 May;8(3):363-8. doi: 10.1111/1751-7915.12255. Epub 2015 Feb 12. Microb Biotechnol. 2015. PMID: 25678254 Free PMC article.
Cited by
-
Gut microbiota, metabolites and host immunity.Nat Rev Immunol. 2016 May 27;16(6):341-52. doi: 10.1038/nri.2016.42. Nat Rev Immunol. 2016. PMID: 27231050 Free PMC article. Review.
-
De Novo Genome Sequencing, Annotation, and Taxonomy of Unknown Bacteria.Cold Spring Harb Protoc. 2023 Jan 3;2023(1):1-3. doi: 10.1101/pdb.top107847. Cold Spring Harb Protoc. 2023. PMID: 36283838 Free PMC article.
-
Invisible Inhabitants of Plants and a Sustainable Planet: Diversity of Bacterial Endophytes and their Potential in Sustainable Agriculture.Indian J Microbiol. 2024 Jun;64(2):343-366. doi: 10.1007/s12088-024-01225-6. Epub 2024 Mar 5. Indian J Microbiol. 2024. PMID: 39011025 Review.
-
Activity-based metaproteomics driven discovery and enzymological characterization of potential α-galactosidases in the mouse gut microbiome.Commun Chem. 2024 Aug 16;7(1):184. doi: 10.1038/s42004-024-01273-5. Commun Chem. 2024. PMID: 39152233 Free PMC article.
-
Gut microbiome lipid metabolism and its impact on host physiology.Cell Host Microbe. 2023 Feb 8;31(2):173-186. doi: 10.1016/j.chom.2023.01.009. Cell Host Microbe. 2023. PMID: 36758518 Free PMC article. Review.
References
-
- Handelsman J, Rondon MR, Brady SF, Clardy J, Goodman RM. Molecular biological access to the chemistry of unknown soil microbes: a new frontier for natural products. Chem Biol. 1998;5:R245–9. - PubMed
-
- Tyson GW, et al. Community structure and metabolism through reconstruction of microbial genomes from the environment. Nature. 2004;428:37–43. - PubMed
-
- Venter JC, et al. Environmental genome shotgun sequencing of the Sargasso Sea. Science. 2004;304:66–74. One of the first large-scale environmental metagenomic sequencing projects; presented profiles of taxonomic composition and function from geographically diverse marine microbial communities. - PubMed
Publication types
MeSH terms
Grants and funding
LinkOut - more resources
Full Text Sources
Other Literature Sources