Our ongoing work to build and deploy responsible AI
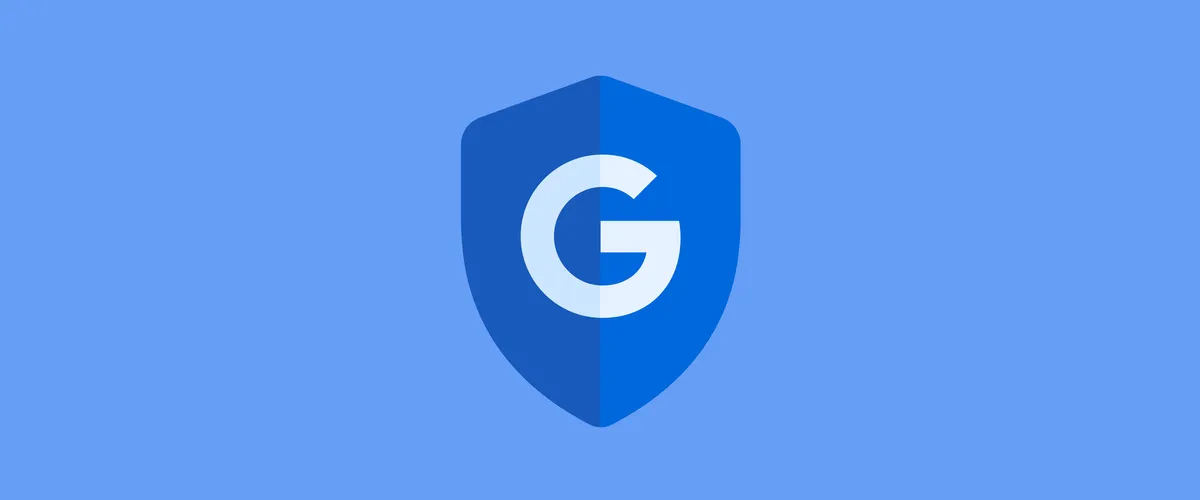
Editor’s note: This week, at the Google Responsible AI Summit in Paris, our VP of Trust & Safety Laurie Richardson delivered a keynote address to an audience of experts across academia, industry, startups, government and civil society. The following excerpt has been edited for brevity.
AI has the potential to solve big challenges, from saving lives by predicting when and where floods may occur, to transforming our understanding of the biological world and drug discovery. However, in order to realize these opportunities, it is critically important that we build and maintain trust in AI’s potential.
That’s why, as people begin to use AI in their daily lives, we are building technology in ways that seek to maximize benefits and minimize risks.
Our AI Responsibility Lifecycle
Our Trust & Safety teams are pioneering testing, training and red-teaming techniques to ensure that when our GenAI products go to market, they are both bold and responsible. Every day, we learn more about how to test for safety, neutrality, fairness and dangerous capabilities, and we’re committed to sharing our approach more broadly.
This year we launched our AI Responsibility Lifecycle framework to the public. This is a four-phase process — covering Research, Design, Governance and Sharing — that guides responsible AI development end-to-end at Google.

Detecting abuse at scale
Our teams across Trust & Safety are also using AI to improve the way we protect our users online. AI is showing tremendous promise for speed and scale in nuanced abuse detection. Building on our established automated processes, we have developed prototypes that leverage recent advances, to assist our teams in identifying abusive content at scale.
Using LLMs, our aim is to be able to rapidly build and train a model in a matter of days — instead of weeks or months — to find specific kinds of abuse on our products. This is especially valuable for new and emerging abuse areas, such as Russian disinformation narratives following the invasion of Ukraine, or for nuanced scaled challenges, like detecting counterfeit goods online. We can quickly prototype a model and automatically route it to our teams for enforcement.
LLMs are also transforming training. Using new techniques, we can now expand coverage of abuse types, context and languages in ways we never could have before — including doubling the number of languages covered with our on-device safety classifiers in the last quarter alone. Starting with an insight from one of our abuse analysts, we can use LLMs to generate thousands of variations of an event and then use this to train our classifiers.
We're still testing these new techniques to meet rigorous accuracy standards, but prototypes have demonstrated impressive results so far. The potential is huge, and I believe we are at the cusp of dramatic transformation in this space.
Boosting collaboration and transparency
Addressing AI-generated content will require industry and ecosystem collaboration and solutions; no one company or institution can do this work alone. Earlier this week at the summit, we brought together researchers and students to engage with our safety experts to discuss risks and opportunities in the age of AI. In support of an ecosystem that generates impactful research with real-world applications, we doubled the number of Google Academic Research Awards recipients this year to grow our investment into Trust & Safety research solutions.
Finally, information quality has always been core to Google’s mission, and part of that is making sure that users have context to assess the trustworthiness of content they find online. As we continue to bring AI to more products and services, we are focused on helping people better understand how a particular piece of content was created and modified over time.
Earlier this year, we joined the Coalition for Content Provenance and Authenticity (C2PA), as a steering committee member. We are partnering with others to develop interoperable provenance standards and technology to help explain whether a photo was taken with a camera, edited by software or produced by generative AI. This kind of information helps our users make more informed decisions about the content they’re engaging with — including photos, videos and audio — and builds media literacy and trust.
Our work with the C2PA directly complements our own broader approach to transparency and the responsible development of AI. For example, we’re continuing to bring our SynthID watermarking tools to additional gen AI tools and more forms of media including text, audio, visual and video.
We're committed to deploying AI responsibly — from using AI to strengthen our platforms against abuse to developing tools to enhance media literacy and trust — all while focused on the importance of collaborating, sharing insights and building AI responsibly, together.