How to build a gen AI application
Shantanu Pai
UX Director, Cloud AI
As enterprises race towards building and deploying generative AI applications, there are many open questions about how to design the end user experience of these applications to take advantage of this powerful technology, while avoiding its pitfalls. The Cloud AI and Industry Solutions team has been conducting extensive design and user research activities to understand how users perceive and engage with generative AI applications. Here we share our learnings in order to help enterprise customers build useful and delightful end user experiences for their generative AI applications.Â
Though generative AI applications have exploded in popularity in the last few months, very little is understood today about usersâ mental models, perceptions, preferences, and challenges while interacting with these applications. Our user experience (UX) team launched an organization-wide effort aimed at creating a collection of user-tested micro-interaction design patterns and research-backed guidance for using those design patterns in generative AI applications.Â
For this, we first launched a âdesign challengeâ within our UX team to solicit a variety of micro-interaction designs, guided by generative AI design principles. Next, we created prototypes of various chatbot applications that incorporated those designs. Finally, we collected qualitative feedback from 15 external users on these prototypes through various user research methods, like moderated and unmoderated user feedback sessions.Â
Below, we distill our takeaways in the form of design principles and design patterns to illustrate those principles.Â
Key takeaways
1. Help users explore generative variability
One of our key learnings was that we should help users explore generative variability, that is the ability of a generative AI application to produce a range of outputs for the same prompt or question. Additionally, we need to help users understand triggers and end points of an interaction with a generative AI model.Â
- Generative variability: Generative variability is a salient feature of generative AI apps. In our studies, we found that users liked micro-interactions that helped them take advantage of this âgenerative variabilityâ through the âGenerate Againâ button (Fig 1) that allowed them to regenerate results for their prompt . They also liked the ability to specify dimensions or preferences for re-generating results, e.g., providing options to re-generate a trip itinerary based on the price or popularity of the attractions (as in the drop-down in Fig 1).
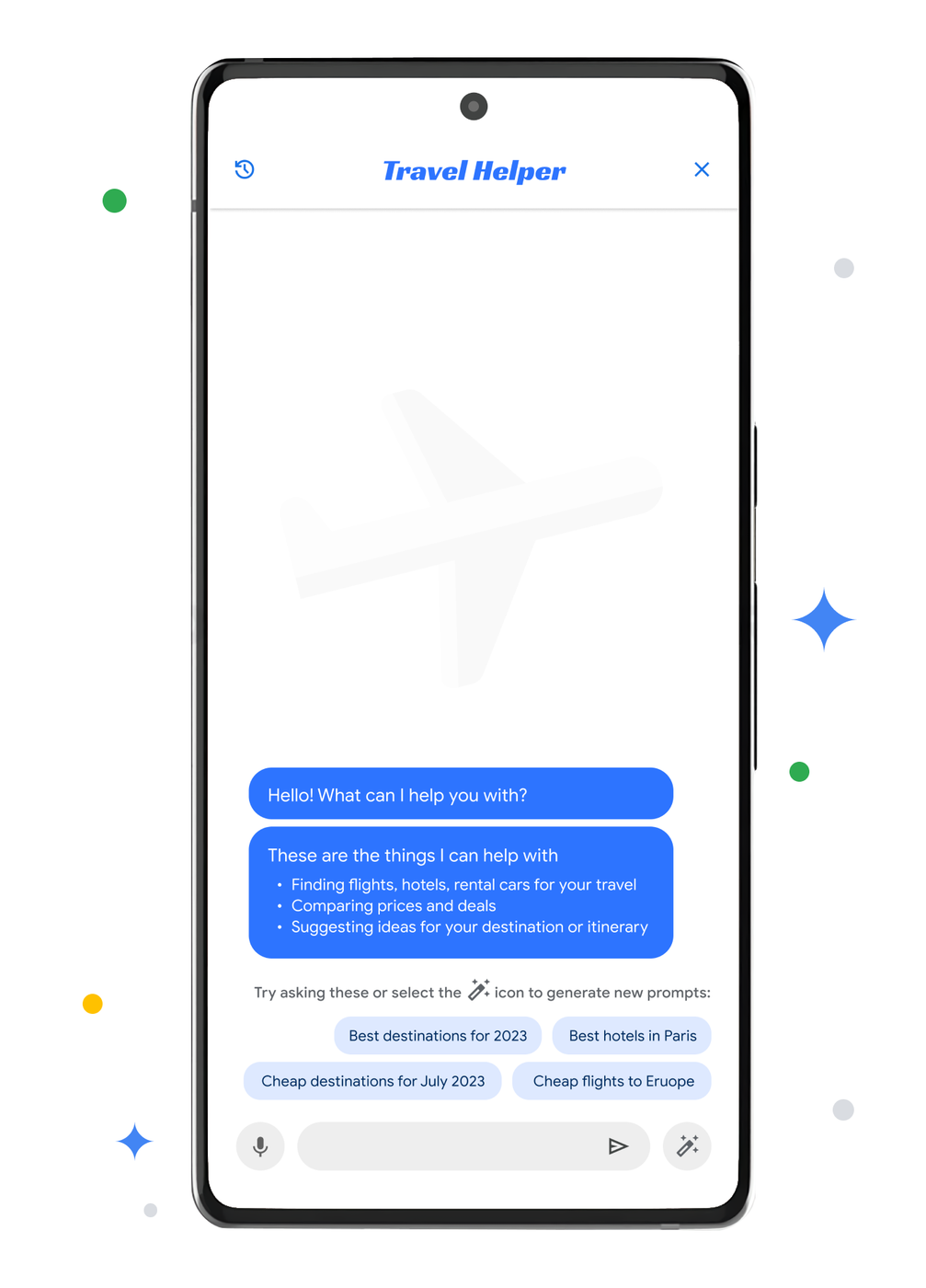
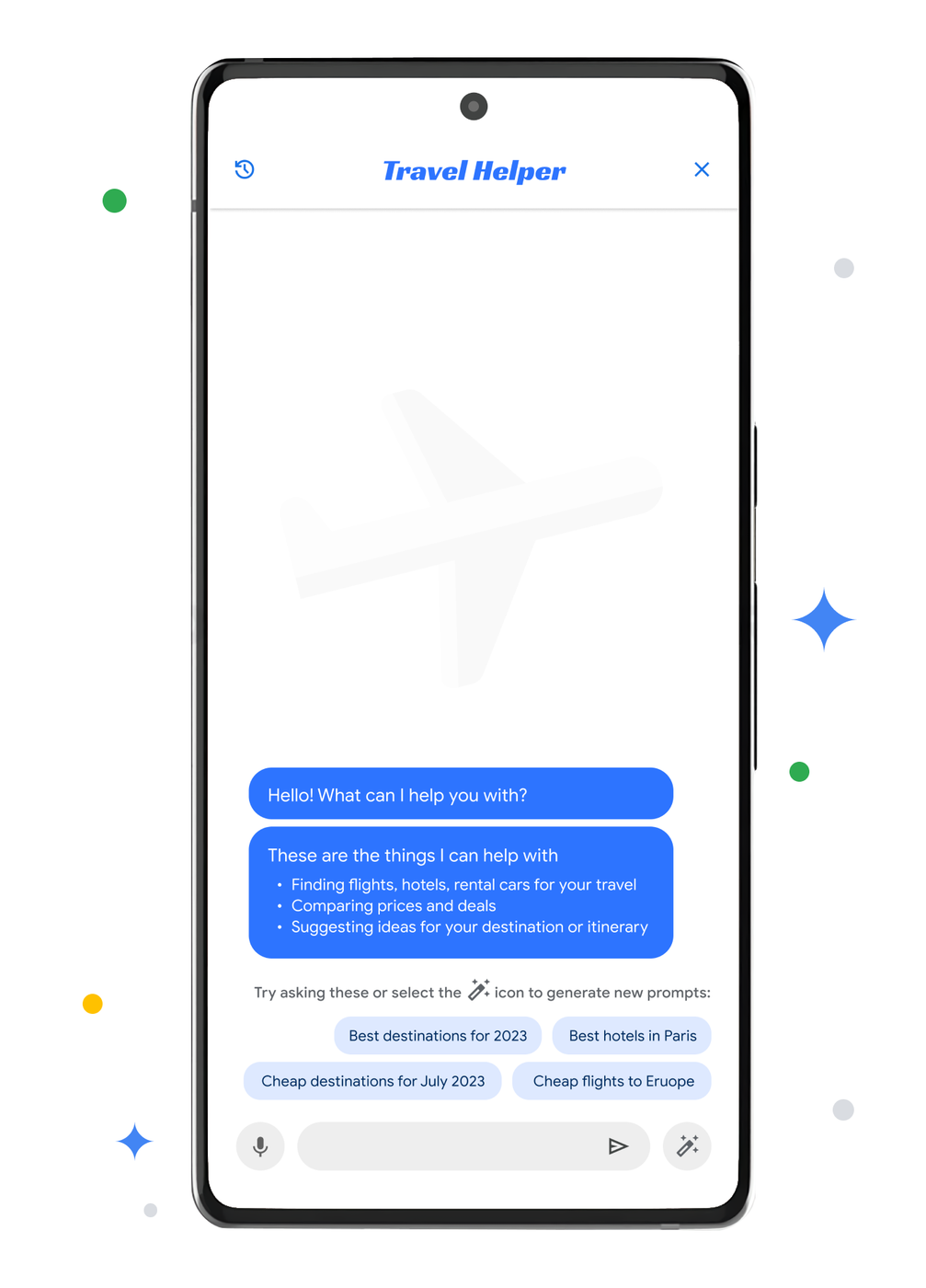
Fig (1)
- Triggers: Users wanted to understand and control how content was generated in the course of completing a task. For example, when we showed users a âGenerate Imageâ button within a chat conversation (Fig 3), they wanted to know when and with what frequency this button would be displayed. Further, they preferred the âgenerate imageâ option appearing when they explicitly showed intent to find an image (Fig 4) to it appearing automatically during a chat conversation without explicit intent indicated from the user (Fig 3).
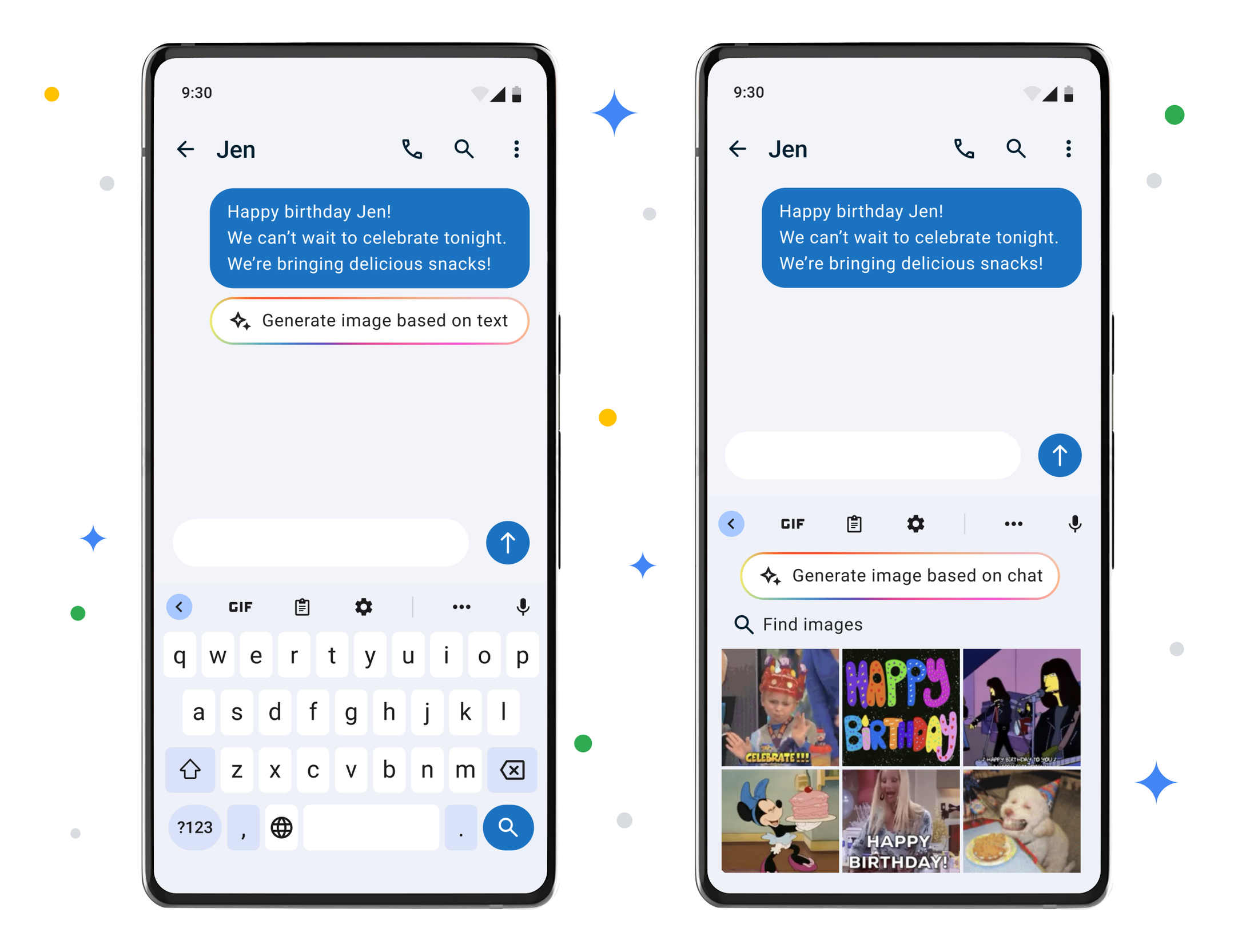
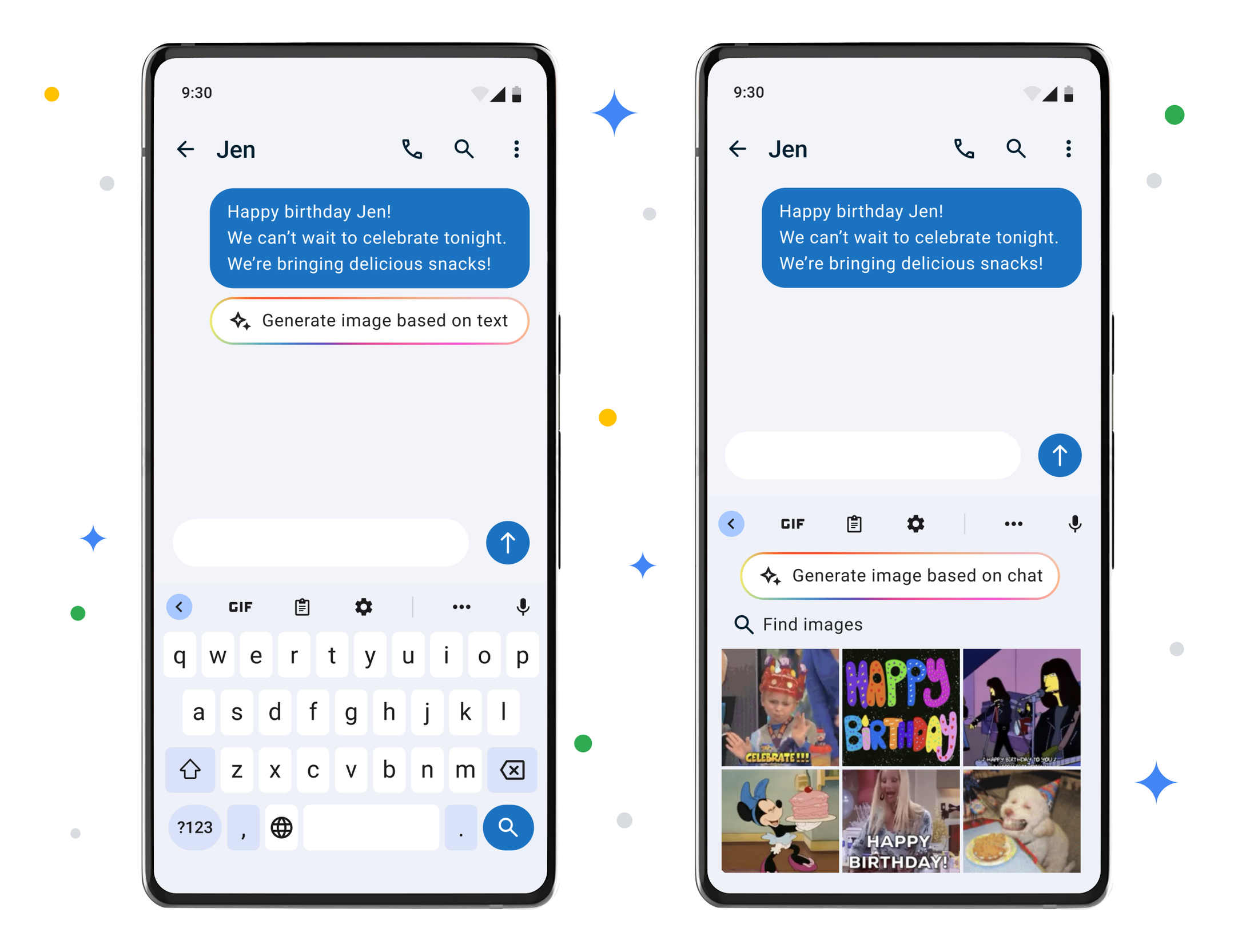
Fig (3), Fig (4)
-
End-points: While users liked being able to generate alternative results for their prompts, they needed help understanding when or how to stop regenerating results. To address this, we could provide notifications when results are becoming repetitive, and let them know that the system will notify them if new recommendations become available.
 2. Help users build trustÂ
It was important for users to feel like they can trust the generated responses. We found that citing the source of the content helps increase user trust. Users appreciated citations in various formats. For example, when a conversational bot provided hotel recommendations in a table format (Fig 5), users liked having links to each hotelâs website so they could visit the hotel page, check out photos, and confirm that the price was correct. When presented with a response summarized from many sources (Fig 6), they wanted links to the individual sources that were used in arriving at the summary.
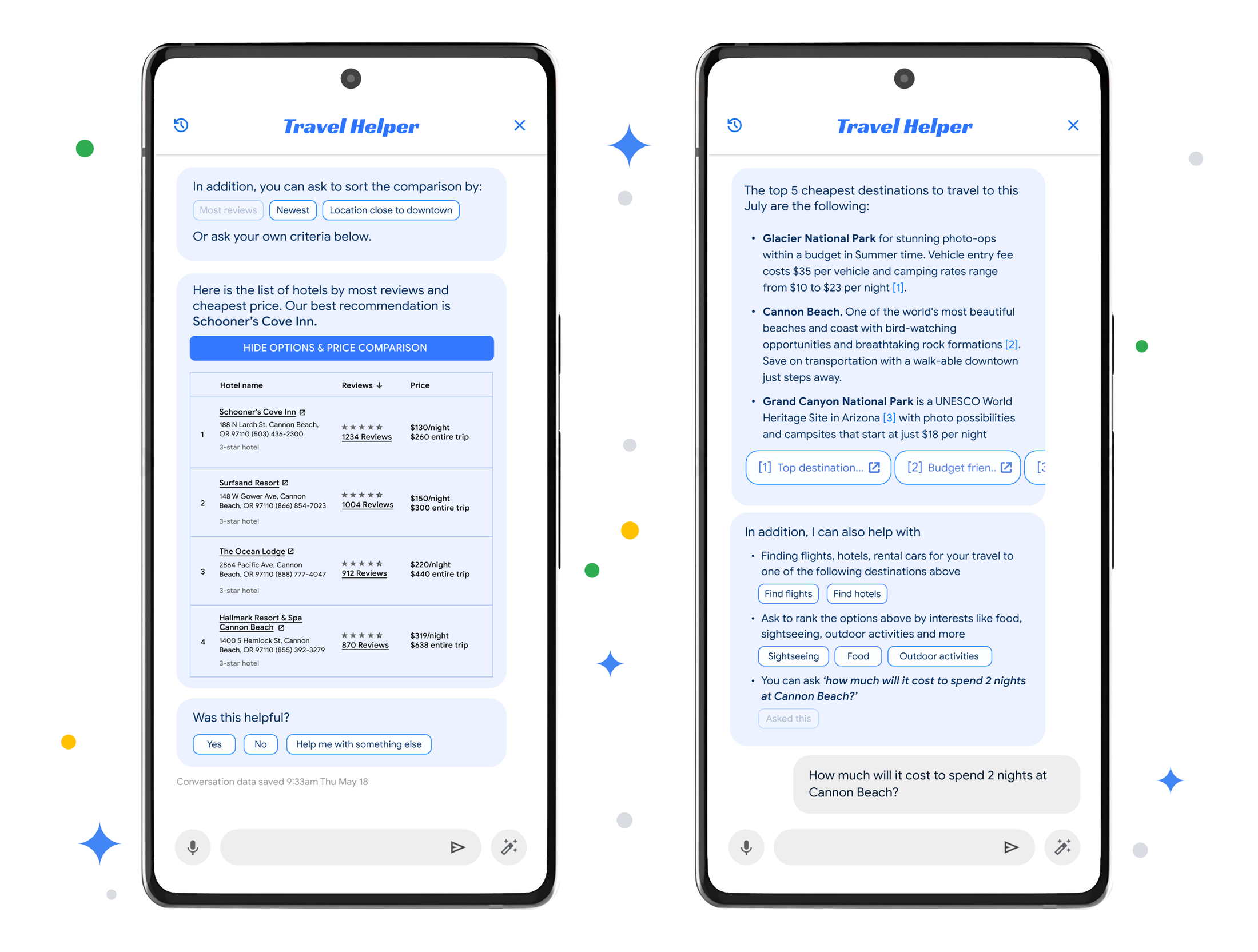
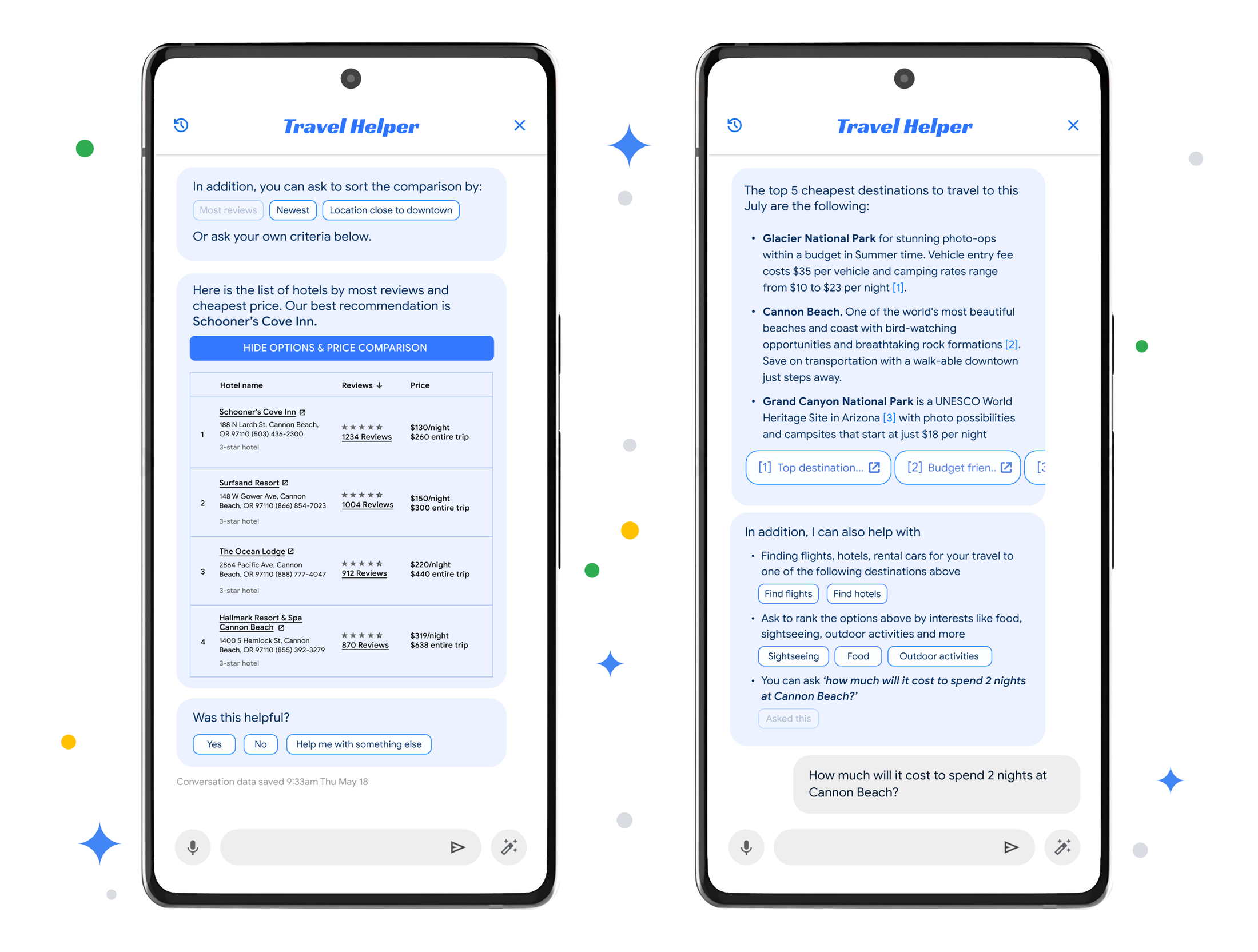
Fig (5), Fig (6)
3. Give users control over generated responses
Provide users control by letting them choose between multiple responses generated or by allowing them to modify the generated response based on their preferences. This helps ensure that users get the information that they need, in the format that they want. For example, in the chat application, users wanted to be able to edit the image that was generated based on the chat content to align it with their own preferences.
Another way of giving users control is to help them with prompt engineering. Users of generative AI applications may not understand how the quality of their prompt affects answers, and may be unaware of different prompting techniques that they can apply. In our studies, users liked âsuggested promptâ chips (Fig 7) as a way to explore better and/or related prompts. They also liked the ability to edit prompts through dropdowns that helped select alternative values for prompt parameters (Fig 8).
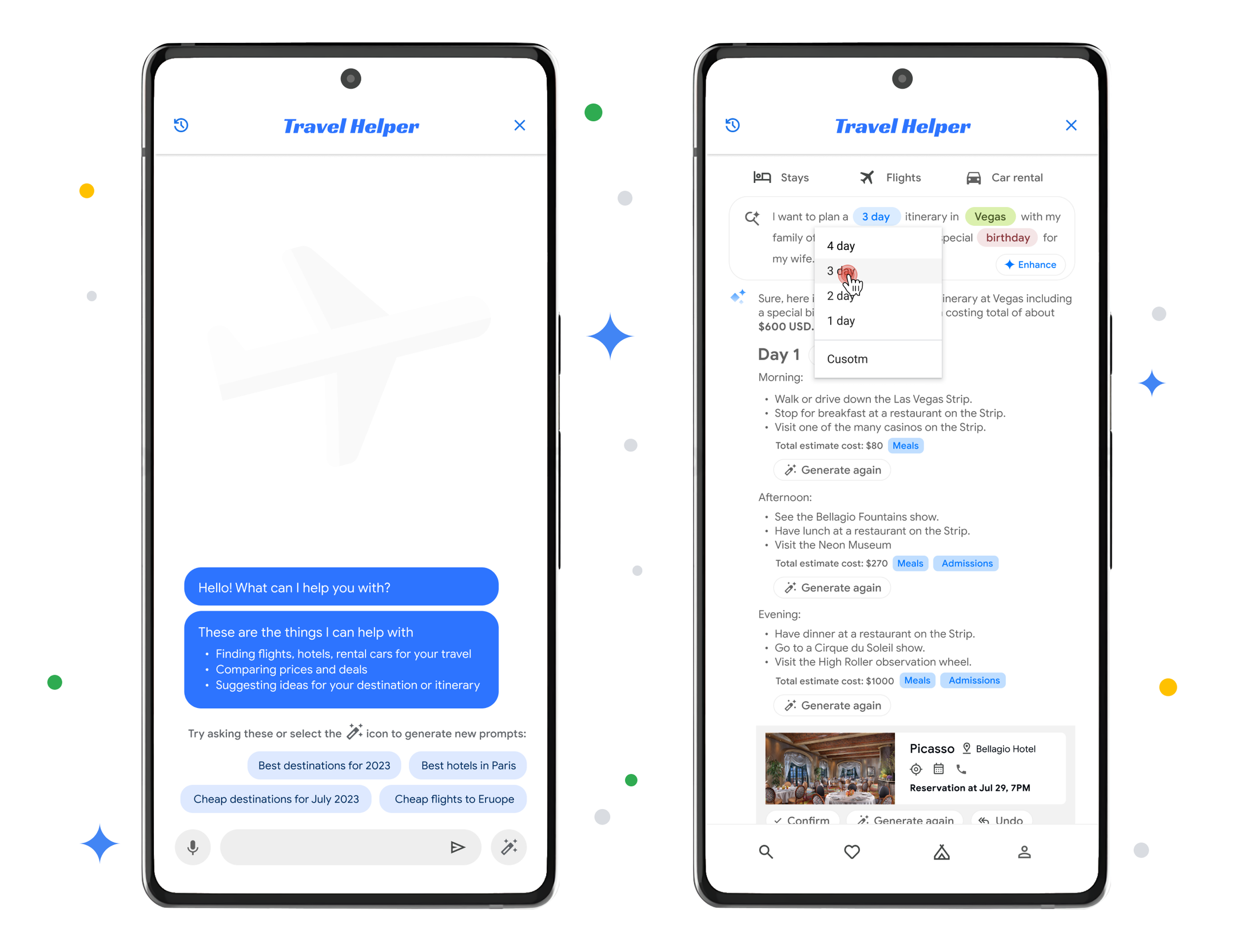
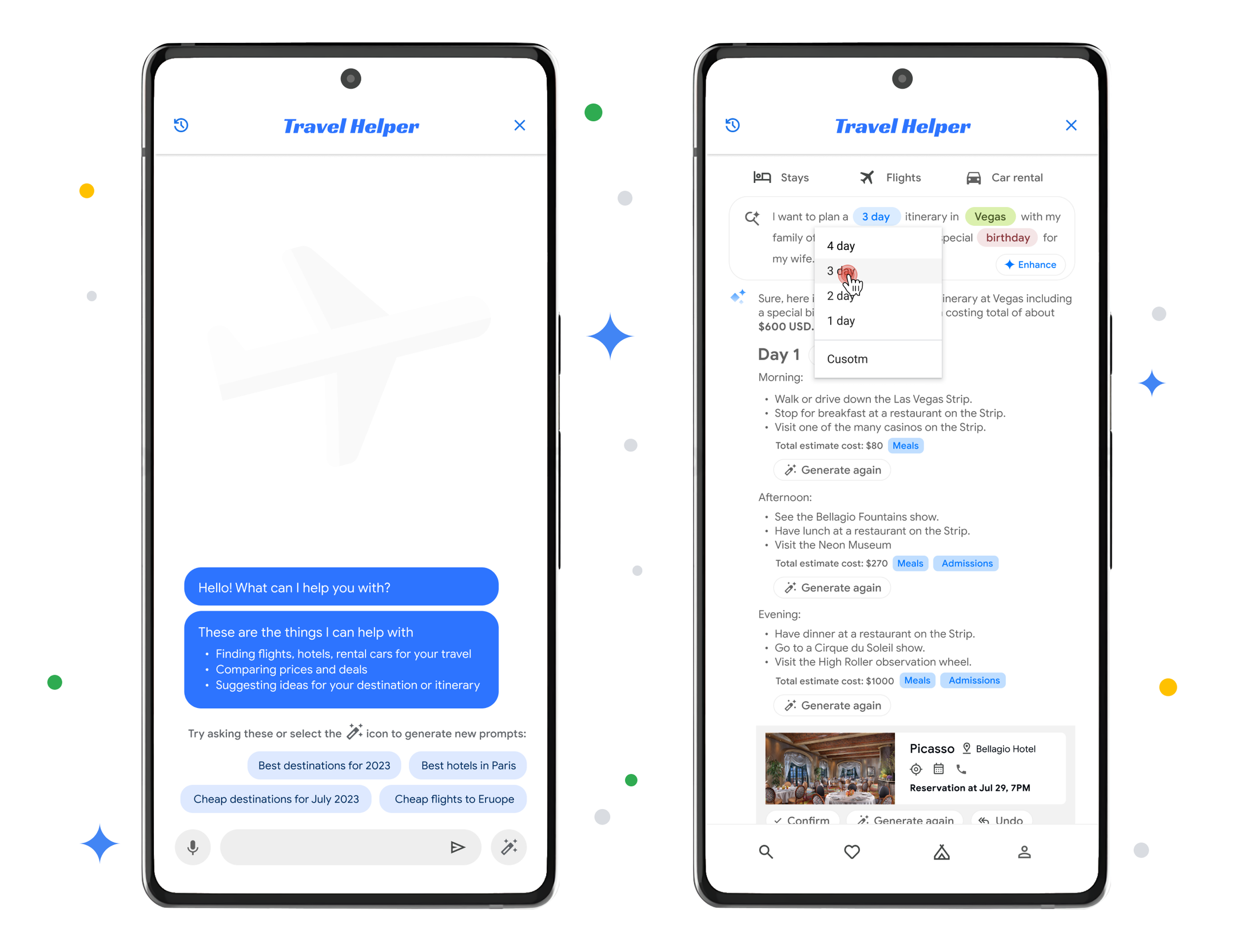
Fig (7), Fig (8)
4. Improve results through user feedback
User feedback can be used to improve the quality of the generated information, and to make sure that it is aligned with the needs of the users. We found that users cared about the placement and timing of the feedback collection. For example, when users saw the feedback request below (Fig 9) during a conversation with a travel chatbot, some thought that the chatbot was done providing information and was ready to move on to another topic. This was perhaps because the feedback collection was disjointed visually from the botâs response. We should design feedback collection to be âin-the-momentâ by placing the feedback request next to the response we need feedback on (Fig 10). Additionally, we could collect reasons from the user to explain their rating, providing insight into how to make the generated responses better.
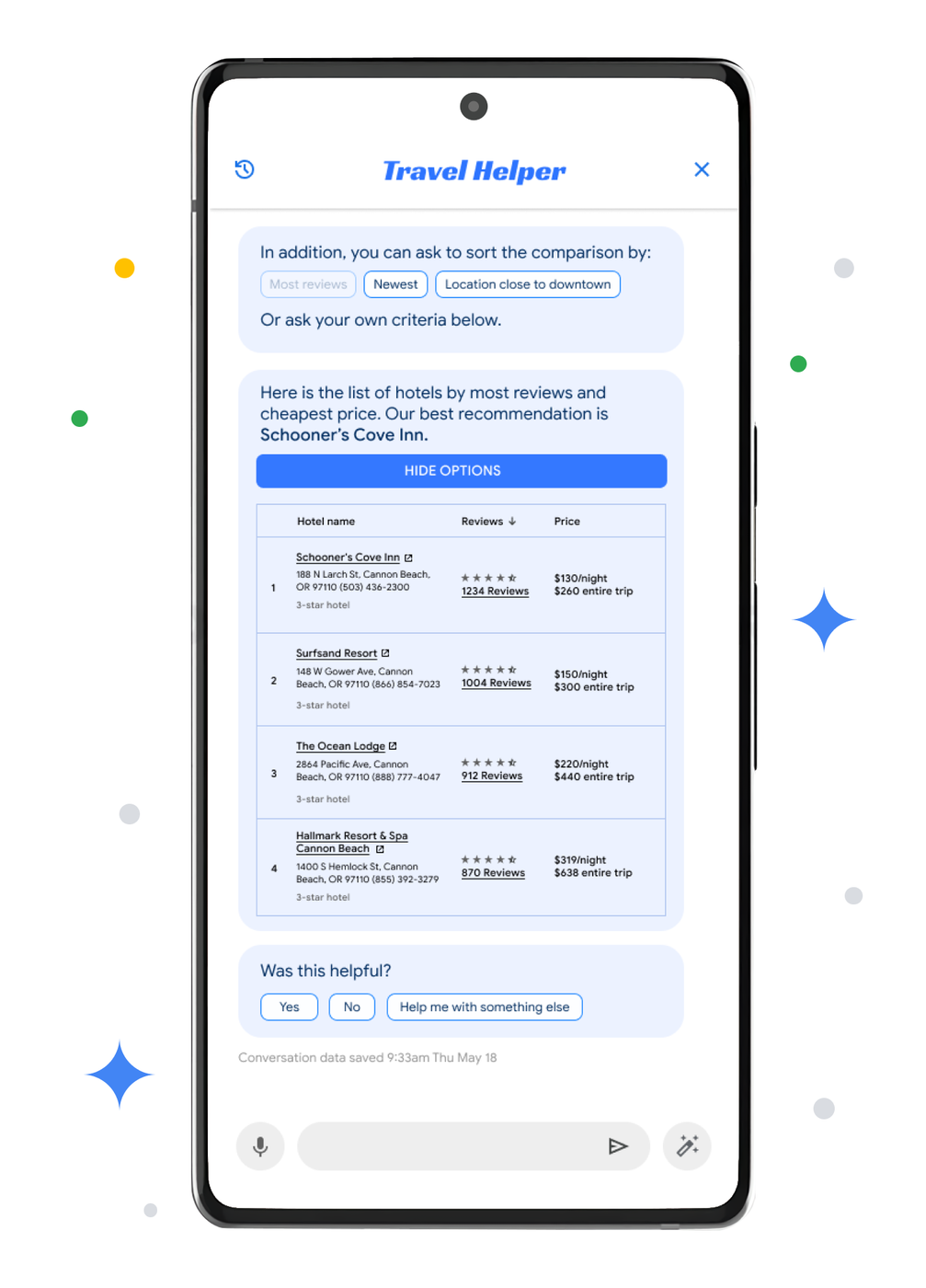
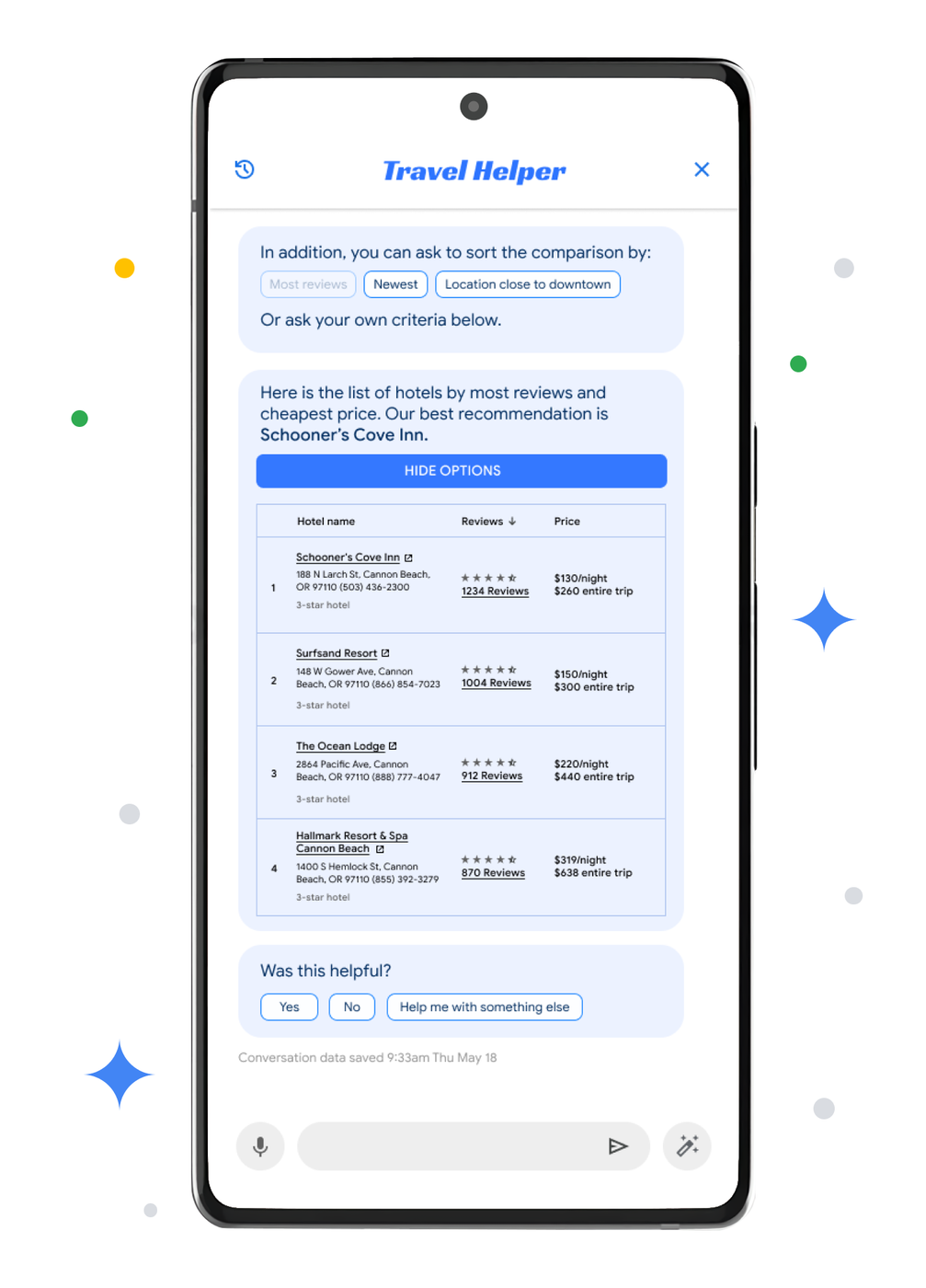
Fig (9)
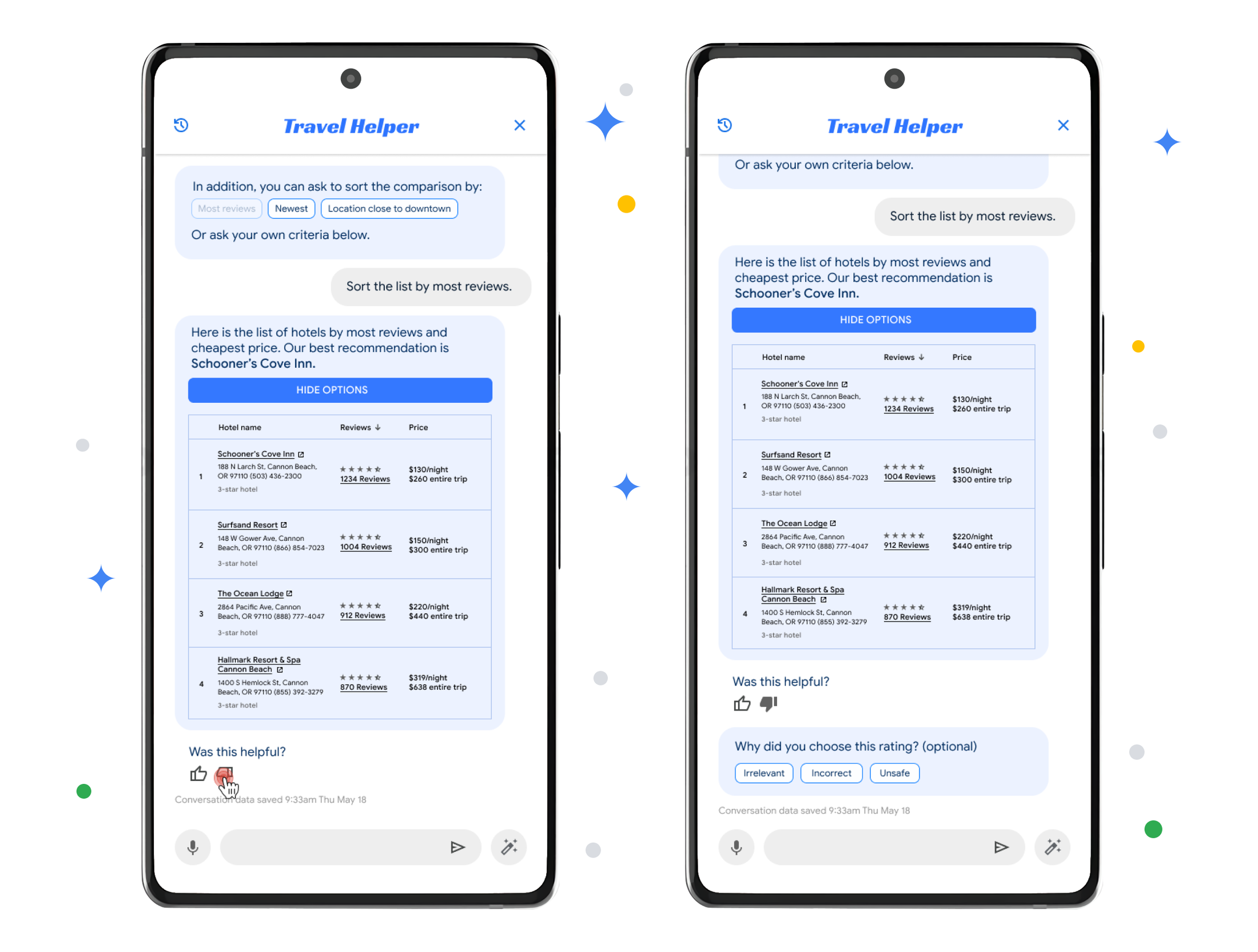
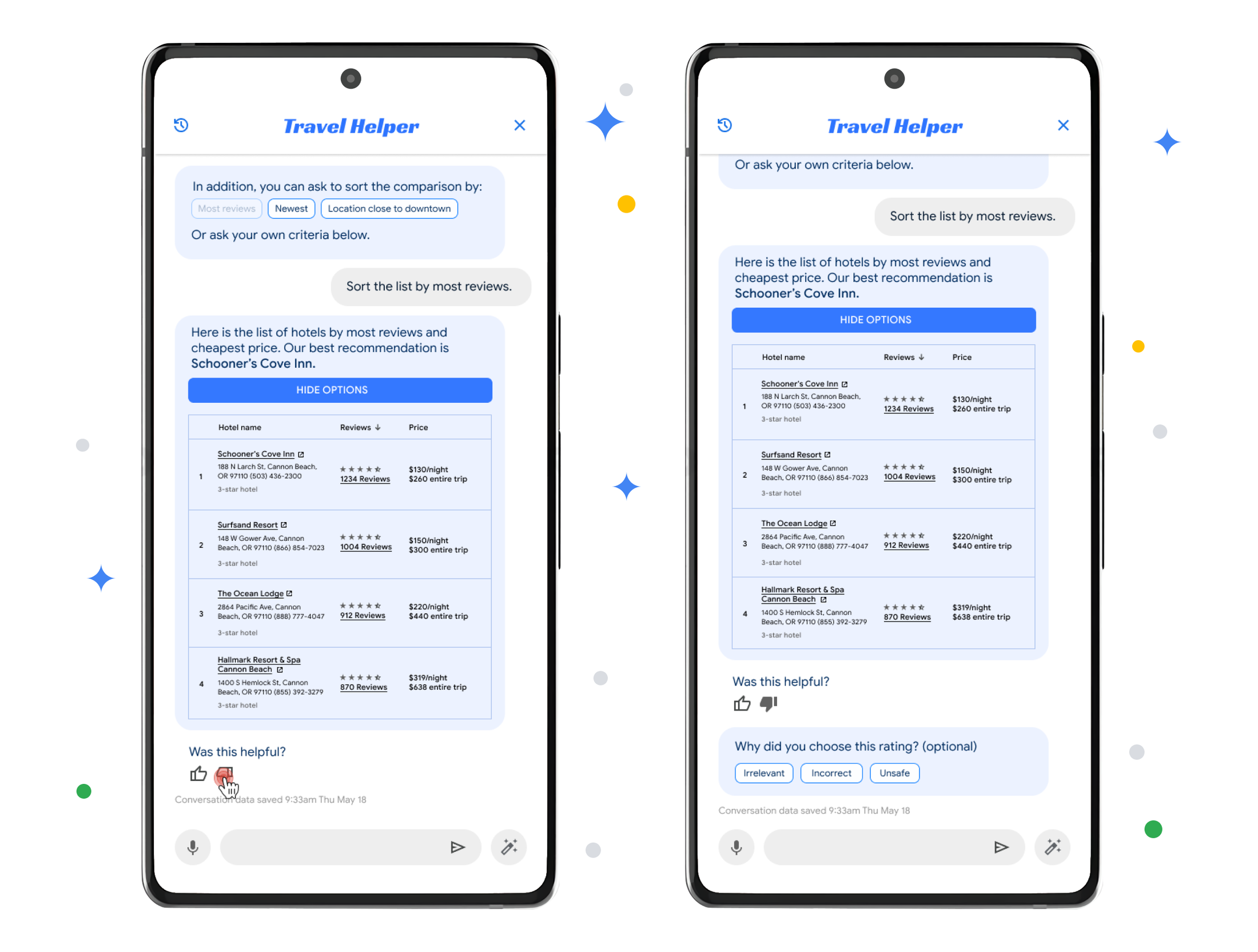
Fig (10)
Conclusion
During this iterative research and design process, we also learned best practices for conducting user research on interfaces that provide generative outputs. For example, user studies with a user research expert guiding the exploration worked much better than studies where we left users alone with the prototypes. But most critically we learned that users care about transparency, and want to know more about what's happening under the hood to see if they can trust the generated outputs. Providing users a sense of control and ways to give feedback also goes a long way to driving trust in the generated outputs.