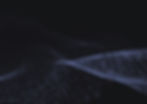
Bayesian Scientific Machine Learning (SciML) from PK to Synthetic Controls and Digital Twins

Make better decisions by using disease-specific models
How predictive is my new biomarker? What attributes differentiate responders from non-responders? How fast will a patient progress on or off treatment? What is the probability that my new treatment is better than the competitor or standard of care? What is the best dose for a specific treatment or a specific person? These are some of the questions we are working on with leading Pharma and Biotech companies.

Interpretability
Our models are generative, transparent, explainable, and testable. In contrast to black box methods, we know what these models are doing.

Predictive Accuracy
In-sample predictions are easy. Our models make well calibrated predictions out of sample: for a new patient, a new study arm, and even a new trial.
.png)
Algorithms
Recent advances in computational statistics allow us to tackle models previously thought too difficult due to non-linearities and the number of unknowns.

Small Data and RWE
Our models work well in the small data regime, such as in platform trials in Oncology and Rare Diseases, where we need to take advantage of information external to the clinical trial.

Joint Outcome-Biomarker Models
Linking the model for the hazard with
sub-models for individual biomarkers.
Joint models for comorbidities.